Evaluating Several Ways To Combine Handcrafted Feature-Based Systems With A Deep Learning System For The Luna16 Challenge Framework
MEDICAL IMAGING 2020: COMPUTER-AIDED DIAGNOSIS(2020)
Abstract
Computer aided diagnosis systems are used to assist radiologists in their decision making. The sensitivity of these systems is hindered by the complexity of the structures inside the lungs. Several systems and methods have been proposed to detect and classify lung nodules, but all of them have their strengths and weaknesses. One way to overcome the weaknesses is to combine multiple systems. Systems based on handcrafted features capture a limited set of characteristics from the image, while deep learning based classifiers can deal with a wider range of structures. In this work, several ways to combine a handcrafted feature based classifier with four convolutional neural network are explored. The systems were combined merging the probabilities assigned to the detections in several ways. Support-vector machine, multilayer perceptron and random forest classifiers were used to combine the selected classifiers. The LUNA16 Challenge was used to evaluate the performance of the resulting hybrid systems. In all cases, the hybrid systems outperformed the individual systems. Although the average of sensitivities are similar for most of the combinations, the best hybrid system achieves a gain of 35 extra nodules at 4 FP per scan.
MoreTranslated text
Key words
convolutional neural netwoks, support vector machine, fusion, random forest, multilayer perceptron, computer aided diagnosis, LUNA16
AI Read Science
Must-Reading Tree
Example
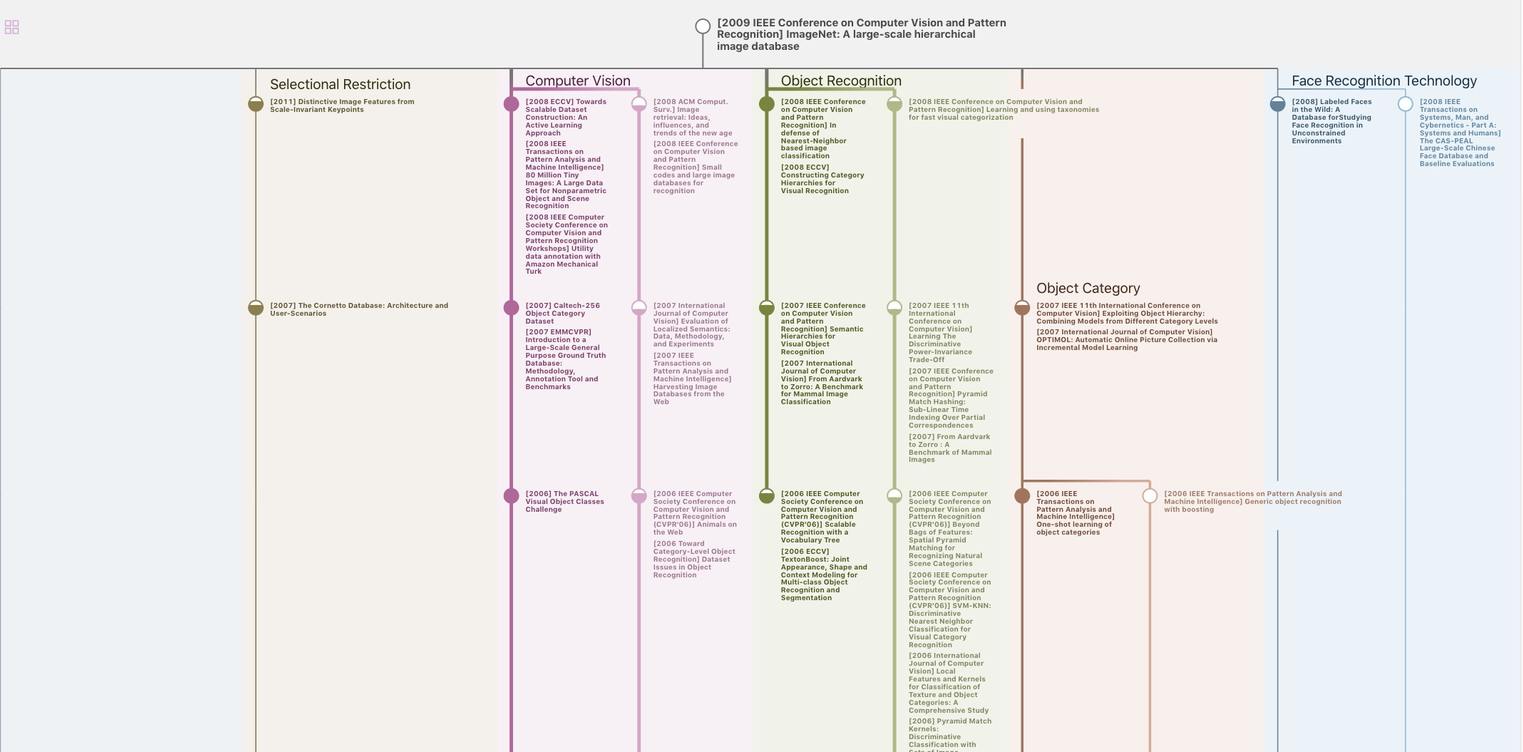
Generate MRT to find the research sequence of this paper
Chat Paper
Summary is being generated by the instructions you defined