Hs-Kdnet: A Lightweight Network Based On Hierarchical-Split Block And Knowledge Distillation For Fault Diagnosis With Extremely Imbalanced Data
IEEE TRANSACTIONS ON INSTRUMENTATION AND MEASUREMENT(2021)
摘要
Because of the cost, it is unrealistic to sample the failure state for a long time, which makes the data collected from the scenario of engineering usually extremely imbalanced. However, imbalanced training data pose a negative effect on the fault diagnosis algorithms based on the data driven. When the data are extremely imbalanced, this problem becomes more challenging. Furthermore, to reduce the deployment cost, in industrial practice, it is often required that the parameters and computation of the deployed diagnosis model should be within a certain range, which puts forward the requirement of lightweight for diagnosis model. Therefore, in this article, a novel lightweight framework for fault diagnosis with extremely imbalanced data, called HS-KDNet, is proposed. Soft labels generated by knowledge distillation can represent the similarity between categories, i.e., through to learn the soft labels, the information about all categories are considered in each update of the parameters, not only the information about the current samples. Consequently, unlike traditional data re-balancing strategies based on generating pseudo samples, we utilized knowledge distillation to suppress the adverse effects of imbalanced data for the first time. On two classical bearing datasets, the effectiveness and superiority of the proposed HS-KDNet were demonstrated, and the experimental results shown that, except for HS-KDNet, knowledge distillation can significantly inhibit the adverse effects of imbalanced data on other simple models.
更多查看译文
关键词
Convolutional neural networks (CNNs), fault diagnosis, imbalanced data, knowledge distillation, lightweight network
AI 理解论文
溯源树
样例
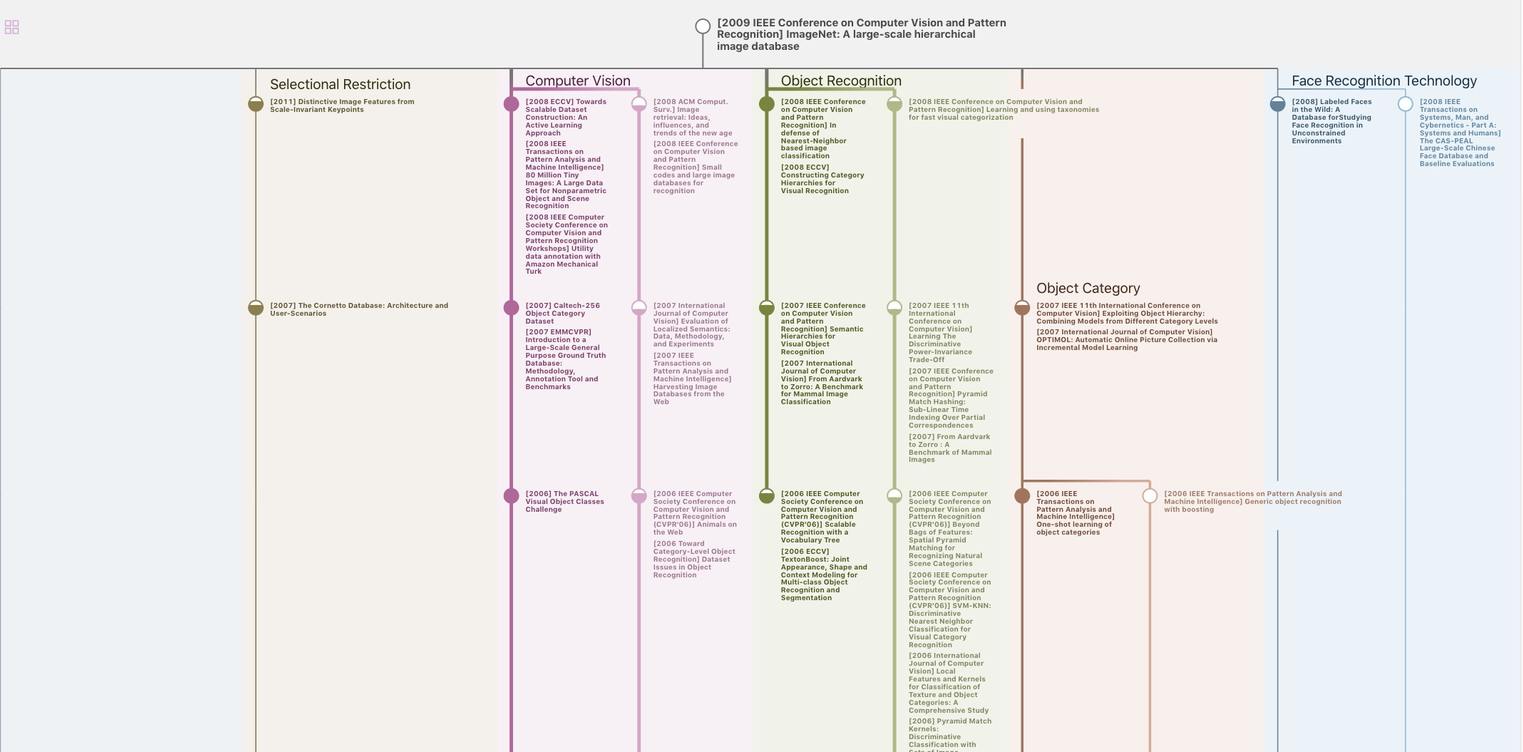
生成溯源树,研究论文发展脉络
Chat Paper
正在生成论文摘要