An Improved Variable Kernel Density Estimator Based On L-2 Regularization
MATHEMATICS(2021)
摘要
The nature of the kernel density estimator (KDE) is to find the underlying probability density function (p.d.f) for a given dataset. The key to training the KDE is to determine the optimal bandwidth or Parzen window. All the data points share a fixed bandwidth (scalar for univariate KDE and vector for multivariate KDE) in the fixed KDE (FKDE). In this paper, we propose an improved variable KDE (IVKDE) which determines the optimal bandwidth for each data point in the given dataset based on the integrated squared error (ISE) criterion with the L-2 regularization term. An effective optimization algorithm is developed to solve the improved objective function. We compare the estimation performance of IVKDE with FKDE and VKDE based on ISE criterion without L-2 regularization on four univariate and four multivariate probability distributions. The experimental results show that IVKDE obtains lower estimation errors and thus demonstrate the effectiveness of IVKDE.
更多查看译文
关键词
probability density function, kernel density estimation, Parzen window, bandwidth, kernel function
AI 理解论文
溯源树
样例
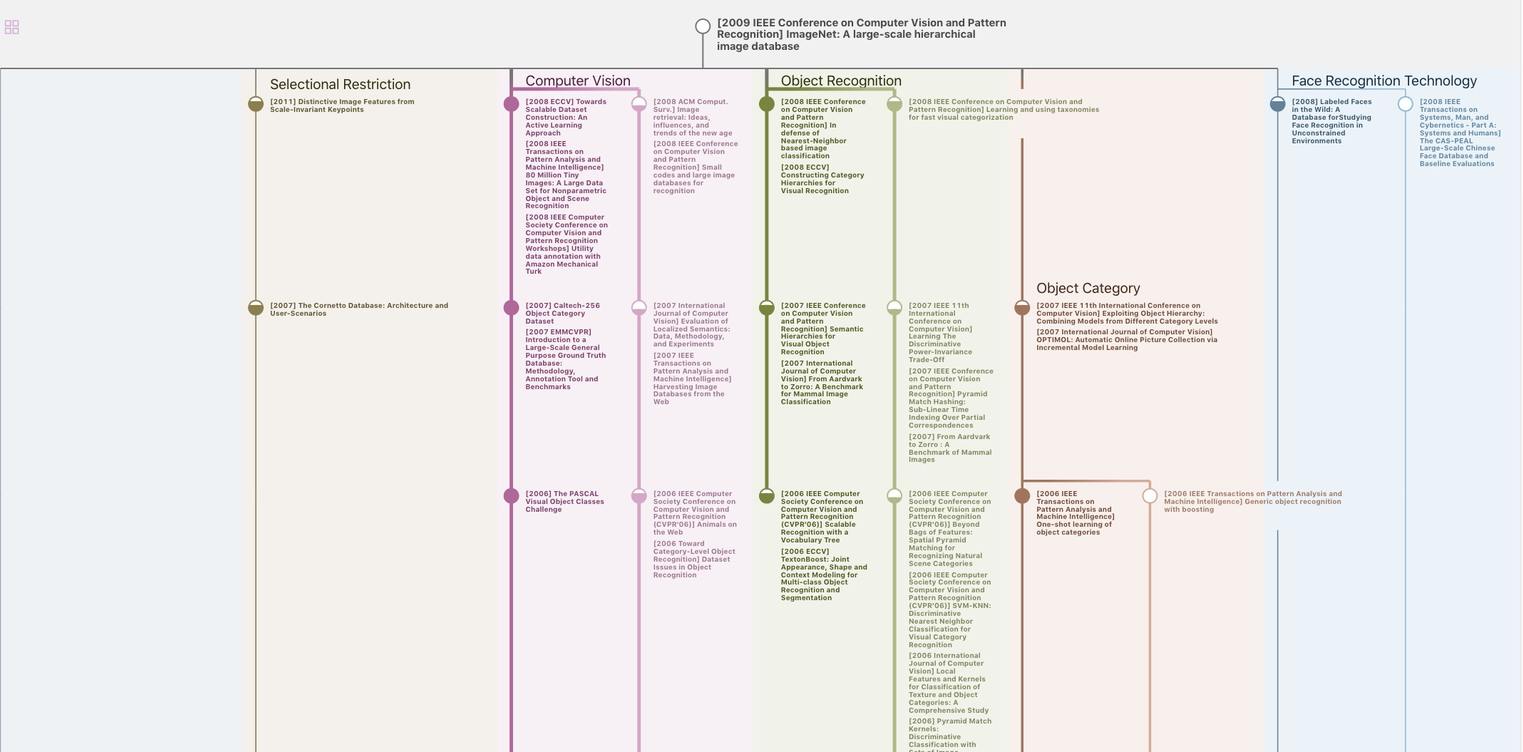
生成溯源树,研究论文发展脉络
Chat Paper
正在生成论文摘要