FL-MAC-RDP: Federated Learning over Multiple Access Channels with Rényi Differential Privacy
INTERNATIONAL JOURNAL OF THEORETICAL PHYSICS(2021)
Abstract
Federated Learning (FL) is a promising paradigm, where the local users collaboratively learn models by repeatedly sharing information while the data is kept distributing on these users. FL has been considered in multiple access channels (FL-MAC), which is a hot issue. Even though FL-MAC has many advantages, it is still possible to leak privacy to a third party during the whole training process. To avoid privacy leakage, we propose to add Rényi differential privacy (RDP) into FL-MAC. At the same time, to maximize the convergent rate of users under the constraints of transmission rate and privacy, the quantization stochastic gradient descent (QSGD) is performed by users. We also illustrate our results on MNIST, and the illustration demonstrate that our scheme can improve the model accuracy with a little loss of communication efficiency.
MoreTranslated text
Key words
Rényi differential privacy,Federated learning,Multiple access channels,Quantization Stochastic Gradient Descent
AI Read Science
Must-Reading Tree
Example
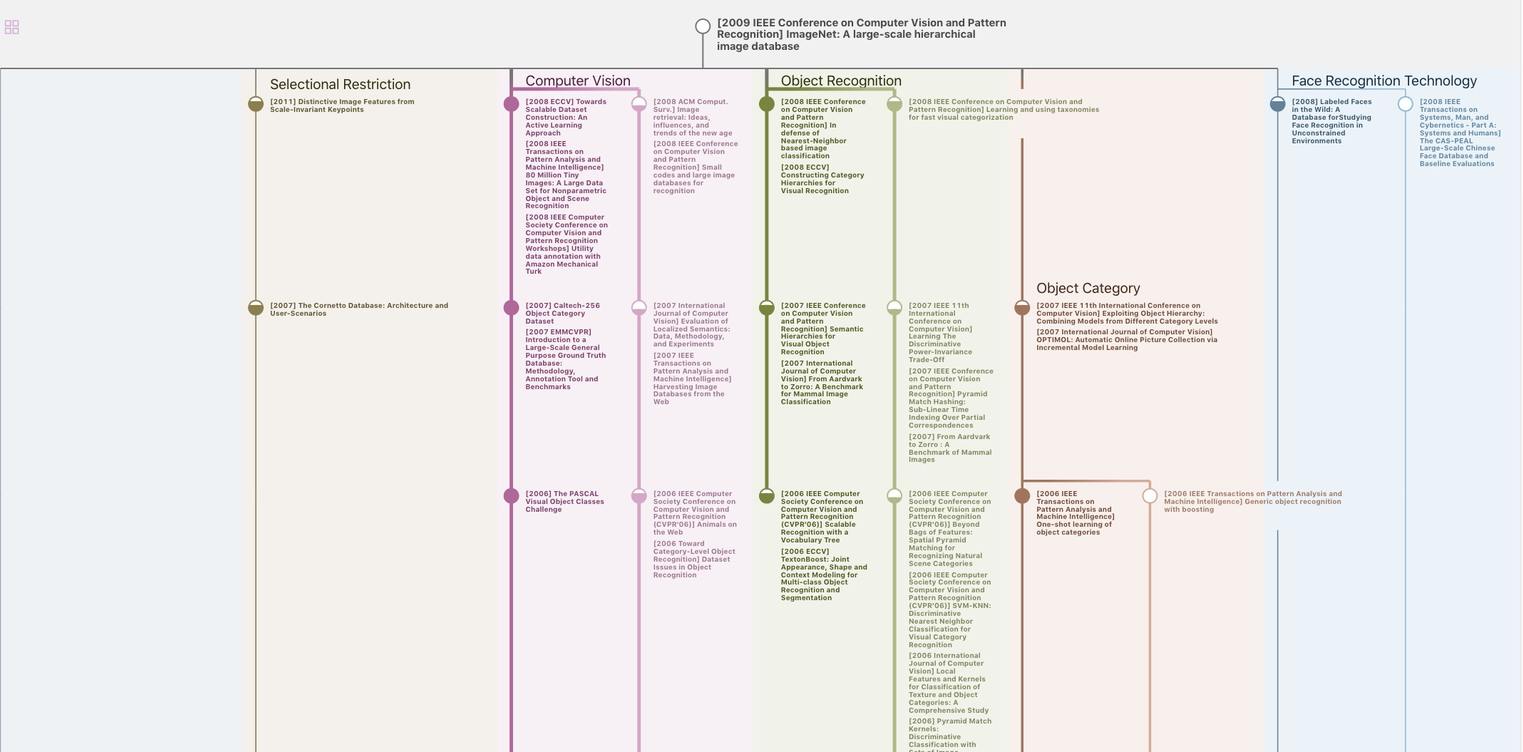
Generate MRT to find the research sequence of this paper
Chat Paper
Summary is being generated by the instructions you defined