Use Of Machine Learning In The Prediction Of Emergency Department Management Of Patients With Urolithiasis
JOURNAL OF UROLOGY(2021)
Abstract
You have accessJournal of UrologyStone Disease: Epidemiology & Evaluation II (MP54)1 Sep 2021MP54-18 USE OF MACHINE LEARNING IN THE PREDICTION OF EMERGENCY DEPARTMENT MANAGEMENT OF PATIENTS WITH UROLITHIASIS Akhil A. Saji, John L. Phillips, Majid Eshghi, and Nathan C. Wong Akhil A. SajiAkhil A. Saji More articles by this author , John L. PhillipsJohn L. Phillips More articles by this author , Majid EshghiMajid Eshghi More articles by this author , and Nathan C. WongNathan C. Wong More articles by this author View All Author Informationhttps://doi.org/10.1097/JU.0000000000002084.18AboutPDF ToolsAdd to favoritesDownload CitationsTrack CitationsPermissionsReprints ShareFacebookLinked InTwitterEmail Abstract INTRODUCTION AND OBJECTIVE: The initial acute management of patients with urolithiasis is often conducted by non-urologists. We explored whether machine learning (ML) algorithms can be used to aid in the identification of patients who are appropriate for conservative management or prioritized for acute urologic intervention. METHODS: We performed a retrospective review of patients who presented to the emergency department and underwent initial observation with a diagnosis of urolithiasis at our tertiary center between January 2014 and October 2016. We trained six unique machine learning models with 27 different variables including patient demographics, clinical presentation, laboratory/imaging, initial management) in a hypothesis-free manner to build models that could predict need for urologic intervention. To create our ML models, the cohort data was randomly split into 80/20 training and test sub-cohorts with a respective intervention prevalence of 35.4% and 43.9%. RESULTS: A total of 137 patients were included in our study cohort, of which 52 (38%) were admitted to hospital and underwent urologic intervention (our predictive outcome of interest) including stent placement and ureteroscopy or percutaneous nephrolithotripsy. The other 85 patients were discharged and underwent outpatient management. The area under the curve for our models was highest with random forest (RF) (0.957) and gradient boost (GB) (0.918), followed by decision tree (0.792) and K-nearest neighbor (0.746), and then stochastic gradient descent (0.657) and naïve bayes (0.623). We also evaluated the predictive capacity of traditional statistic modeling with logistic regression (LR) as a comparator which had an AUC of 0.667 (Figure 1). CONCLUSIONS: Our machine learning algorithms may accurately predict the need for urologic intervention for patients with urolithiasis, particularly random forest and gradient boost classifiers which both outperformed traditional logistic regression. With further refinement and the addition of more data, these algorithms may be used by non-urologists to aid in the decision making process of these patients. Source of Funding: None © 2021 by American Urological Association Education and Research, Inc.FiguresReferencesRelatedDetails Volume 206Issue Supplement 3September 2021Page: e956-e957 Advertisement Copyright & Permissions© 2021 by American Urological Association Education and Research, Inc.MetricsAuthor Information Akhil A. Saji More articles by this author John L. Phillips More articles by this author Majid Eshghi More articles by this author Nathan C. Wong More articles by this author Expand All Advertisement Loading ...
MoreTranslated text
Key words
emergency department management,machine learning,emergency department,prediction
AI Read Science
Must-Reading Tree
Example
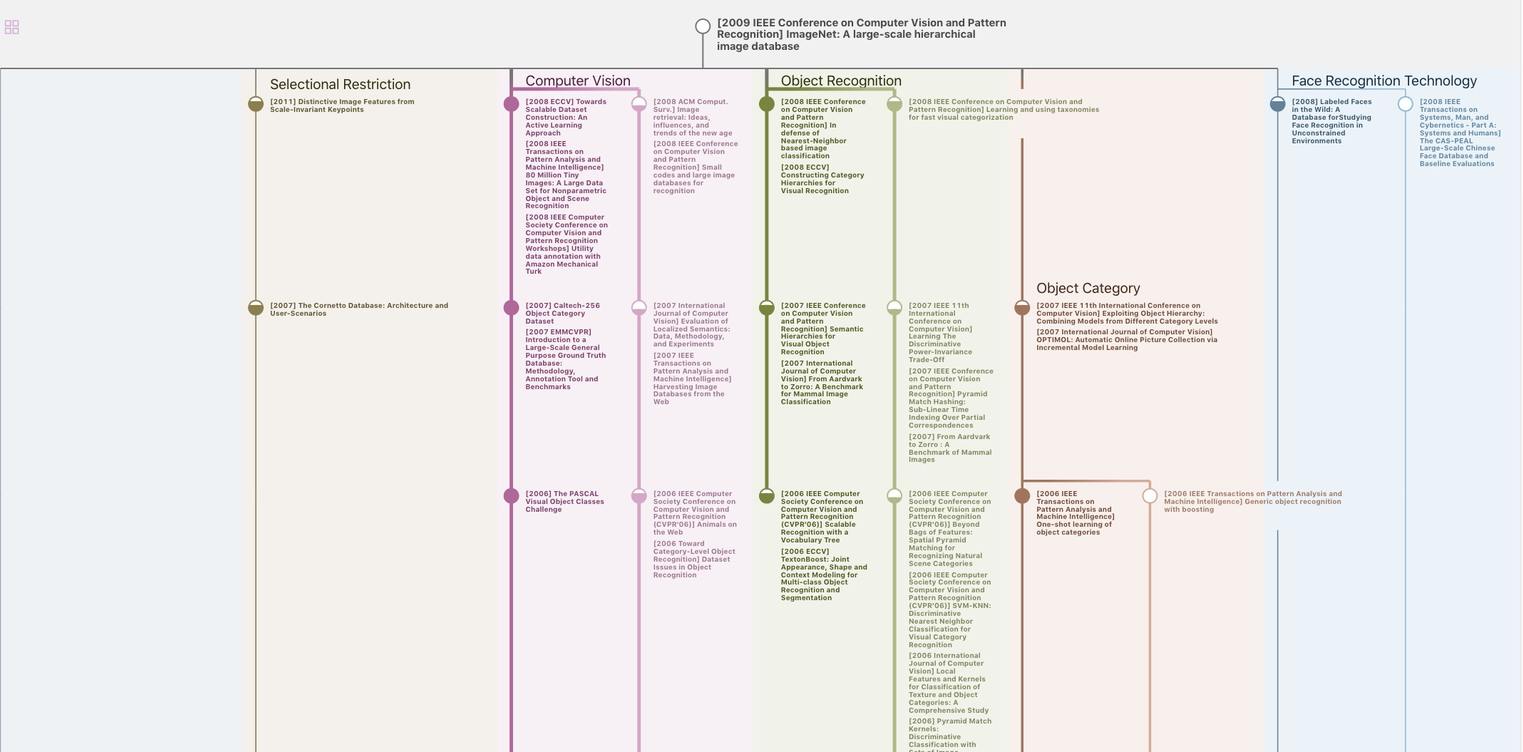
Generate MRT to find the research sequence of this paper
Chat Paper
Summary is being generated by the instructions you defined