Detecting Pipeline Pathways in Landsat 5 Satellite Images with Deep Learning
ENERGIES(2021)
摘要
Energy system modeling is essential in analyzing present and future system configurations motivated by the energy transition. Energy models need various input data sets at different scales, including detailed information about energy generation and transport infrastructure. However, accessing such data sets is not straightforward and often restricted, especially for energy infrastructure data. We present a detection model for the automatic recognition of pipeline pathways using a Convolutional Neural Network (CNN) to address this lack of energy infrastructure data sets. The model was trained with historical low-resolution satellite images of the construction phase of British gas transport pipelines, made with the Landsat 5 Thematic Mapper instrument. The satellite images have been automatically labeled with the help of high-resolution pipeline route data provided by the respective Transmission System Operator (TSO). We have used data augmentation on the training data and trained our model with four different initial learning rates. The models trained with the different learning rates have been validated with 5-fold cross-validation using the Intersection over Union (IoU) metric. We show that our model can reliably identify pipeline pathways despite the comparably low resolution of the used satellite images. Further, we have successfully tested the model's capability in other geographic regions by deploying satellite images of the NEL pipeline in Northern Germany.
更多查看译文
关键词
pipeline detection,CNN,Landsat 5,U-Net,gas transport network
AI 理解论文
溯源树
样例
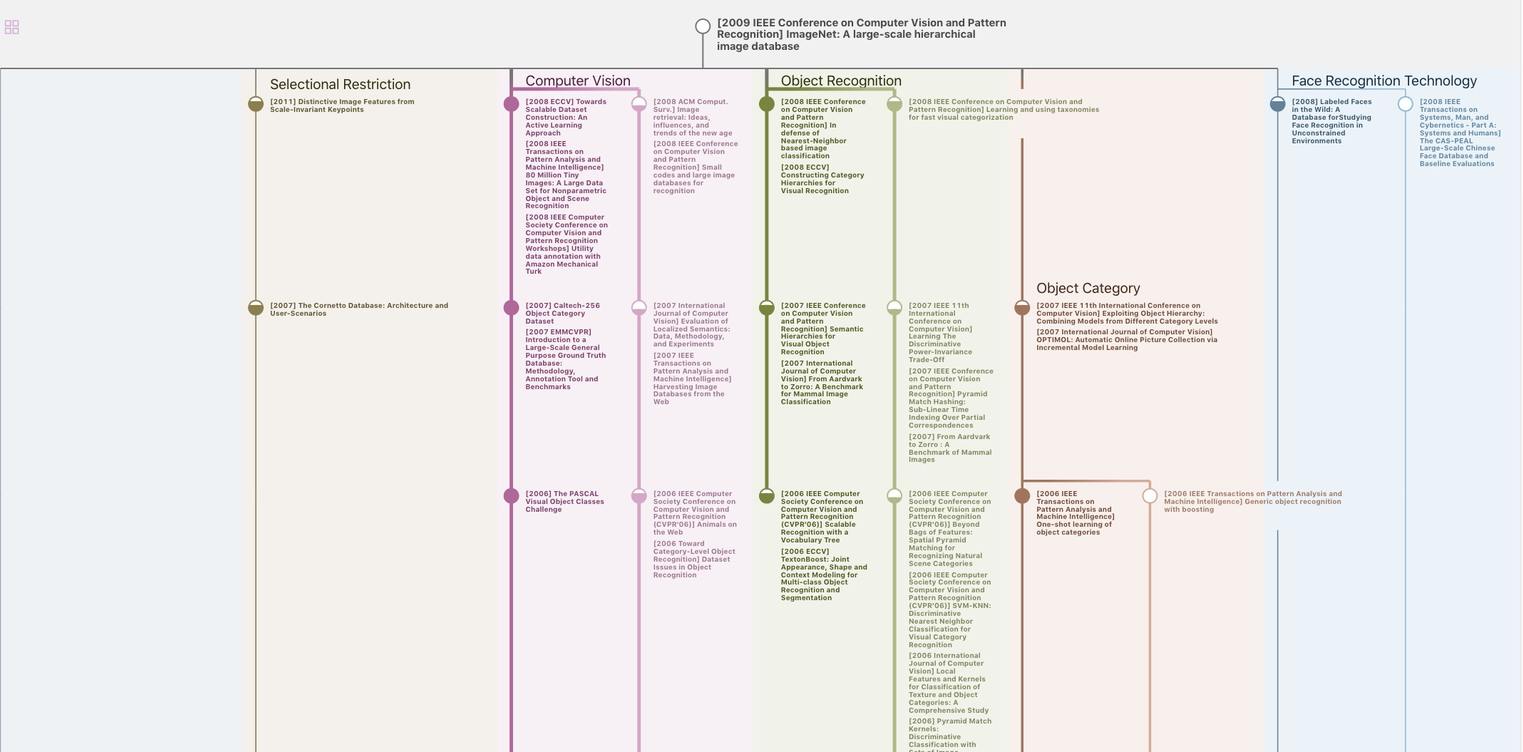
生成溯源树,研究论文发展脉络
Chat Paper
正在生成论文摘要