Classification of Contaminated Insulators Using k-Nearest Neighbors Based on Computer Vision
COMPUTERS(2021)
摘要
Contamination on insulators may increase the surface conductivity of the insulator, and as a consequence, electrical discharges occur more frequently, which can lead to interruptions in a power supply. To maintain reliability in an electrical distribution power system, components that have lost their insulating properties must be replaced. Identifying the components that need maintenance is a difficult task as there are several levels of contamination that are hard to notice during inspections. To improve the quality of inspections, this paper proposes using k-nearest neighbors (k-NN) to classify the levels of insulator contamination based on images of insulators at various levels of contamination simulated in the laboratory. Computer vision features such as mean, variance, asymmetry, kurtosis, energy, and entropy are used for training the k-NN. To assess the robustness of the proposed approach, a statistical analysis and a comparative assessment with well-consolidated algorithms such as decision tree, ensemble subspace, and support vector machine models are presented. The k-NN showed up to 85.17% accuracy using the k-fold cross-validation method, with an average accuracy higher than 82% for the multi-classification of contamination of insulators, being superior to the compared models.
更多查看译文
关键词
classification of insulators,electrical power system,k-nearest neighbors,computer vision
AI 理解论文
溯源树
样例
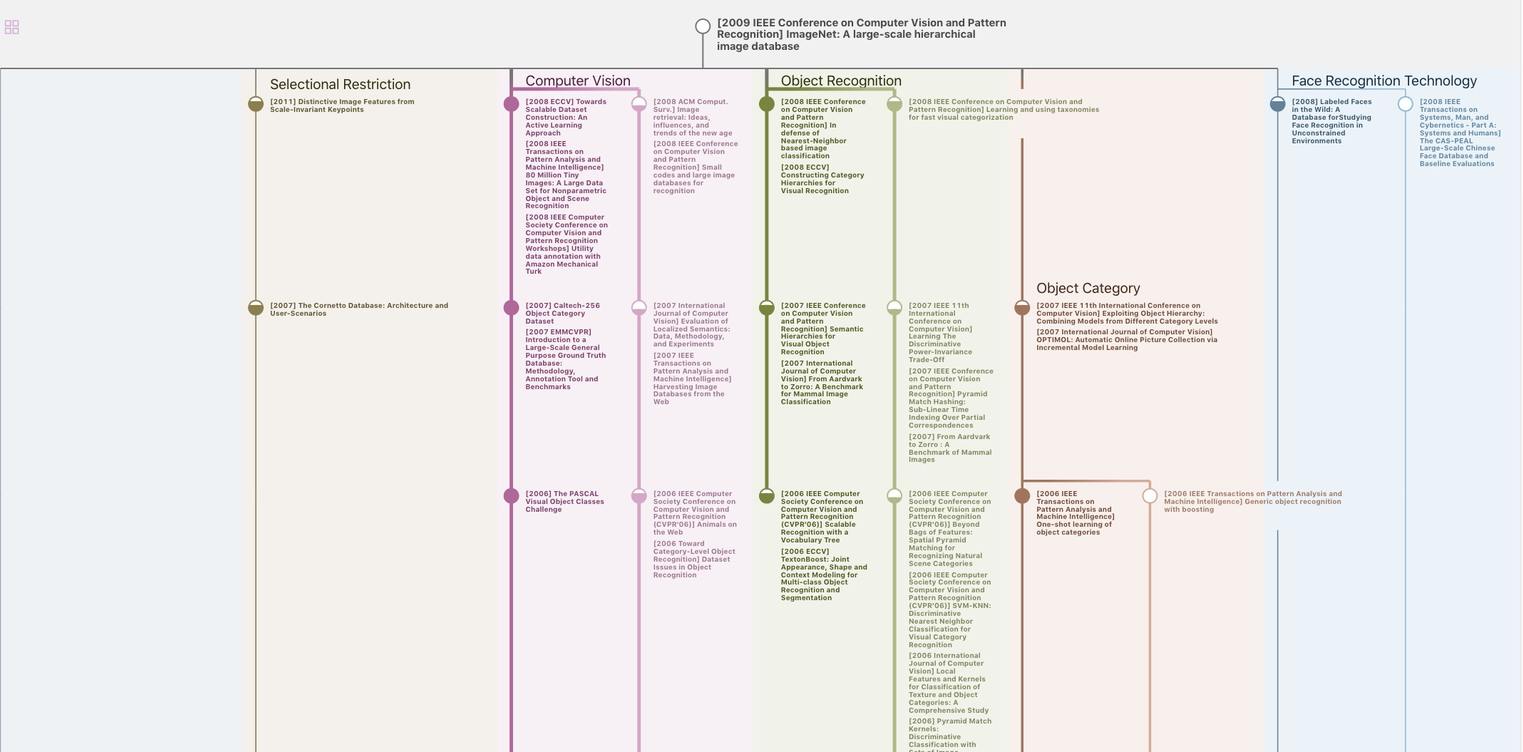
生成溯源树,研究论文发展脉络
Chat Paper
正在生成论文摘要