A Multimodal Adversarial Attack Framework Based on Local and Random Search Algorithms
INTERNATIONAL JOURNAL OF COMPUTATIONAL INTELLIGENCE SYSTEMS(2021)
摘要
Although many problems in computer vision and natural language processing have made breakthrough progress with neural networks, adversarial attack is a serious potential problem in many neural network-based applications. Attackers can mislead classifiers with slightly perturbed examples, which are called adversarial examples. As the existing adversarial attacks are specific to application and have difficulty in general usage, we propose a multimodal adversarial attack framework to attack both text and image classifiers. The proposed framework firstly generates candidate set to find the substitution words or pixels and generate candidate adversarial examples. Secondly, the framework updates candidate set and search adversarial examples with three local or random search methods [beam search, genetic algorithm (GA) search, particle swarm optimization (PSO) search]. The experiments demonstrate that the proposed framework effectively generates image and text adversarial examples. Comparing the proposed methods with other image adversarial attacks in MNIST dataset, the PSO search in the framework has 98.4% attack success rate which outperforms other methods. Besides, the beam search has the best attack efficiency and human imperception in both MNIST and CIFAR-10 dataset. Comparing with other text adversarial attacks, the beam search in the framework has an attack success rate of 91.5%, which outperforms other existing and the proposed search methods. In attack efficiency, the beam search also outperforms other methods, meaning that we can craft text adversarial examples with less perturbation using beam search. (c) 2021 The Authors. Published by Atlantis Press B.V. This is an open access article distributed under the CC BY-NC 4.0 license (http://creativecommons.org/licenses/by-nc/4.0/).
更多查看译文
关键词
Adversarial attack,Multimodal applications,Adversarial image,Adversarial text,Local search,Random search
AI 理解论文
溯源树
样例
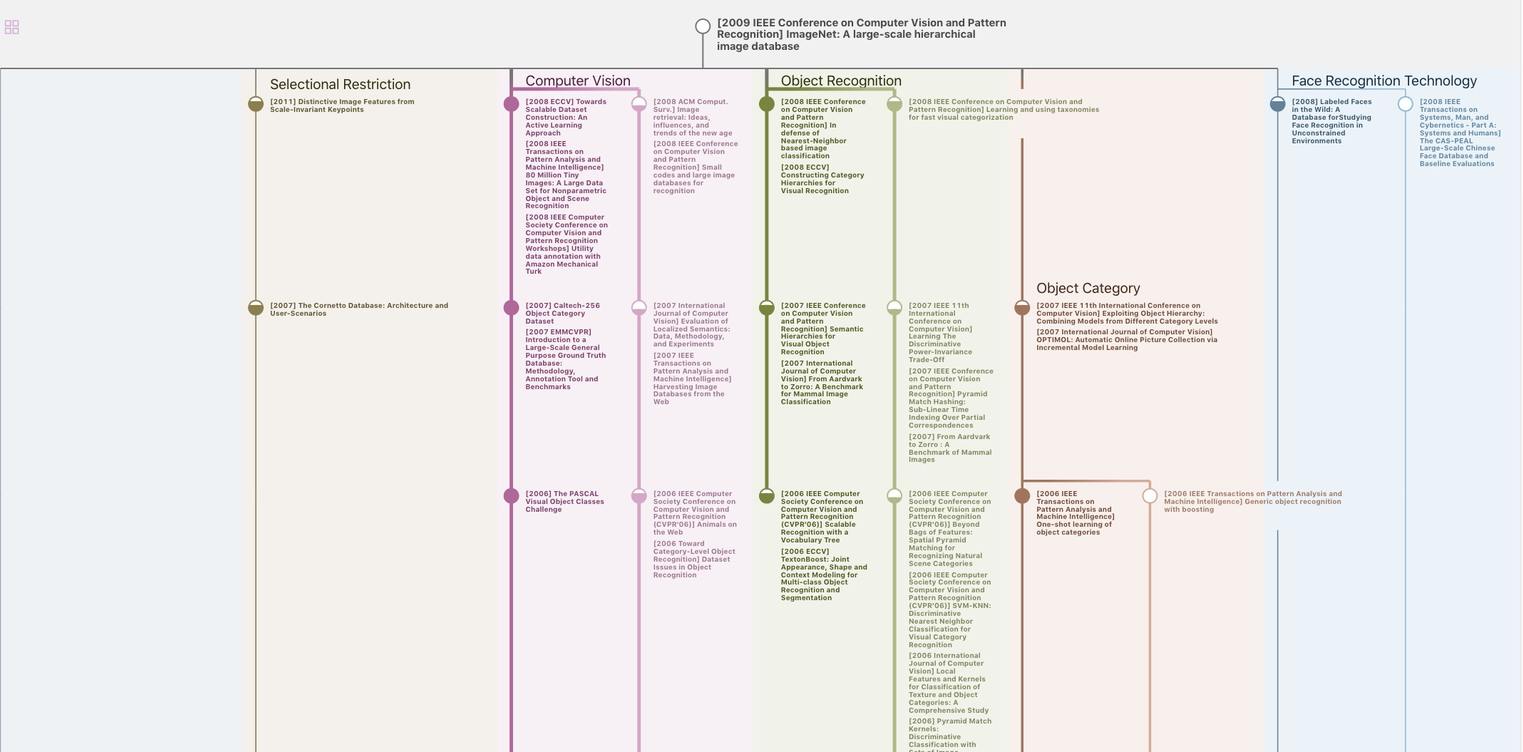
生成溯源树,研究论文发展脉络
Chat Paper
正在生成论文摘要