Identification Of Efficacious Treatment For Glioblastoma (Gbm) By Applying Computational Drug Sensitivity Imputation To Novel Gbm Avatars And Gbm Clinical Datasets.
CANCER RESEARCH(2021)
摘要
Abstract Glioblastoma (GBM) is a debilitating disease associated with poor prognosis and a limited response to therapies. At present, patients receive chemotherapy with temozolomide, following radiation therapy and surgery; however, none of the treatments are curative, and clinical trials are recommended as the preferred option for eligible patients. Regrettably, only 3-5% of GBM patients survive 3 years or more. Therefore, it is imperative to develop efficacious therapies. To achieve this goal, we have developed a computation method that allows us to impute drug sensitivity when transcriptome data is available. We have applied this imputation method to several publicly available normal brain tissue, low grade glioma (LGG), and GBM patient datasets to identify compounds of interest. We also compared the leads from these patient/in vivo data analyses with those generated from previous imputations made using a novel GBM avatar model; this model was created by introducing different genetic driver mutations using CRISPR-Cas9 into human induced pluripotent stem cells, followed by differentiation to GBM-associated mutations containing neural progenitor cells (NPC) and animal engraftment to develop human adult GBM models. We applied the drug imputation to over 1200 human normal brain, LGG and GBM samples as well as 18 NPC, tumor sphere and engrafted GBM tumor avatar samples. For each sample, we imputed over 700 drug sensitivity scores. Our model was trained independently using one of two separate high throughput in vitro drug screening datasets (GDSC and CTRP). To identify drugs showing higher sensitivity in GBM than in normal tissues, we compared the imputed drug sensitivity for each compound between samples belonging to the precancerous or the non-high grade glioma state (represented by the normal tissue and LGG data) and samples representing the cancerous or high grade glioma state (GBM). We identified a number of compounds showing higher imputed sensitivity in samples belonging to the cancerous state when compared to those in the precancerous or LGG state. We were also able to cross check compounds of interest with our avatar data. Among them, some agents have been used in the clinic to treat GBM, supporting the validity of integration of our computational tool with novel ex vivo GBM avatars and GBM clinical data in the development of new therapeutic strategy for GBM. More importantly, we have identified other compounds with various mechanism of action that show higher efficacy in GBM and will be further evaluated with the goal to quickly translate into clinic. Therefore, we can successfully integrate ovel experimental and computational models to speed up drug discovery with the goal of finding new therapeutics for GBM. Citation Format: Danielle Maeser, Robert Gruener, Robert Galvin, Tomoyuki Koga, Frank B. Furnari, David Largaespada, Clark C. Chen, Stephanie R. Huang. Identification of efficacious treatment for glioblastoma (GBM) by applying computational drug sensitivity imputation to novel GBM avatars and GBM clinical datasets [abstract]. In: Proceedings of the American Association for Cancer Research Annual Meeting 2021; 2021 Apr 10-15 and May 17-21. Philadelphia (PA): AACR; Cancer Res 2021;81(13_Suppl):Abstract nr 1300.
更多查看译文
AI 理解论文
溯源树
样例
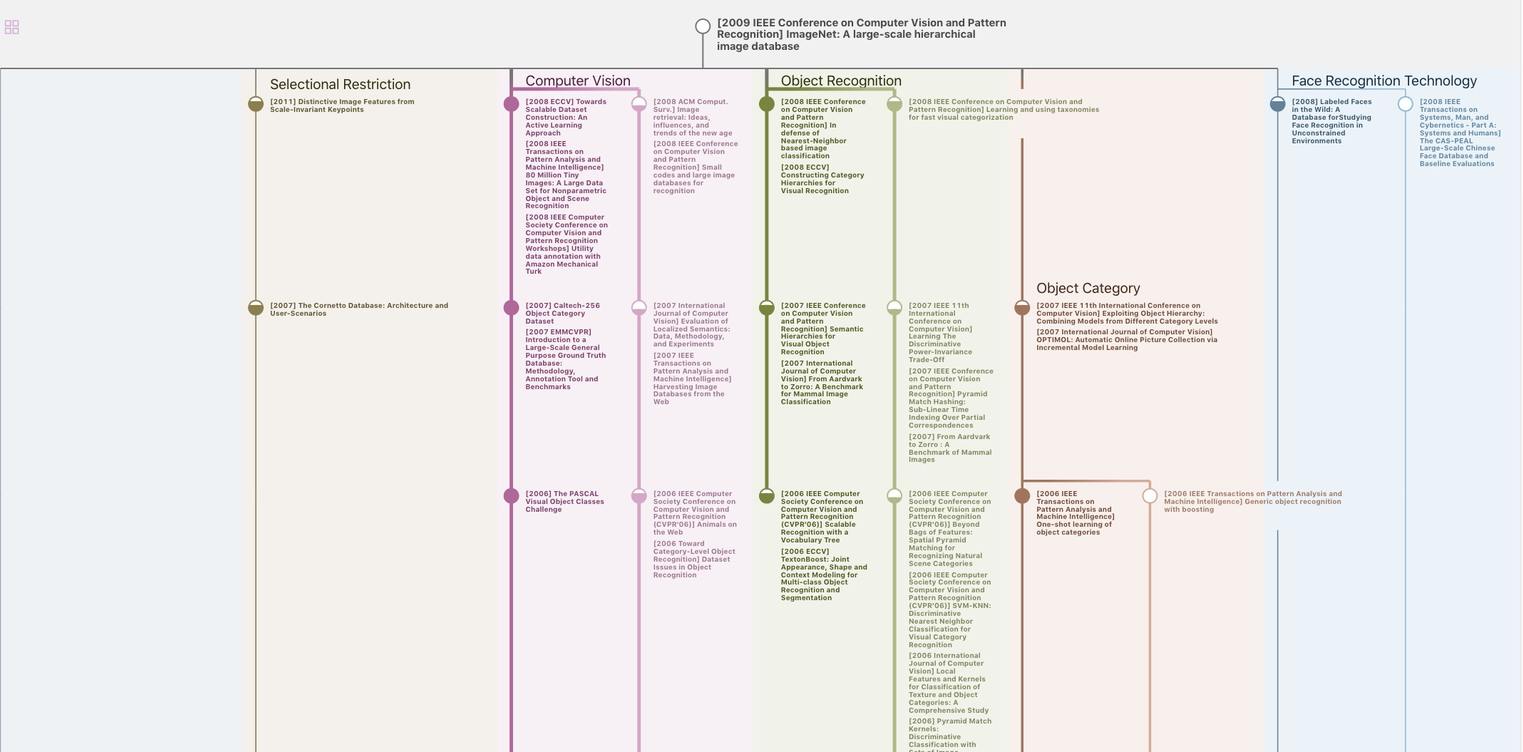
生成溯源树,研究论文发展脉络
Chat Paper
正在生成论文摘要