Integrating data-driven and simulation models to predict traffic state affected by road incidents
Transportation Letters(2022)
摘要
Predicting the traffic conditions in urban networks is a priority for traffic management centres. This becomes very challenging, especially when the network is affected by traffic incidents that vary in both time and space. Although data-driven modelling can be considered an ideal tool for short-term traffic predictions, its performance is severely degraded if little historical traffic information is available under incident conditions. This paper addresses this challenge by integrating data-driven and traffic simulation modelling approaches. Instead of directly predicting the traffic states using limited historical data, we employ a traffic simulation reinforced by data-driven models. The traffic simulation uses newly reported incident information and the estimated origin-destination (OD) demand flows to capture the complex interaction between drivers and road network, and predicts traffic states under extreme conditions. We showcase the capability of the proposed data-driven enforced traffic simulation platform for incident impact analysis in a real-life sub-network in Sydney, Australia.
更多查看译文
关键词
Demand estimation and prediction,micro-simulation,machine learning,incident management
AI 理解论文
溯源树
样例
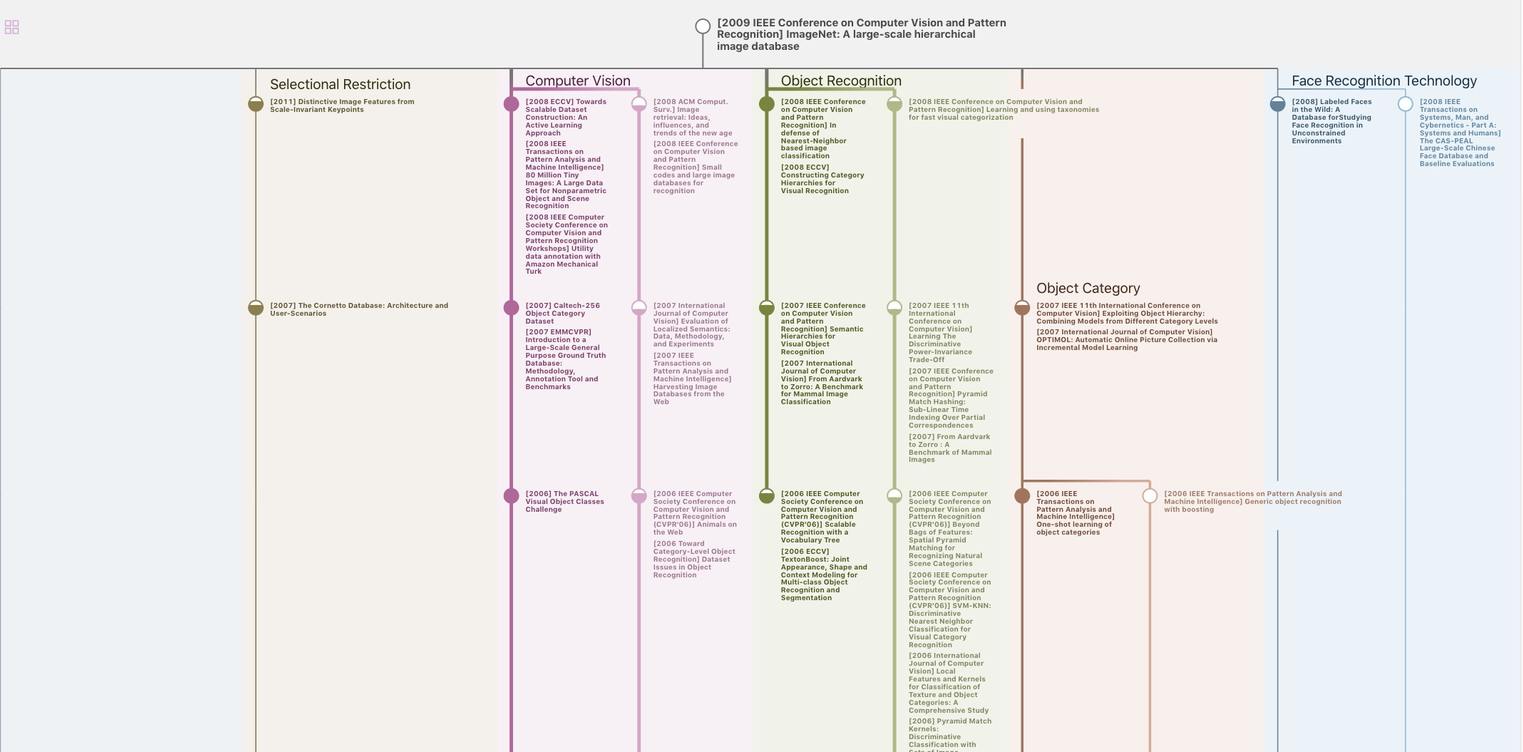
生成溯源树,研究论文发展脉络
Chat Paper
正在生成论文摘要