Predicting The Concentration Of Sulfate (So42-) In Drinking Water Using Artificial Neural Networks: A Case Study: Medea-Algeria
DESALINATION AND WATER TREATMENT(2021)
Abstract
The aim of this work was to use artificial neural networks (ANN) and multiple linear regressions (MLR) models to predict the soluble sulfate content in drinking water. A set of 84 data points were used. For the ANN, 18 neurons were used in the input layer, 8 neurons at hidden layer, and 1 was used in the output layer. Levenberg Marquardt learning (LM) algorithm with hyperbolic tangent sigmoid transfer function logarithmic was used at the hidden and output layer. The comparison of the obtained results in term of root mean square error (RMSE) and correlation coefficient (R) using the ANN and MLR models revealed the superiority of the (ANN) model in predicting the soluble sulfate content in drinking water. Indeed, the statistical results showed a correlation coefficient R = 0.99973 with RMSE = 5.9755 for the ANN model and R = 0.941 with RMSE = 88.3068 for the MLR model. A nonlinear relationship between the soluble sulfate content and the physico-chemical characteristics of drinking water (conductivity, turbidity, potential hydrogen, hardness, calcium, magnesium, chlorides, total alkali metric titre, material organic, nitrogen dioxide, nitrates, sodium, bicarbonate, potassium, heavy metals (Mn2+, Fe3+, and Al+) and dry residues) was demonstrated, showing that the soluble sulfate content concentration can be predicted.
MoreTranslated text
Key words
Drinking water, Physico-chemical parameters, Sulfate, Modeling, Artificial neural networks, Multiple linear regressions
AI Read Science
Must-Reading Tree
Example
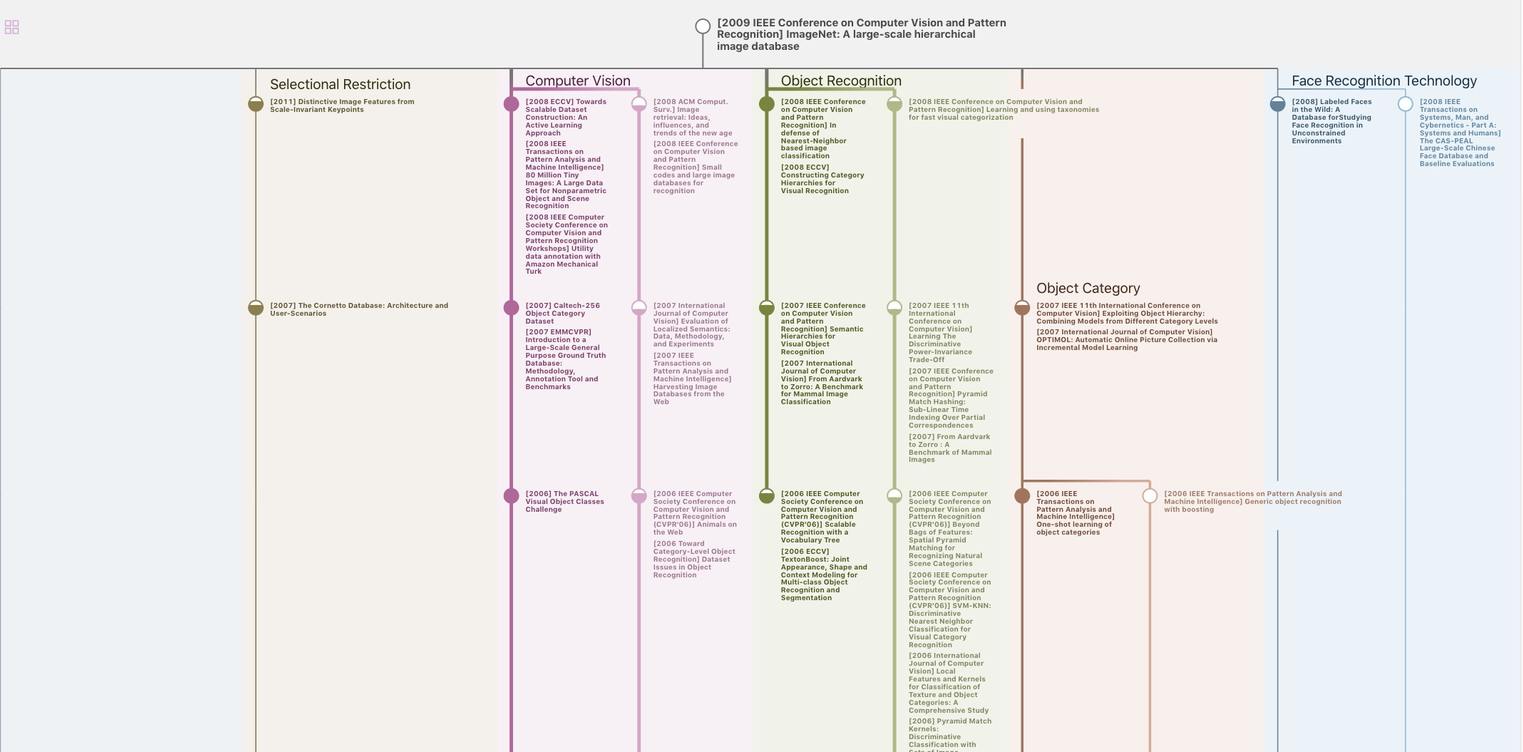
Generate MRT to find the research sequence of this paper
Chat Paper
Summary is being generated by the instructions you defined