Closing The Loop - Finding Lung Cancer Patients Using Nlp
2017 IEEE INTERNATIONAL CONFERENCE ON BIG DATA (BIG DATA)(2017)
摘要
The average survival time for untreated primary carcinoma of the lung, from the onset of symptoms, is 8.2 months [1]. Lung cancer is the second most common cancer in both men and women. Early detection of malignancy and treatment of lung nodules increases chances of survival. In a medium to large sized health system, hundreds of chest, thorax, and abdominal CT scans are performed daily. Many of these scans are looking for problems with organs other than the lung. The radiologist, while reading the CT scans, makes notes about findings on pulmonary nodules-an incidental finding. Additional follow-up is often delayed or missed all together, as the pulmonary nodule was not the chief complaint for the visit or the reason for the imaging study. Geisinger's Close-the-Loop clinical program uses Natural Language Processing (NLP) of radiology notes to isolate pulmonary nodule mentions and the RADS score associated with the nodule to determine the urgency of follow-up recommendations. Close-the-Loop care managers, using information output from the NLP, contact patients for follow-up visits and treatments. The NLP algorithm is complicated by variations in style, content, context, and negations of the text being analyzed by the machine. The other complication arises with processing large number of notes efficiently and in a timely manner. Geisinger's lung nodule NLP solution analyzed millions of radiology notes and found thousands of patients with need for follow-up care with a validated accuracy of 90%.
更多查看译文
关键词
lung nodule, natural language processing, cancer, NLP, hospital notes mining, healthcare
AI 理解论文
溯源树
样例
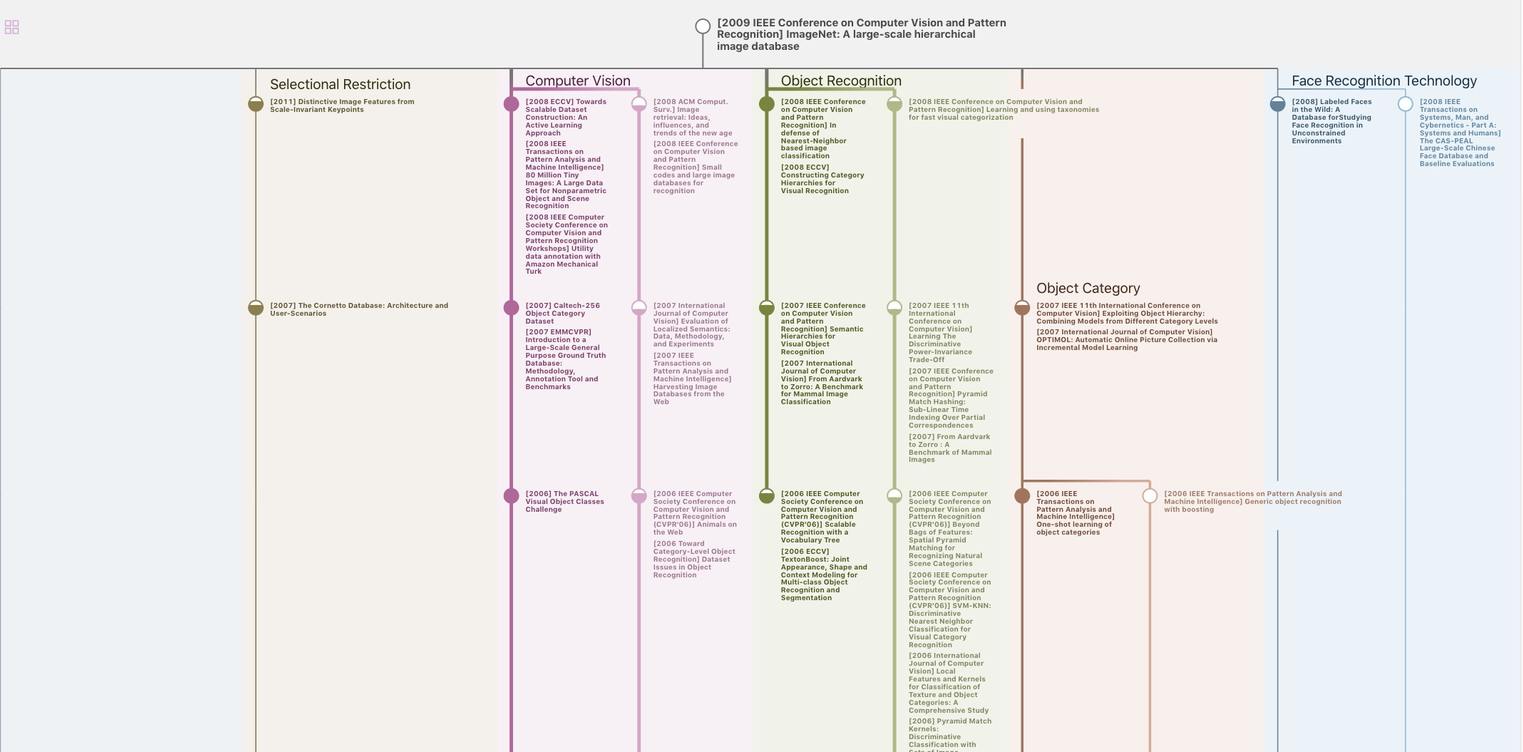
生成溯源树,研究论文发展脉络
Chat Paper
正在生成论文摘要