Interpretable video tag recommendation with multimedia deep learning framework
INTERNET RESEARCH(2022)
摘要
Purpose Tags help promote customer engagement on video-sharing platforms. Video tag recommender systems are artificial intelligence-enabled frameworks that strive for recommending precise tags for videos. Extant video tag recommender systems are uninterpretable, which leads to distrust of the recommendation outcome, hesitation in tag adoption and difficulty in the system debugging process. This study aims at constructing an interpretable and novel video tag recommender system to assist video-sharing platform users in tagging their newly uploaded videos. Design/methodology/approach The proposed interpretable video tag recommender system is a multimedia deep learning framework composed of convolutional neural networks (CNNs), which receives texts and images as inputs. The interpretability of the proposed system is realized through layer-wise relevance propagation. Findings The case study and user study demonstrate that the proposed interpretable multimedia CNN model could effectively explain its recommended tag to users by highlighting keywords and key patches that contribute the most to the recommended tag. Moreover, the proposed model achieves an improved recommendation performance by outperforming state-of-the-art models. Practical implications The interpretability of the proposed recommender system makes its decision process more transparent, builds users' trust in the recommender systems and prompts users to adopt the recommended tags. Through labeling videos with human-understandable and accurate tags, the exposure of videos to their target audiences would increase, which enhances information technology (IT) adoption, customer engagement, value co-creation and precision marketing on the video-sharing platform. Originality/value The proposed model is not only the first explainable video tag recommender system but also the first explainable multimedia tag recommender system to the best of our knowledge.
更多查看译文
关键词
Interpretable AI, Machine learning, Recommender system, User-generated content, Multimedia, Convolutional neural network
AI 理解论文
溯源树
样例
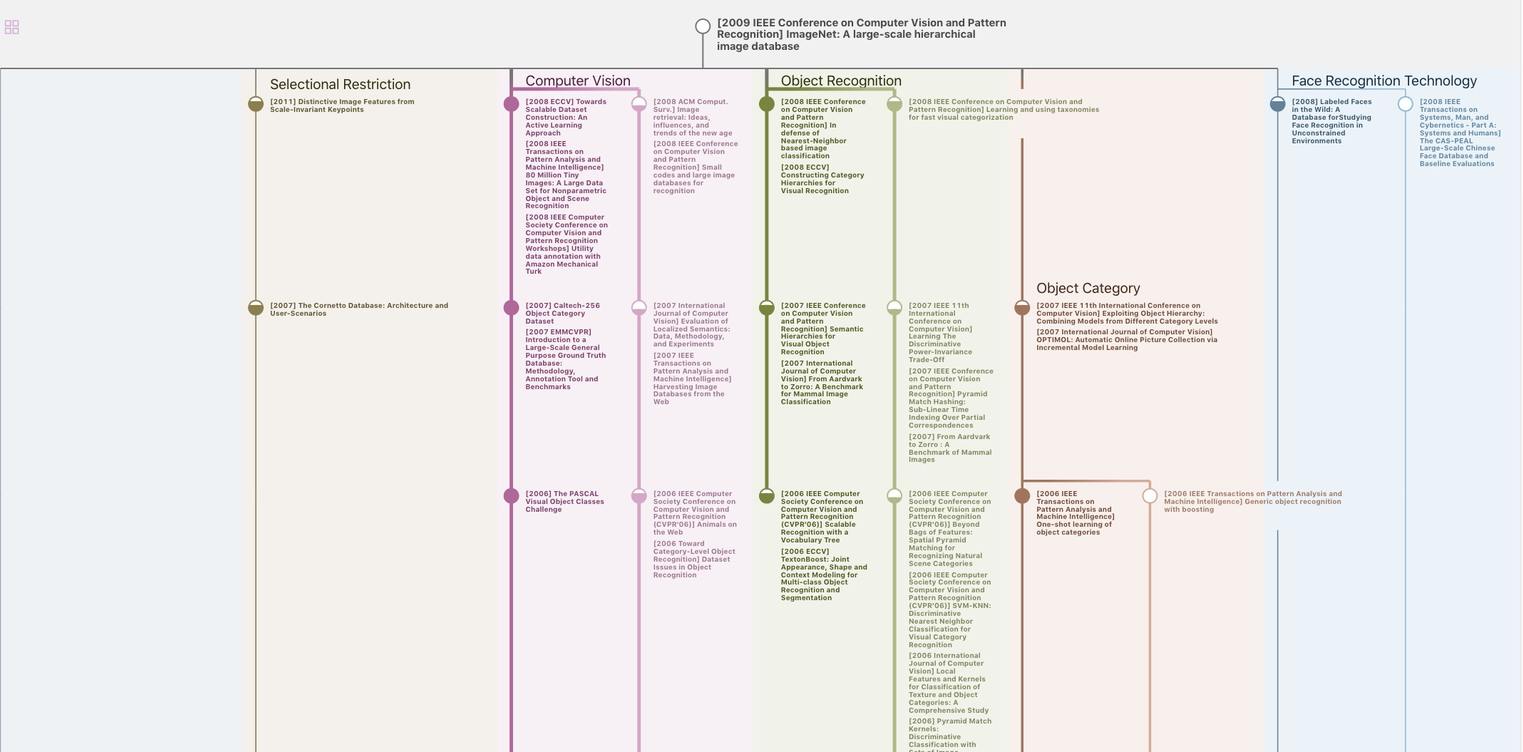
生成溯源树,研究论文发展脉络
Chat Paper
正在生成论文摘要