Predictive Models Leveraging Machine Learning And Real-World Data For Early Diagnosis: An Application In Amyotrophic Lateral Sclerosis
VALUE IN HEALTH(2021)
摘要
To assess the utility of machine learning for predicting early diagnosis of amyotrophic lateral sclerosis (ALS) based on real-world data (RWD). We identified 4779 patients with ALS and without primary lateral sclerosis from the Optum® de-identified Electronic Health Record (EHR) dataset (2007-2020), and 47,781 patients as the control cohort who did not have ALS and were demographically matched by age and gender in a 1:10 target to control ratio. Mutual information was used to explore and identify features in RWD, including lab, microbiology, and natural language processing biomarkers available in EHR, by comparing the target population (ALS patients) with the demographically matched control cohort. We trained various machine learning models (eg, logistic regression, random forest, gradient boosting, support vector machines, neural networks, soft voting) spanning different periods of time relative to a defined index date and compared their performance in predicting early diagnosis of ALS. Predictive models trained with gradient boosting on data closer to the defined index date, including lab tests from EHR, performed the best and had a very low false positive rate (AUC=0.9463). This model suggested that the top 5 predictors of an undiagnosed ALS patient were muscle weakness (generalized), normal thyroid stimulating hormone levels, dysphagia (unspecified), cramp of limb/abnormal involuntary movements, and other musculoskeletal symptoms referable to limbs. Many of the features were diagnoses that could be considered for an earlier evaluation of ALS in clinical practice. Indeed, the model had a sensitivity of 1%, specificity >99.0%, and was able to identify with a precision of 63% patients not yet identified with ALS, suggesting that early screening for ALS would be beneficial. This study highlights opportunities of leveraging machine learning utilizing EHR RWD to identify features that predict early diagnosis of ALS.
更多查看译文
关键词
amyotrophic lateral sclerosis,machine learning,models,real-world
AI 理解论文
溯源树
样例
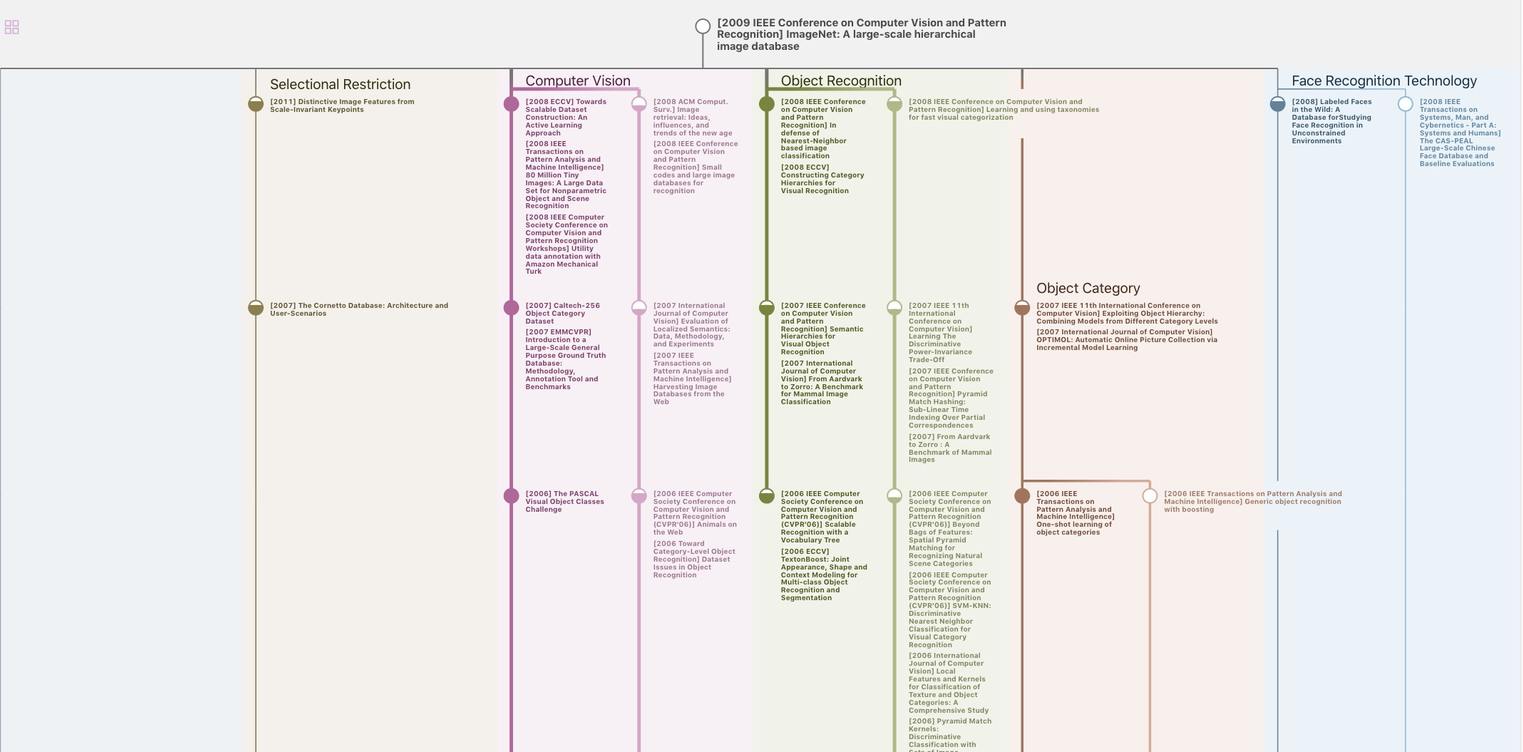
生成溯源树,研究论文发展脉络
Chat Paper
正在生成论文摘要