An Ultralow-Power Real-Time Machine Learning Based fNIRS Motion Artifacts Detection
IEEE TRANSACTIONS ON VERY LARGE SCALE INTEGRATION (VLSI) SYSTEMS(2024)
Abstract
Due to iterative matrix multiplications or gradient computations, machine learning modules often require a large amount of processing power and memory. As a result, they are often not feasible for use in wearable devices, which have limited processing power and memory. In this study, we propose an ultralow-power and real-time machine learning-based motion artifact detection module for functional near-infrared spectroscopy (fNIRS) systems. We achieved a high classification accuracy of 97.42%, low field-programmable gate array (FPGA) resource utilization of 38 354 lookup tables and 6024 flip-flops, as well as low power consumption of 0.021 W in dynamic power. These results outperform conventional CPU support vector machine (SVM) methods and other state-of-the-art SVM implementations. This study has demonstrated that an FPGA-based fNIRS motion artifact classifier can be exploited while meeting low power and resource constraints, which are crucial in embedded hardware systems while keeping high classification accuracy.
MoreTranslated text
Key words
Support vector machines,Functional near-infrared spectroscopy,Motion artifacts,Field programmable gate arrays,Hardware,Machine learning,Kernel,Field-programmable gate array (FPGA),functional near-infrared spectroscopy (fNIRS),low power,machine learning,motion artifact detection,real time,support vector machines (SVMs)
AI Read Science
Must-Reading Tree
Example
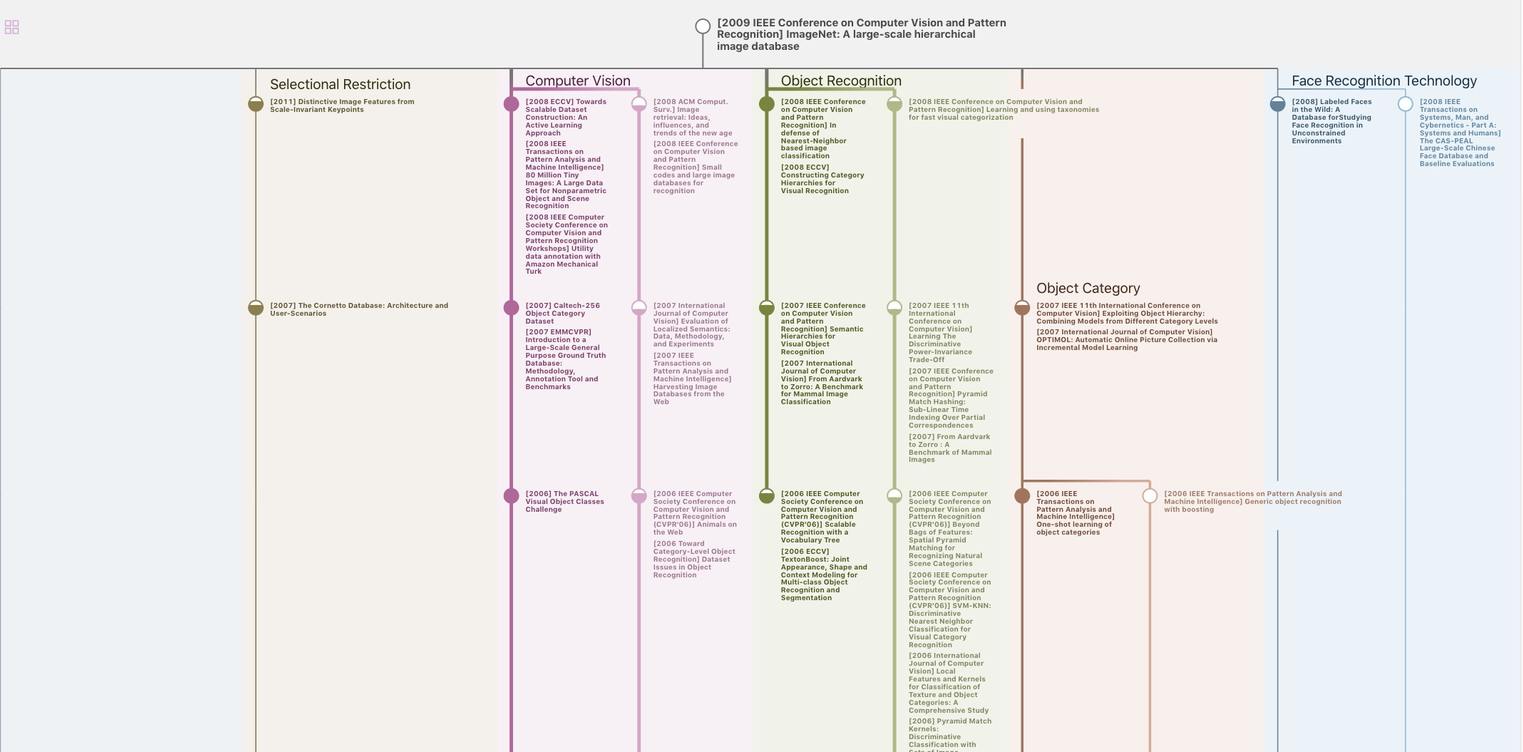
Generate MRT to find the research sequence of this paper
Chat Paper
Summary is being generated by the instructions you defined