Probabilistic Electricity Price Forecasting With Narx Networks: Combine Point Or Probabilistic Forecasts? (Vol 36, Pg 466, 2020)
INTERNATIONAL JOURNAL OF FORECASTING(2021)
Abstract
A recent electricity price forecasting (EPF) study has shown that the Seasonal Component Artificial Neural Network (SCANN) modeling framework, which consists of decomposing a series of spot prices into a trend-seasonal and a stochastic component, modeling them independently and then combining their forecasts, can yield more accurate point predictions than an approach in which the same non-linear autoregressive NARX-type neural network is calibrated to the prices themselves. Here, considering two novel extensions of the SCANN concept to probabilistic forecasting, we find that (i) efficiently calibrated NARX networks can outperform their autoregressive counterparts, even without combining forecasts from many runs, and that (ii) in terms of accuracy it is better to construct probabilistic forecasts directly from point predictions, however, if speed is a critical issue, running quantile regression on combined point forecasts (i.e., committee machines) may be an option worth considering. Moreover, we confirm an earlier observation that averaging probabilities outperforms averaging quantiles when combining predictive distributions in EPF.
MoreTranslated text
Key words
probabilistic electricity price forecasting,probabilistic forecasts,narx networks
AI Read Science
Must-Reading Tree
Example
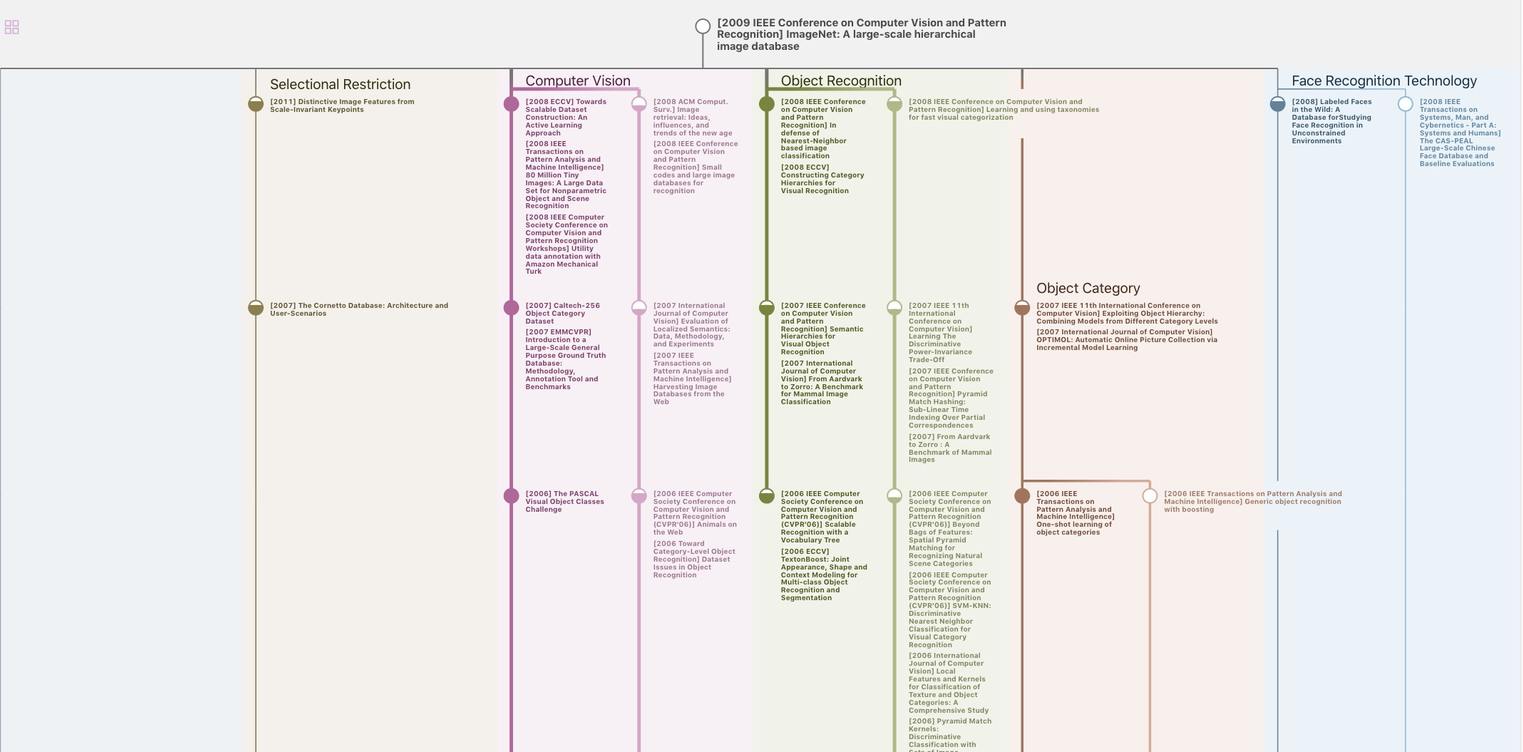
Generate MRT to find the research sequence of this paper
Chat Paper
Summary is being generated by the instructions you defined