Efficient Nested Modifier Adaptation For Rto Using Lagrangian Functions
27TH EUROPEAN SYMPOSIUM ON COMPUTER AIDED PROCESS ENGINEERING, PT B(2017)
Abstract
Real-Time Optimization (RTO) is not always able to achieve its targets due to the presence of plant-model mismatch. Modifier Adaptation (MA) overcomes this issue introducing modifiers for the cost and constraint functions of the economic optimization problem to bring the process to the optimum operating point. Several applications have shown the effectiveness of this technique, however, the size of the problem with respect to the number of decision variables and the number of constraints is still an open issue because it increases the number of modifiers, slowing down the convergence to the optimum. For dealing with process-dependent constraints, an alternative Nested Modifier Adaptation technique (NMA) can be implemented which uses the Lagrangian function and thus only one gradient modifier for each process input is required, speeding up the convergence to the real optimum.
MoreTranslated text
Key words
Real-Time Optimization, Modifier Adaptation, uncertainty
AI Read Science
Must-Reading Tree
Example
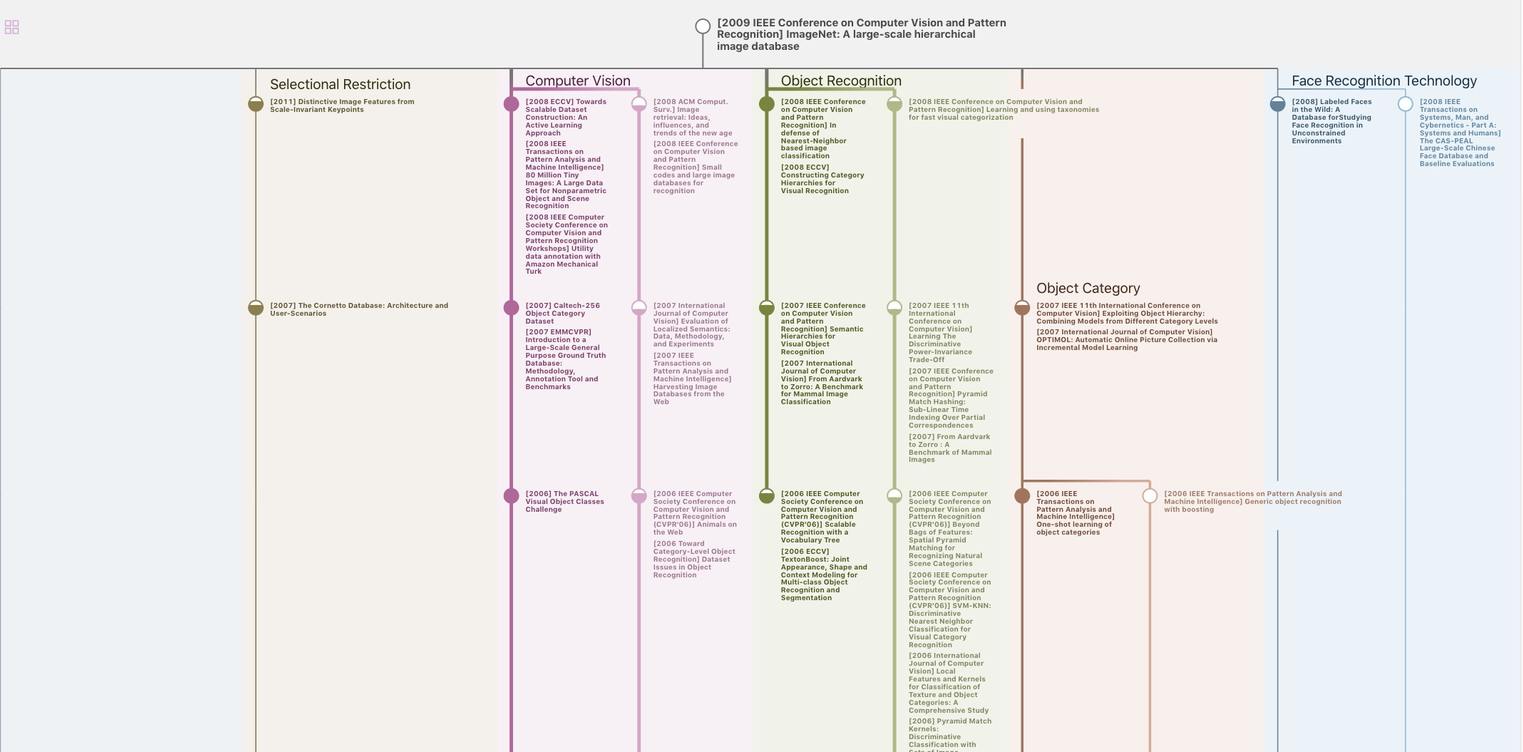
Generate MRT to find the research sequence of this paper
Chat Paper
Summary is being generated by the instructions you defined