An Improved Two-Stream Inflated 3d Convnet For Abnormal Behavior Detection
INTELLIGENT AUTOMATION AND SOFT COMPUTING(2021)
摘要
Abnormal behavior detection is an essential step in a wide range of application domains, such as smart video surveillance. In this study, we proposed an improved two-stream inflated 3D ConvNet network approach based on probability regression for abnormal behavior detection. The proposed approach consists of four parts: (1) preprocessing pretreatment for the input video; (2) dynamic feature extraction from video streams using a two-stream inflated 3D (I3D) ConvNet network; (3) visual feature transfer into a two-dimensional matrix; and (4) feature classification using a generalized regression neural network (GRNN), which ultimately achieves a probability regression. Compared with the traditional methods, two-stream I3D feature extraction technology is better able to extract visual features and retain the optical flow and red, green and blue (RGB) information in the video. Probabilistic regression technology is better able to quantify data and provide a more intuitive visual experience. The experimental results on 50 detection cases from the UCF-Crime dataset show that the developed model obtains a high average abnormal behavior recognition accuracy. The improved I3D models obtain an average accuracy of 93.7% based on UCF-101, which outperforms the state-of-the-art methods, verifying the robustness and effectiveness of our approach.
更多查看译文
关键词
Abnormal behavior detection, two-stream inflated 3D convnet network, general regression neural network, python
AI 理解论文
溯源树
样例
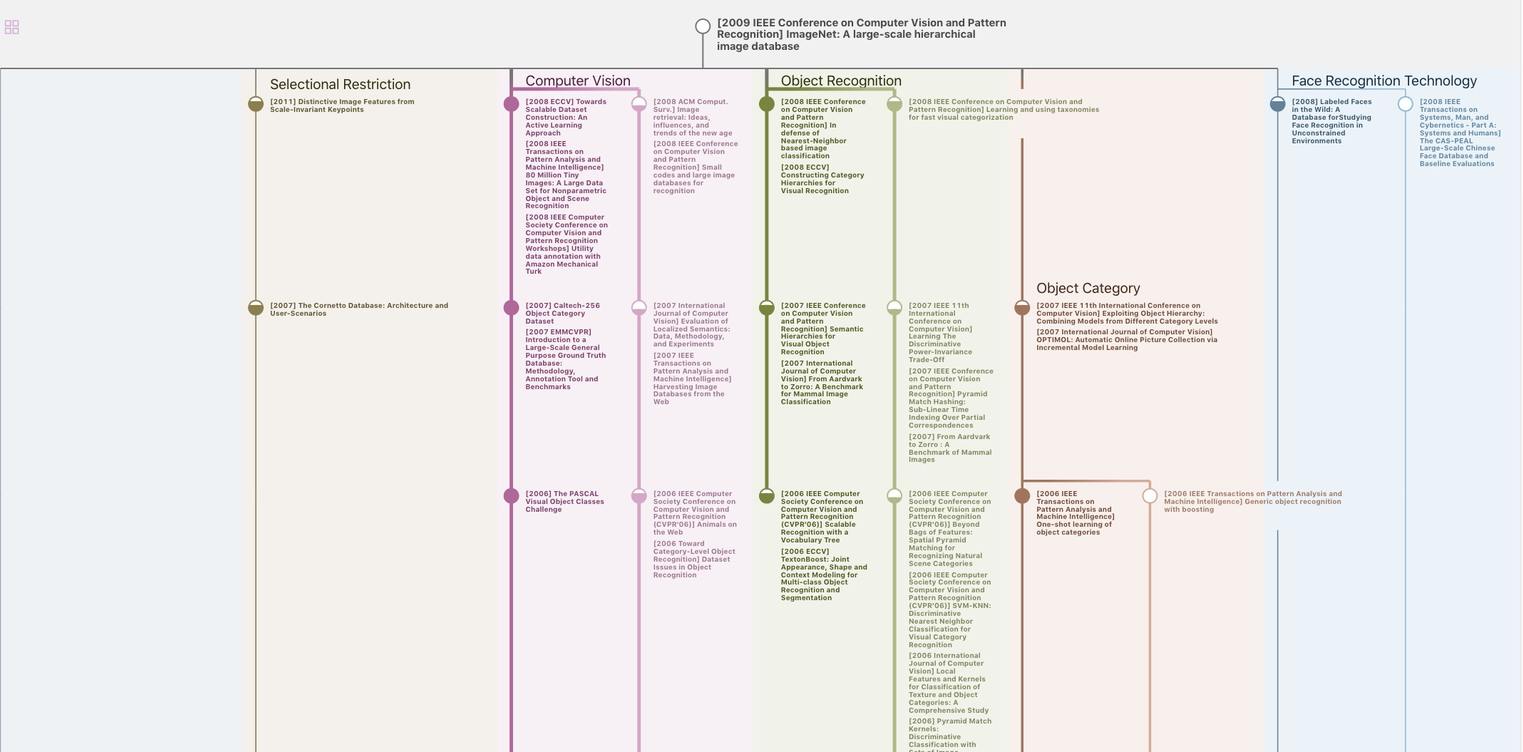
生成溯源树,研究论文发展脉络
Chat Paper
正在生成论文摘要