Learning framework for carbon emissions predictions incorporating a RReliefF driven features selection and an iterative neural network architecture improvement
SN APPLIED SCIENCES(2021)
摘要
Inaccurate carbon emissions predictions may be one of the root factors leading to the overall ineffectiveness of the European Union environmental regulatory framework. Therefore, the present article aims at introducing a novel computational learning framework for carbon emissions prediction incorporating a RReliefF driven features selection and an iterative neural network architecture improvement. Our learning framework algorithmic architecture iteratively chains the features selection process and the backpropagation artificial neural network architecture design based on the data assessment accomplished by the RReliefF algorithm. Thus a better features set / neural network architecture combination is obtained for each specific prediction target. The implemented framework was trained and tested with real world data obtained from the European Union, International Energy Agency, Organisation for Economic Co-operation and Development, and World Bank, for the period 1990–2017. The framework evaluation against current mainstream machine learning models, and its benchmarking comparing to recent published researches on carbon emissions prediction indicates that our research contribution is relevant and capable of supporting the improvement of environmental policies. Graphic abstract
更多查看译文
关键词
CO2 Emissions prediction,Backpropagation neural network,RReliefF,Features selection,Explainable AI
AI 理解论文
溯源树
样例
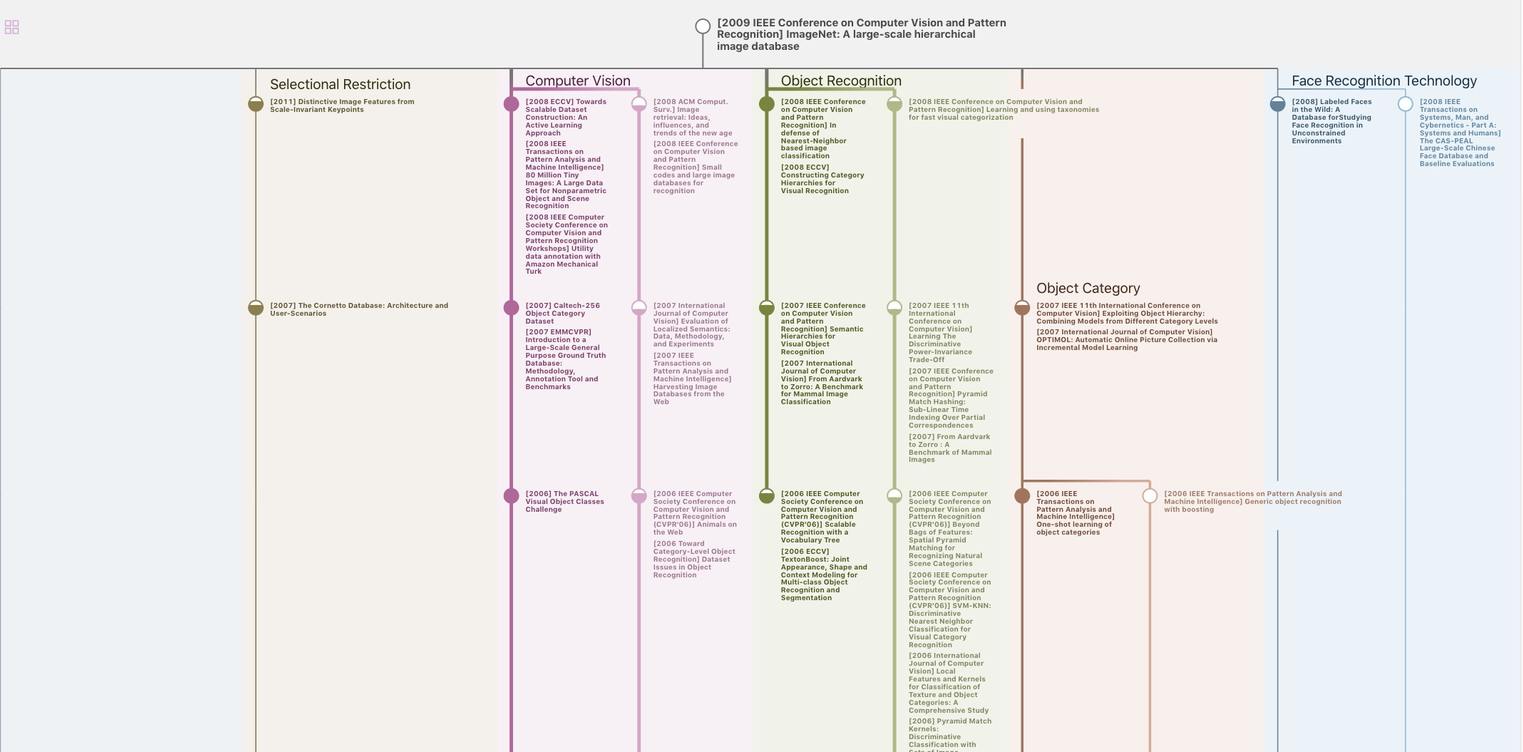
生成溯源树,研究论文发展脉络
Chat Paper
正在生成论文摘要