Hierarchical Transfer Learning for Cycle Time Forecasting for Semiconductor Wafer Lot under Different Work in Process Levels
MATHEMATICS(2021)
摘要
The accurate cycle time (CT) prediction of the wafer fabrication remains a tough task, as the system level of work in process (WIP) is fluctuant. Aiming to construct one unified CT forecasting model under dynamic WIP levels, this paper proposes a transfer learning method for finetuning the predicted neural network hierarchically. First, a two-dimensional (2D) convolutional neural network was constructed to predict the CT under a primary WIP level with the input of spatial-temporal characteristics by reorganizing the input parameters. Then, to predict the CT under another WIP level, a hierarchical optimization transfer learning strategy was designed to finetune the prediction model so as to improve the accuracy of the CT forecasting. The experimental results demonstrated that the hierarchically transfer learning approach outperforms the compared methods in the CT forecasting with the fluctuation of WIP levels.
更多查看译文
关键词
wafer fabrication,cycle time,time series prediction,work in process,convolutional neural network,hierarchical optimization,transfer learning
AI 理解论文
溯源树
样例
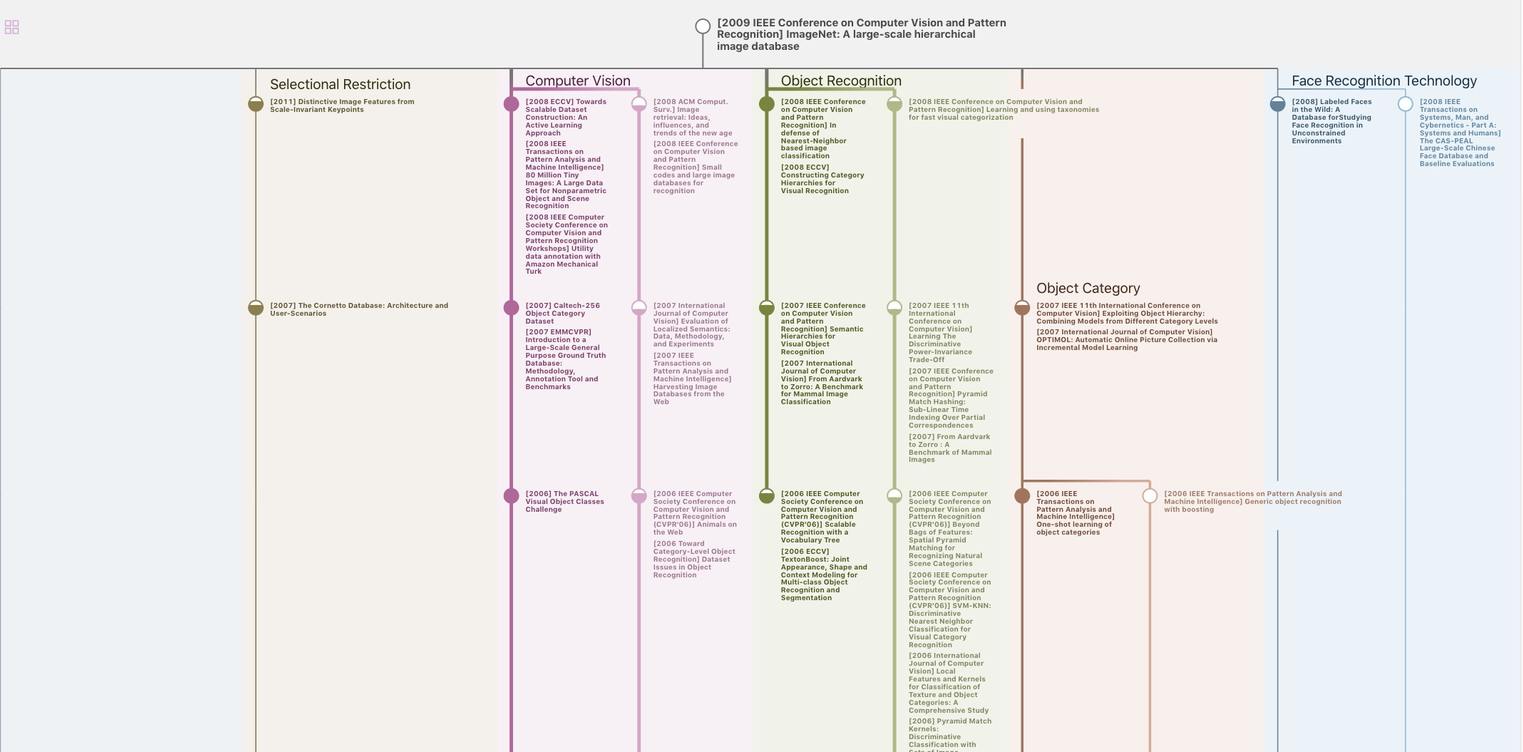
生成溯源树,研究论文发展脉络
Chat Paper
正在生成论文摘要