Seq-SymRF: A random forest model predict potential miRNA-disease associations based on information of sequences and clinical symptoms
crossref(2019)
摘要
Abstract Background: MicroRNA (MiRNA) plays a vital role in biological processes and closely relate with various human diseases. Research on the miRNA-disease associations contributes to the prevention, diagnosis and treatment of diseases, as well as the identification of new drugs and the discovery of lead compounds. Since traditional experiment methods are time-consuming and expensive, it is necessary to develop an efficient and accurate theoretical approach to identify potential miRNA-disease associations. Results: In this study, a sequence- and symptom-based random forest classifier model (Seq-SymRF) was constructed to identify the potential associations between miRNA and disease. Compared with existing methods, features derived from only sequence information were used to characterize miRNA. More importantly, clinical symptoms were utilized to represent disease. Moreover, the clustering method by calculating the Euclidean distance was implemented to construct the reliable negative sample. Based on the five-fold cross-validation, the model achieved the accuracy, specificity, sensitivity, precision and Matthews correlation coefficient of 98.00%, 99.43%, 96.58%, 99.40% and 0.9604, respectively. The areas under receiver operating characteristic curve and precision recall curve were 0.9967 and 0.9975, respectively. Additionally, case study was implemented with leukemia, breast neoplasms and hsa-mir-21. Most of top-25 predicted disease-related miRNAs (36/50 for leukemia; 33/50 for breast neoplasms) and 32 of top-50 predicted miRNA-related diseases were verified by literature and dbDEMC database. Conclusion: We proposed a new method, named Seq-SymRF, for predicting miRNA-disease associations, which could be regarded as a powerful high-throughput virtual screening tool for drug research and development.
更多查看译文
AI 理解论文
溯源树
样例
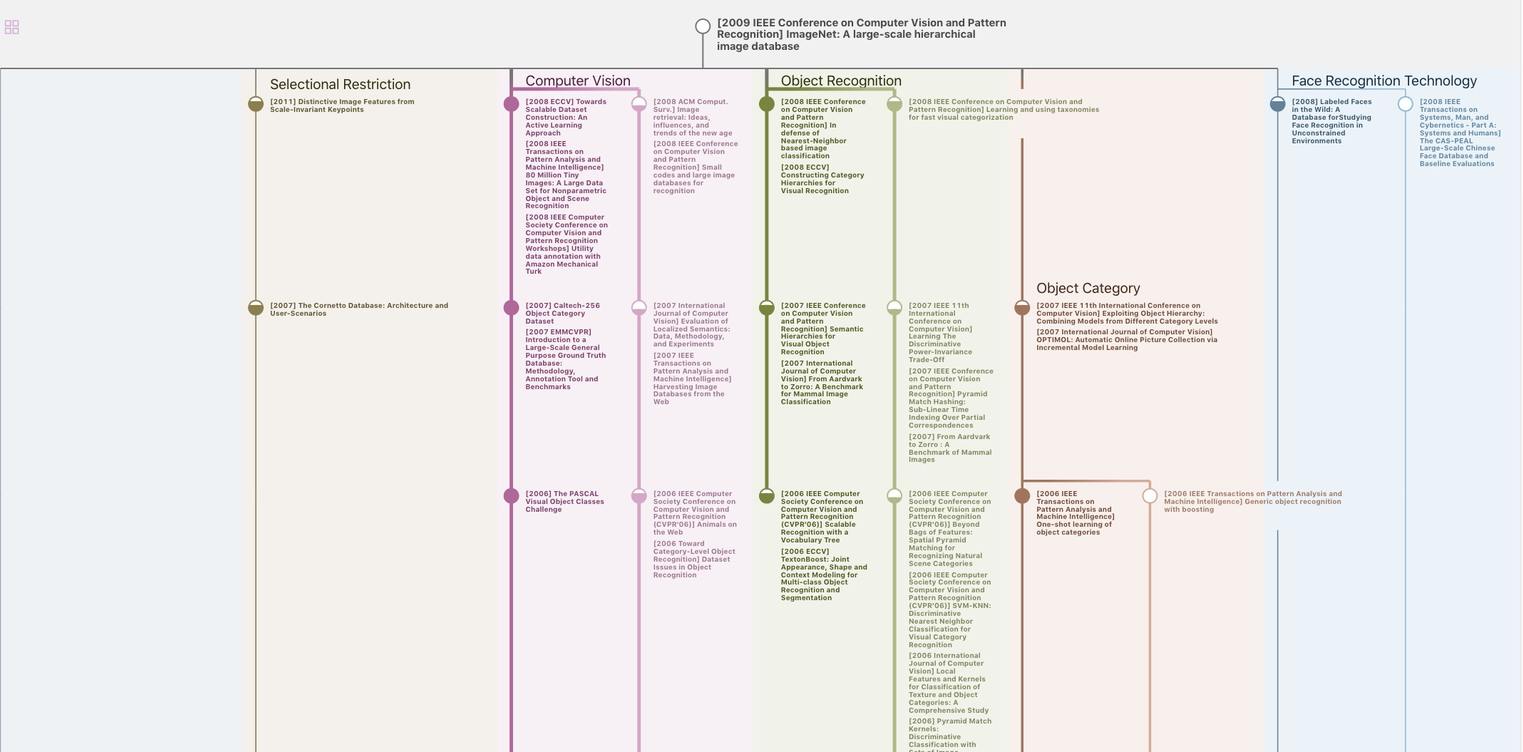
生成溯源树,研究论文发展脉络
Chat Paper
正在生成论文摘要