Cross-Operating Condition Degradation Knowledge Learning For Remaining Useful Life Estimation Of Bearings
IEEE TRANSACTIONS ON INSTRUMENTATION AND MEASUREMENT(2021)
摘要
As one of the most crucial issues in the condition monitoring of mechanical systems, the remaining useful life (RUL) estimation of bearings suffers from the limitation of run-to-failure data, which makes the cross-operating condition RUL estimation of bearings a challenging subject. To address this issue, a cross-operating condition degradation knowledge learning method is proposed by constructing a shared latent feature space across different operating conditions, in which the bearing degradation features are effectively extracted and the cross-domain feature differences are weakened. Focusing on learning the cross-operating condition degradation knowledge, the time-frequency representation (TFR) samples generated by the continuous wavelet transform (CWT) method in both the source and target domains were projected onto a shared latent feature space constructed by a novel joint dictionary matrix factorization (JDMF) method. The joint-domain dictionaries solved by the JDMF method have played a core role in extracting the domain-invariant features that do not change along with the operating conditions of bearings. As a result, the convolutional neural network (CNN) model trained with the source samples in the shared latent feature space can be directly generalized to the target bearings for the degradation indicator (DI) modeling and RUL estimation. The cross-operating condition bearing RUL estimation experiment executed in the PRONOSTIA platform has demonstrated the effectiveness and superiority of the proposed JDMF-CNN framework.
更多查看译文
关键词
Bearing, convolutional neural network (CNN), matrix factorization, remaining useful life (RUL), time-frequency representation (TFR), transfer learning (TL)
AI 理解论文
溯源树
样例
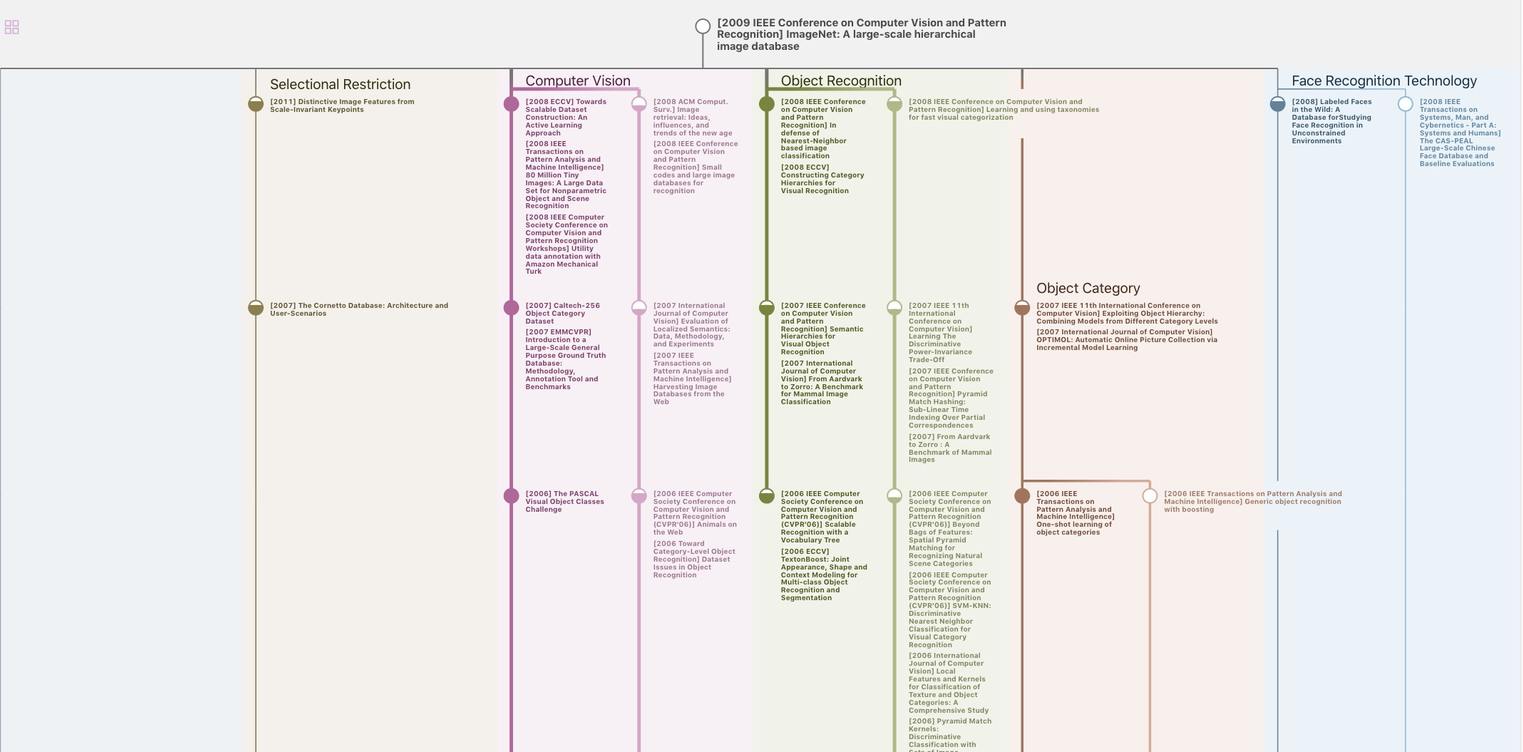
生成溯源树,研究论文发展脉络
Chat Paper
正在生成论文摘要