Missing Data Estimation in a Low-Cost Sensor Network for Measuring Air Quality: a Case Study in Aburrá Valley
WATER AIR AND SOIL POLLUTION(2021)
摘要
According to the World Health Organization (WHO), air pollution is currently one leading cause of death around the world. As a result, some projects have emerged to monitor air quality through the implementation of low-cost Wireless Sensor Networks (WSNs). However, the type of technology and the sensors’ location have an impact on data quality, resulting in a considerable amount of missing data. This hinders the proper implementation of methodologies for sensor calibration and data leverage for dispersion analysis of pollutants and prediction of pollution episodes. This paper presents a methodology based on matrix factorization (MF) to recover missing data from a low-cost WSN for particulate matter PM2.5 measurement. Using the proposed methodology with the study case in Aburrá Valley, Colombia, it is shown that is possible to recover 40 % missing data with less than 12 % errors, obtaining better results than those presented by other methods found in the literature.
更多查看译文
关键词
Matrix factorization, Machine learning, Low-cost sensors network, Missing data estimation
AI 理解论文
溯源树
样例
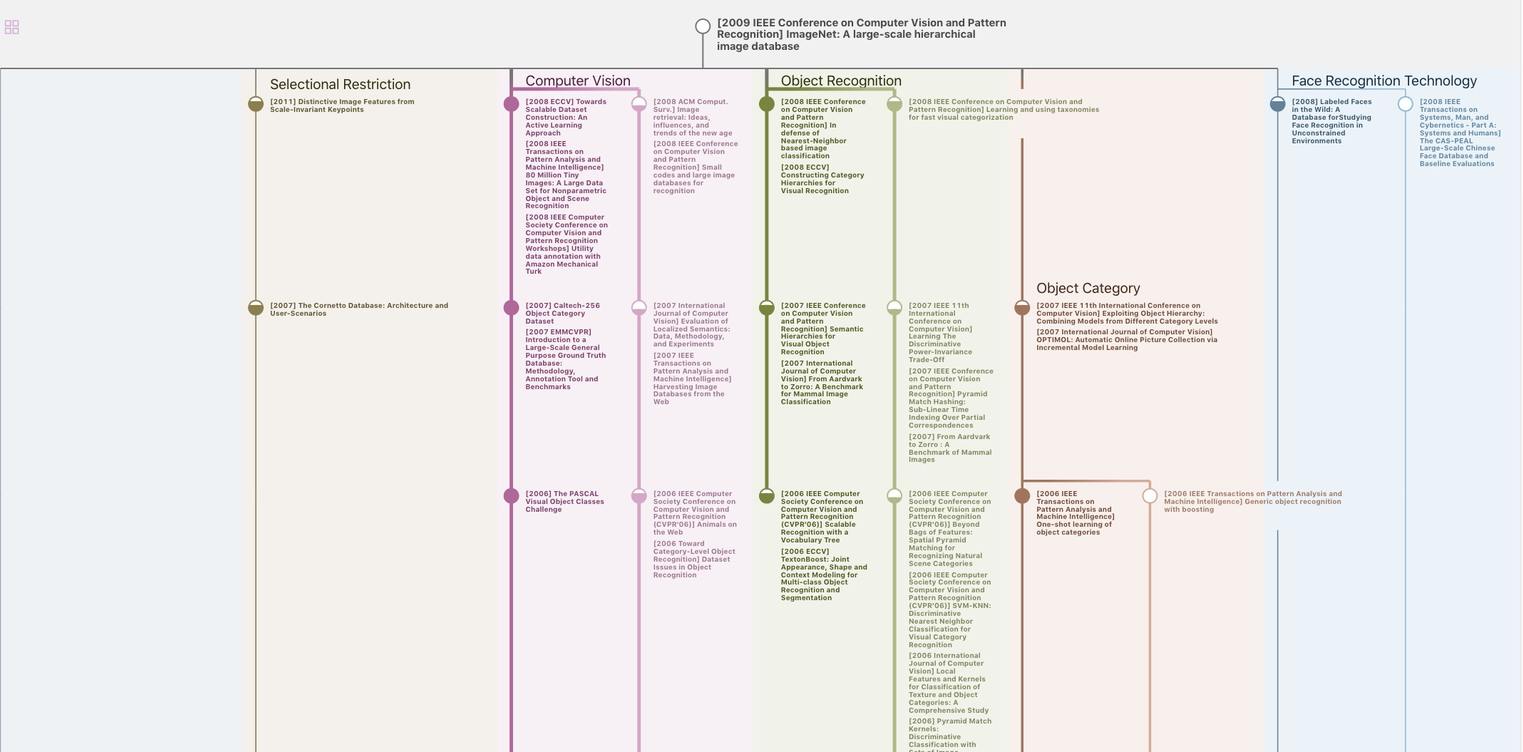
生成溯源树,研究论文发展脉络
Chat Paper
正在生成论文摘要