Anti-Jamming Game to Combat Intelligent Jamming for Cognitive Radio Networks
IEEE ACCESS(2021)
摘要
Cognitive Radio (CR) provides a promising solution to the spectrum scarcity problem in dense wireless networks, where the sensing ability of cognitive users helps acquire knowledge of the environment. However, cognitive users are vulnerable to different types of attacks, due to its shared medium. In particular, jamming is considered as one of the most challenging security threats in CR networks. In jamming, an attacker jams the communication by transmitting a high power noise signal in the vicinity of the targeted node. The jammer could be an intelligent entity that is capable of exploiting the dynamics of the environment. In this work, we provide a machine-learning-based anti-jamming technique for CR networks to avoid a hostile jammer, where both the jamming and anti-jamming processes are formulated based on the Markov game framework. In our framework, secondary users avoid the jammer by maximizing its payoff function using an online, model-free reinforcement learning technique called Q-learning. We consider a realistic mathematical model, where the channel conditions are time-varying and differ from one sub-channel to another, as in practical scenarios. Simulation results show that our proposed approach outperforms existing approaches to combat jamming over a wide range of scenarios.
更多查看译文
关键词
Jamming,Games,Cognitive radio,Wireless sensor networks,Reinforcement learning,Spread spectrum communication,Markov processes,Cognitive radio networks,anti-jamming communication,stochastic game,machine learning,multi-agent reinforcement learning,Q learning
AI 理解论文
溯源树
样例
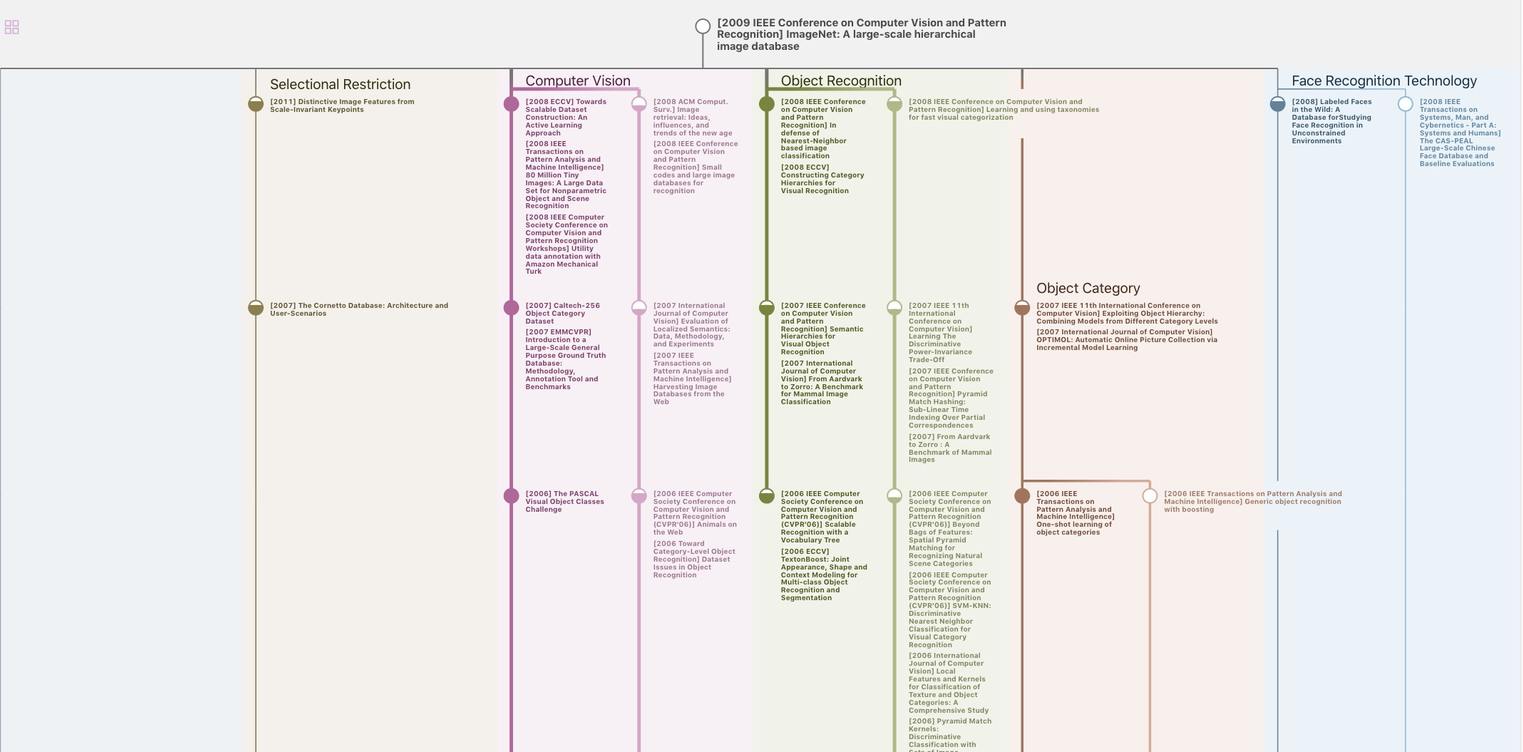
生成溯源树,研究论文发展脉络
Chat Paper
正在生成论文摘要