Random Forest And Long Short-Term Memory Based Machine Learning Models For Classification Of Ion Mobility Spectrometry Spectra
CHEMICAL, BIOLOGICAL, RADIOLOGICAL, NUCLEAR, AND EXPLOSIVES (CBRNE) SENSING XXII(2021)
摘要
The development of alarm algorithms in ion mobility spectrometry (IMS) based chemical vapor detection is challenged by the presence of overlapping chemical peaks. IMS technology identifies a chemical through hard-coded alarm windows. Alarm windows are designed as range of reduced mobility values, and act as an IF-THEN statement. Where if a peak forms in the region it then assigns a preset alarm label. A majority of IMS alarm algorithm design has relied on setting boundary conditions based on a statistical variance in product ion peak positions. To develop these alarm windows for IMS detectors the variance in peak position had to be captured through extensive laboratory testing. These windows are determined through time consuming and rigorous laboratory testing across multiple detectors under multiple conditions. Machine learning (ML) is a field of science that intersects with computer science and mathematics to "teach" a computer using large amounts of data. The development of traditional alarm algorithms IMS has left a plethora of data available to be explored by ML techniques. Presented here is a random forest (RF) classification model along with a long short-term memory (LSTM) based neural network model to label the spectra of IMS data with high accuracy.
更多查看译文
关键词
Machine Learning, Ion mobility Spectrometry, false alarm, Random Forest, LSTM
AI 理解论文
溯源树
样例
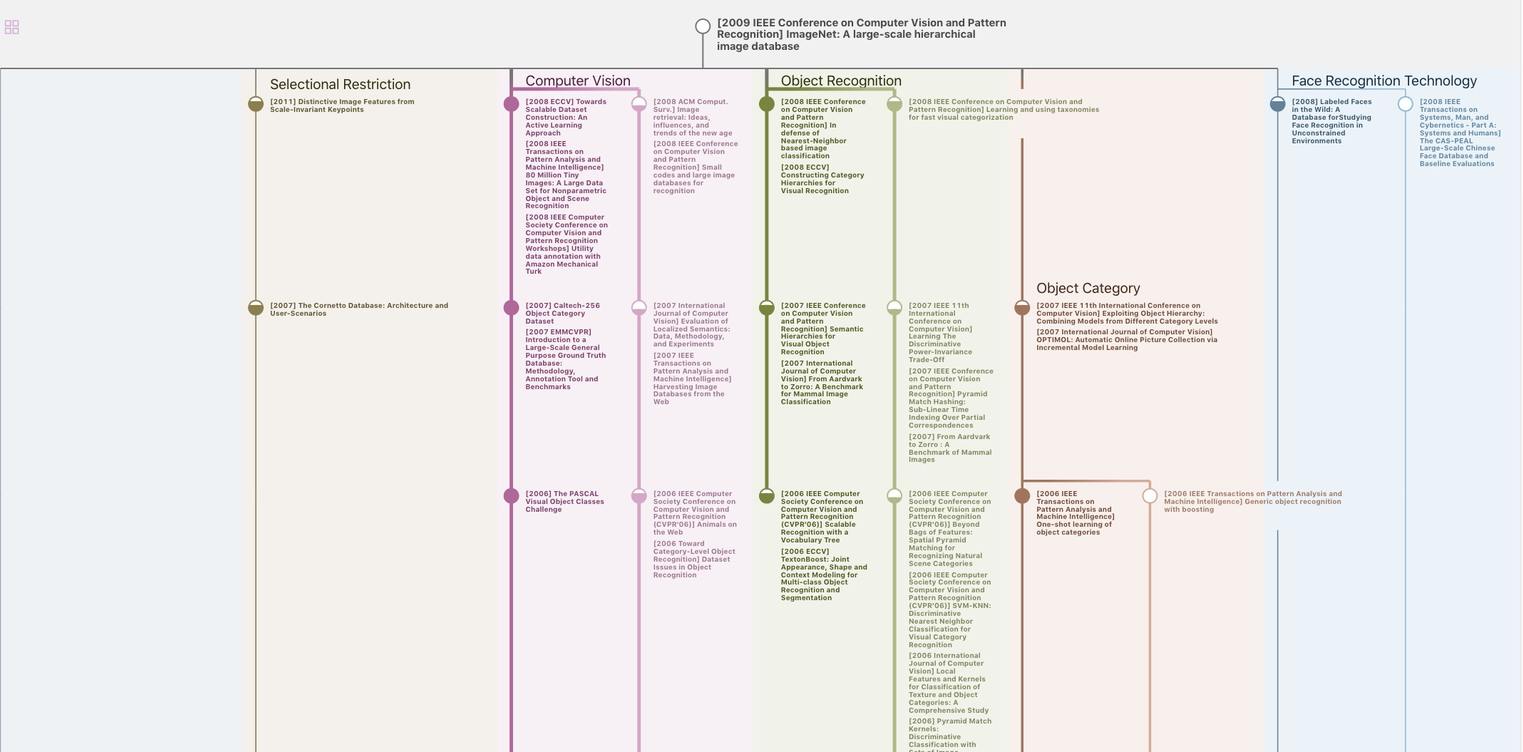
生成溯源树,研究论文发展脉络
Chat Paper
正在生成论文摘要