Investigating The Impact Of Perturbations In Chemical Processes On Data-Based Causality Analysis. Part 1: Defining Desired Performance Of Causality Analysis Techniques
IFAC PAPERSONLINE(2017)
摘要
In large-scale chemical and mineral processes, processing units are connected to each other through material flow, energy flow, or information flow (manifested by control loops). Data-based causality analysis may be may be employed to infer the cause-effect connections between measured variables. In the last decade research into the application of causality analysis approach to chemical processes has proven these data-based techniques to be useful, especially for fault diagnosis. However, the impact of perturbations caused by different process conditions on the ability of these techniques to identify causality has not been thoroughly investigated. Improved understanding of the strengths and weaknesses of these techniques for causality detection under different conditions is essential for streamlining their automated application. In this two-part paper, the impact of perturbations generated by conditions typical to chemical processes is analysed. In this first part, causality analysis techniques are reviewed with the intent to define the desired characteristics and desired causality detection performance of the techniques. In the second part a simple process simulation used to introduce perturbations and analyse their impact on the causality detection ability of transfer entropy and Granger causality. (C) 2017, IFAC (International Federation of Automatic Control) Hosting by Elsevier Ltd. All rights reserved.
更多查看译文
关键词
Process Performance Monitoring, Fault Detection and Diagnosis, Causality Analysis, Root Cause Analysis, Fault Propagation, Statistical methods/signal analysis for FDI
AI 理解论文
溯源树
样例
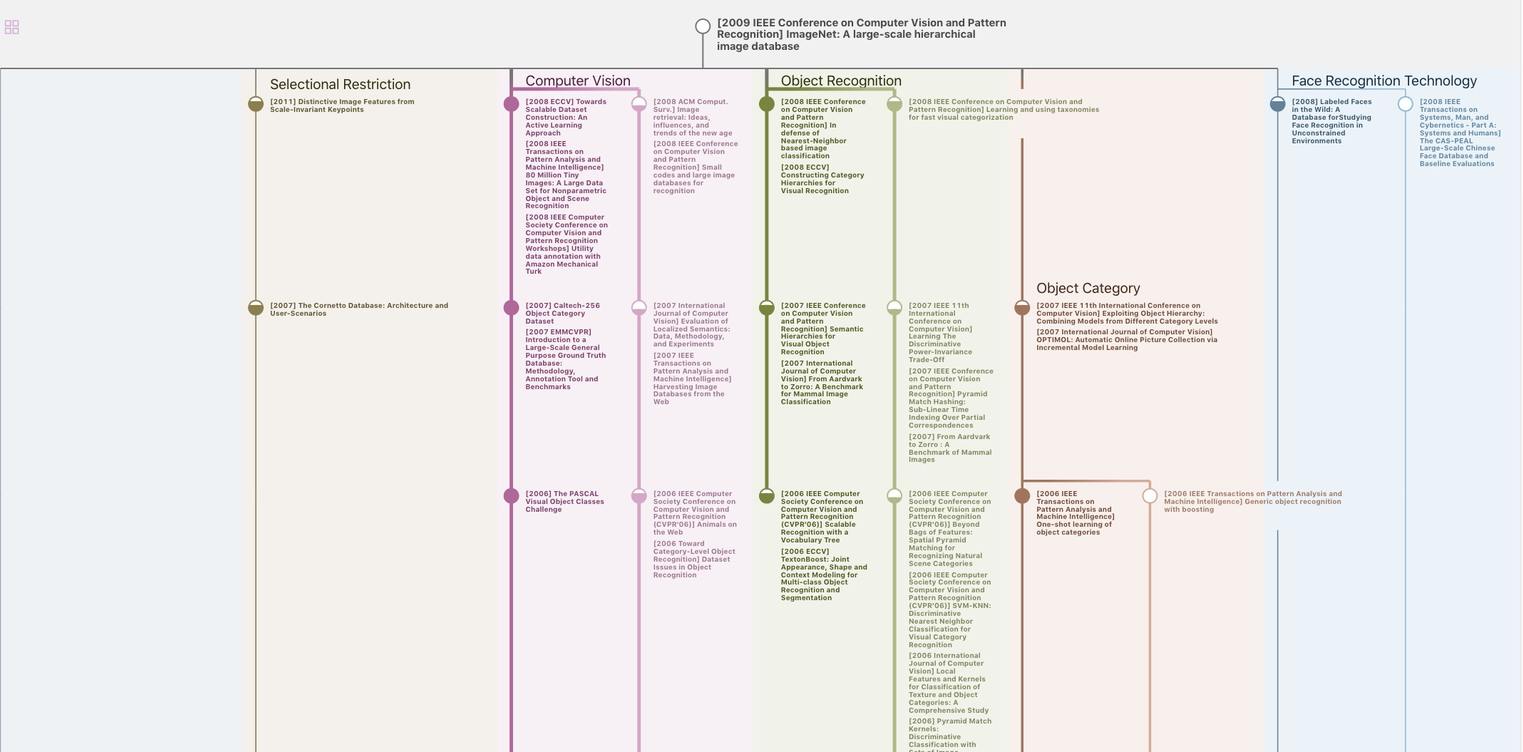
生成溯源树,研究论文发展脉络
Chat Paper
正在生成论文摘要