Evaluating causal-based feature selection for fuel property prediction models
STATISTICAL ANALYSIS AND DATA MINING(2021)
摘要
In-silico screening of novel biofuel molecules based on chemical and fuel properties is a critical first step in the biofuel evaluation process due to the significant volumes of samples required for experimental testing, the destructive nature of engine tests, and the costs associated with bench-scale synthesis of novel fuels. Predictive models are limited by training sets of few existing measurements, often containing similar classes of molecules that represent just a subset of the potential molecular fuel space. Software tools can be used to generate every possible molecular descriptor for use as input features, but most of these features are largely irrelevant and training models on datasets with higher dimensionality than size tends to yield poor predictive performance. Feature selection has been shown to improve machine learning models, but correlation-based feature selection fails to provide scientific insight into the underlying mechanisms that determine structure-property relationships. The implementation of causal discovery in feature selection could potentially inform the biofuel design process while also improving model prediction accuracy and robustness to new data. In this study, we investigate the benefits causal-based feature selection might have on both model performance and identification of key molecular substructures. We found that causal-based feature selection performed on par with alternative filtration methods, and that a structural causal model provides valuable scientific insights into the relationships between molecular substructures and fuel properties.
更多查看译文
关键词
Bayesian networks, causal inference, feature selection, QSPR
AI 理解论文
溯源树
样例
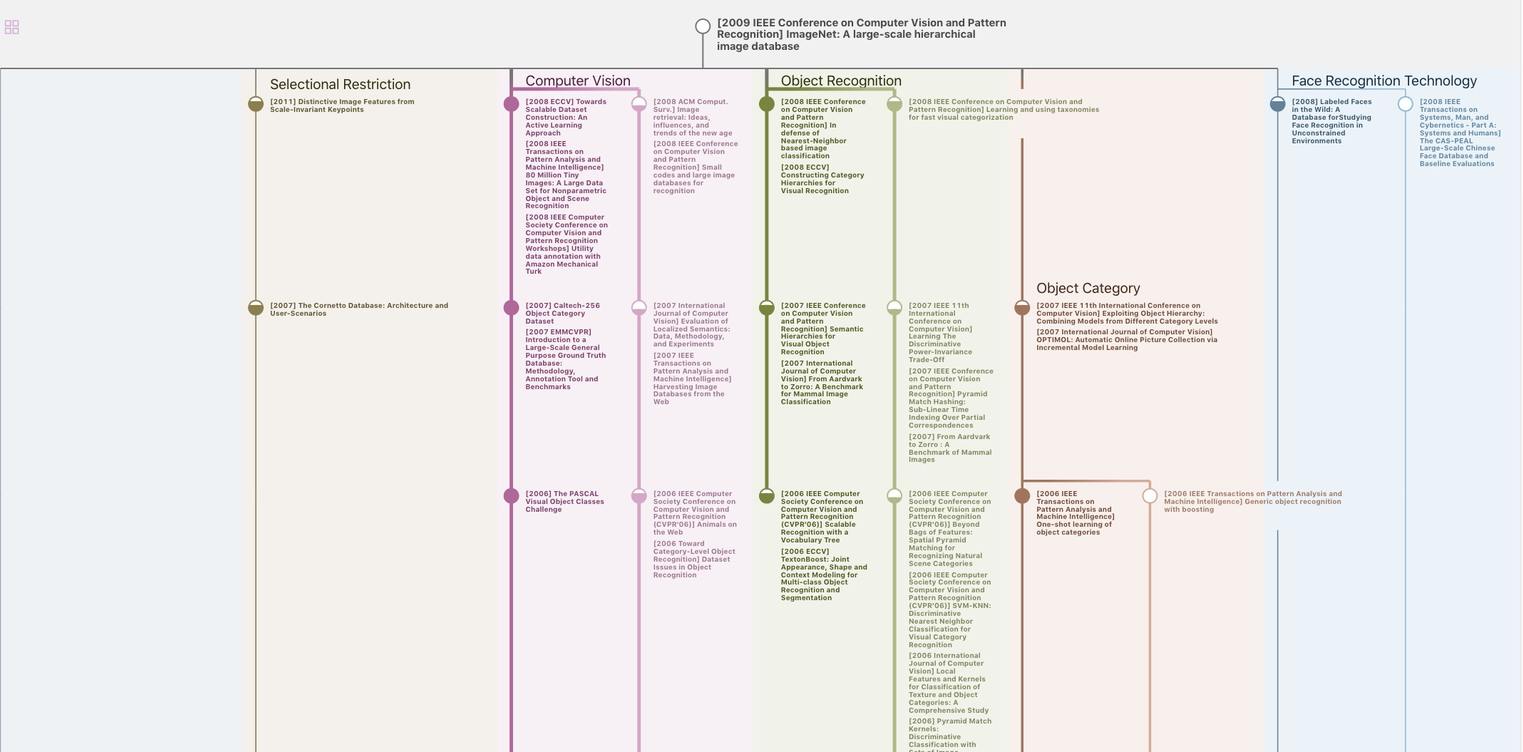
生成溯源树,研究论文发展脉络
Chat Paper
正在生成论文摘要