Risk Bounds For Unsupervised Cross-Domain Mapping With Ipms
JOURNAL OF MACHINE LEARNING RESEARCH(2021)
摘要
The recent empirical success of unsupervised cross-domain mapping algorithms, in mapping between two domains that share common characteristics, is not well-supported by theoretical justifications. This lacuna is especially troubling, given the clear ambiguity in such mappings.We work with adversarial training methods based on integral probability metrics (IPMs) and derive a novel risk bound, which upper bounds the risk between the learned mapping h and the target mapping y, by a sum of three terms: (i) the risk between h and the most distant alternative mapping that was learned by the same cross-domain mapping algorithm, (ii) the minimal discrepancy between the target domain and the domain obtained by applying a hypothesis h* on the samples of the source domain, where h* is a hypothesis selectable by the same algorithm, and (iii) an approximation error term that decreases as the capacity of the class of discriminators increases and is empirically shown to be small. The bound is directly related to Occam's razor and encourages the selection of the minimal architecture that supports a small mapping discrepancy.The bound leads to multiple algorithmic consequences, including a method for hyperparameter selection and early stopping in cross-domain mapping.
更多查看译文
关键词
unsupervised learning, cross-domain alignment, integral probability metrics, adversarial training, image to image translation
AI 理解论文
溯源树
样例
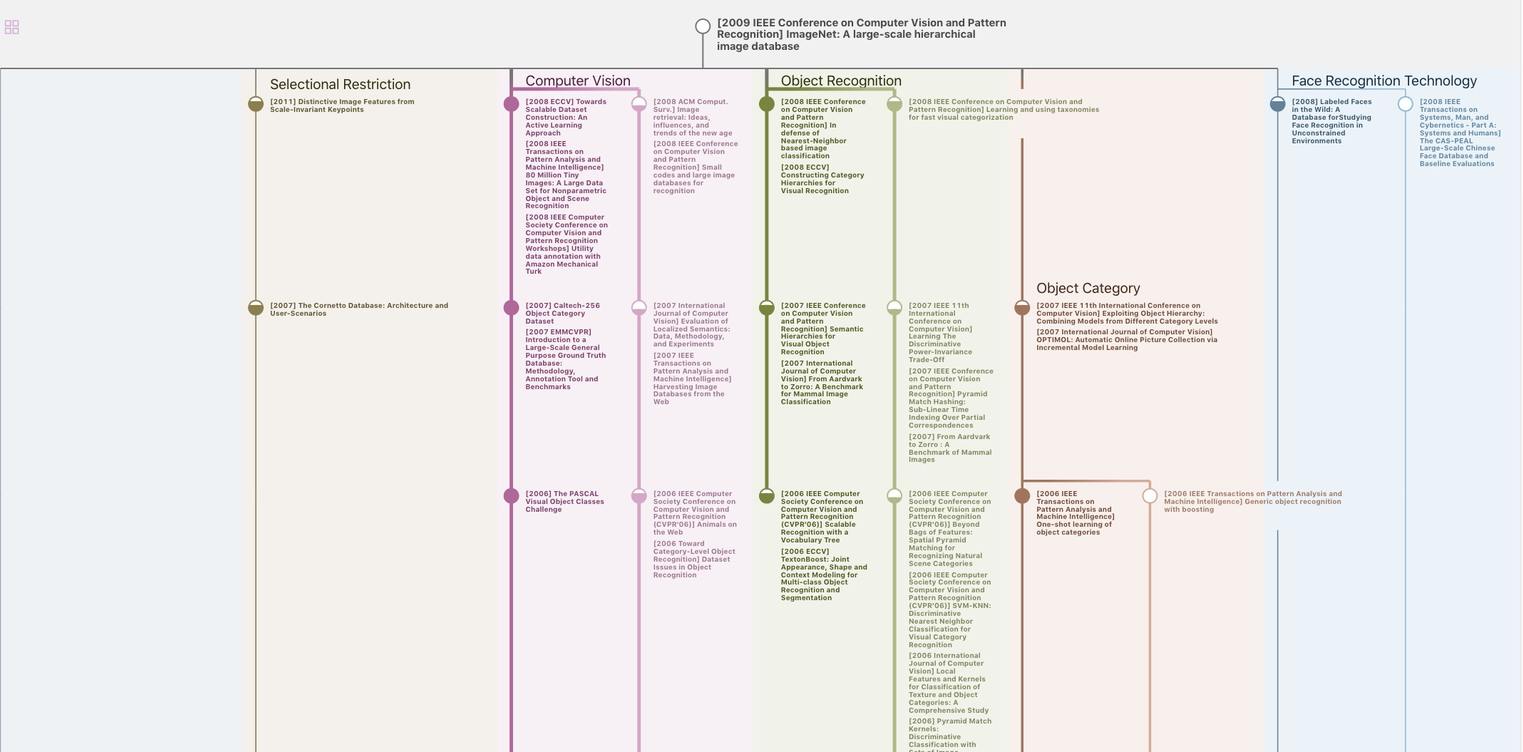
生成溯源树,研究论文发展脉络
Chat Paper
正在生成论文摘要