Active Sense: Early Staging Of Non-Insulin Dependent Diabetes Mellitus (Niddm) Hinges Upon Recognizing Daily Activity Pattern
ELECTRONICS(2021)
摘要
The Human Activity Recognition (HAR) system allows various accessible entries for the early diagnosis of Diabetes as one of the nescient applications domains for the HAR. Long Short-Term Memory (LSTM) was applied and recognized 13 activities that resemble diabetes symptoms. Afterward, risk factor assessment for an experimental subject identified similar activity pattern attributes between diabetic patients and the experimental subject. Because of this, a trained LSTM model was deployed to monitor the average time length for every activity performed by the experimental subject for 30 consecutive days. Concurrently, the symptomatic diabetes activity patterns of diabetic patients were explored. The cosine similarity of activity patterns of the experimental subject and diabetic patients measured 57.39%, putting the experimental subject into moderate risk factor class. The experimental subject was clinically tested for risk factors using the diabetic clinical diagnosis process, known as the A1C. The A1C level was 6.1%, recognizing the experimental subject as a patient suffering from Diabetes. Thus, the proposed novel approach remarkably classifies the risk factor level based on activity patterns.
更多查看译文
关键词
diabetes, early staging, human activity recognition, long short-term memory, smartphone sensors
AI 理解论文
溯源树
样例
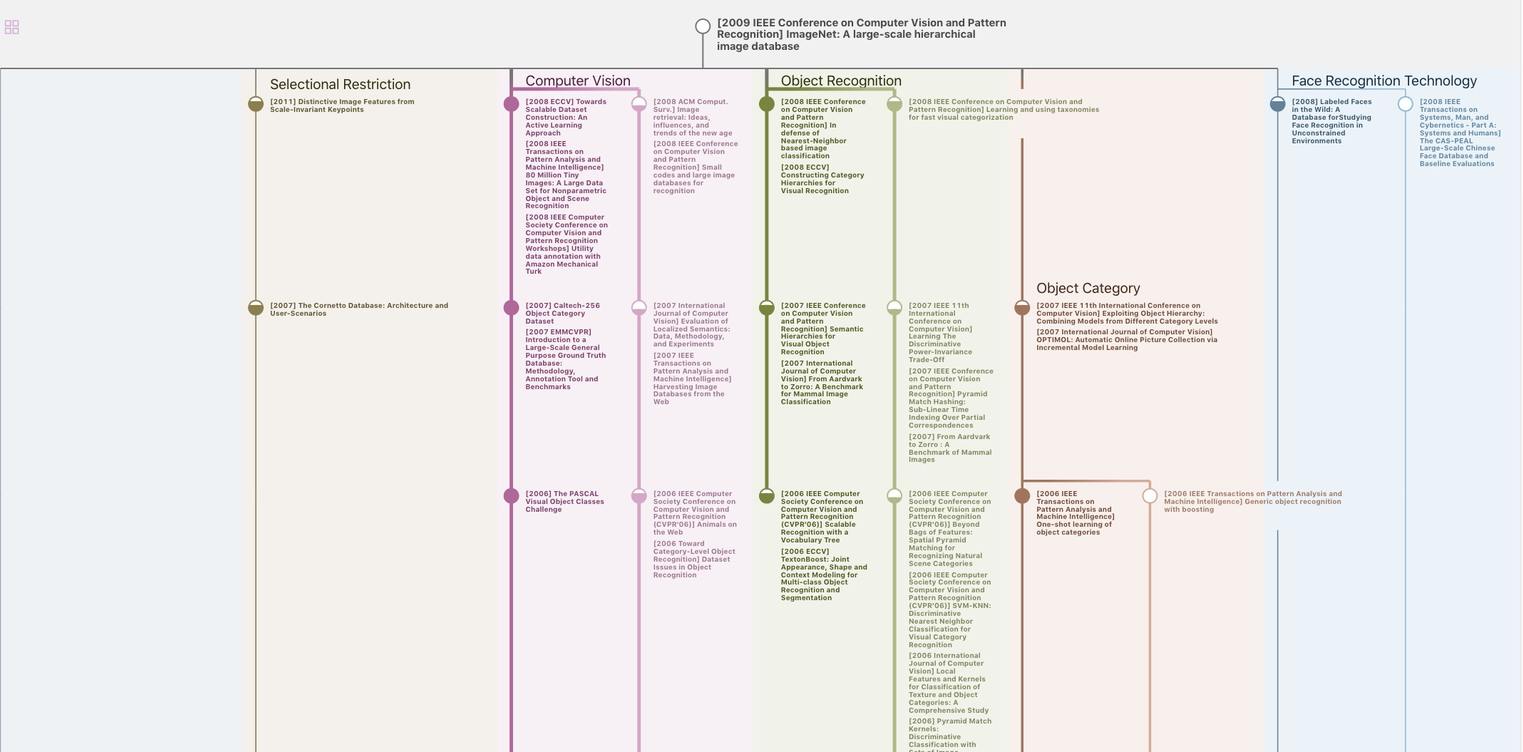
生成溯源树,研究论文发展脉络
Chat Paper
正在生成论文摘要