Data-driven prediction of unsteady pressure distributions based on deep learning
Journal of Fluids and Structures(2021)
摘要
In the present work, an efficient Reduced-Order Model is developed for the prediction of motion-induced unsteady pressure distributions. The model is trained on the basis of synthetic data generated by full-order Computational Fluid Dynamics (CFD) simulations. The nonlinear identification task is to predict a snapshot representing the pressure distribution for the current time step based on respective snapshots of previous time steps and applied excitation. Once a Reduced-Order Model is conditioned on training data, it can predict sequences of the pressure distribution in a recurrent manner based on the excitation signal. Hence, it is able to capture the motion-induced nonlinear unsteady aerodynamics for a given configuration at fixed free-stream conditions. In this way, computationally extensive CFD simulations can be substituted by the application of the more efficient Reduced-Order Model. The nonlinear behavior of the aerodynamic system is captured based on a deep convolutional neural network. The performance of the Reduced-Order Model is demonstrated based on the LANN (Lockheed-Georgia, Air Force Flight Dynamics Laboratory, NASA-Langley and NLR) wing performing high-amplitude pitching motion in transonic flow. The unsteady aerodynamics of the considered test case is dominated by nonlinear effects due to complex moving shock structures both on the upper and lower surface of the wing. The Reduced-Order Model yields a superior prediction accuracy at a speed-up of more than three orders of magnitude compared to the employed CFD method. (C) 2021 Elsevier Ltd. All rights reserved.
更多查看译文
关键词
Deep learning,Unsteady aerodynamics,Reduced-Order Models,Computational Fluid Dynamics,LANN model,Transonic flight
AI 理解论文
溯源树
样例
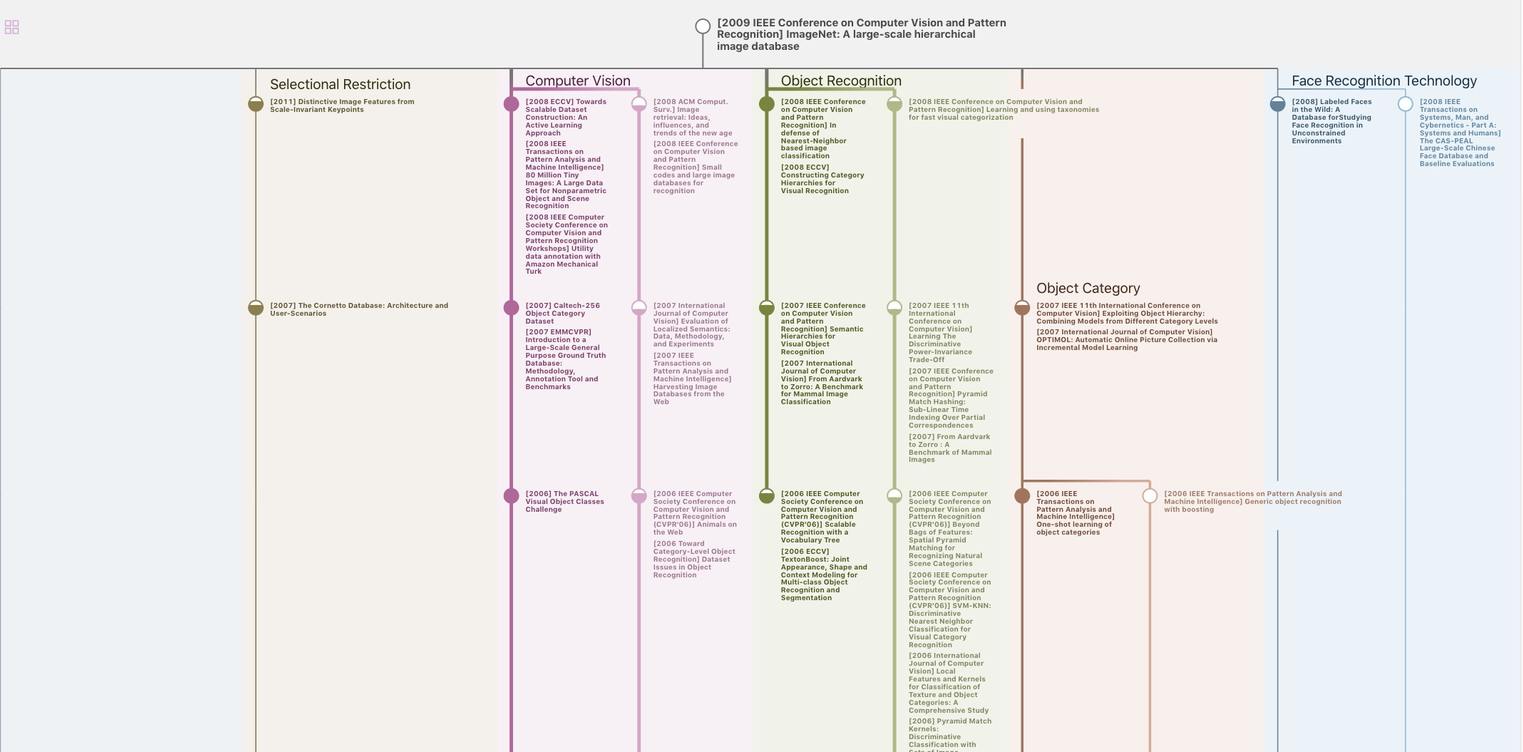
生成溯源树,研究论文发展脉络
Chat Paper
正在生成论文摘要