Adaptive System Identification via Low-Rank Tensor Decomposition
IEEE ACCESS(2021)
摘要
Tensor-based estimation has been of particular interest of the scientific community for several years now. While showing promising results on system estimation and other tasks, one big downside is the tremendous amount of computational power and memory required - especially during training - to achieve satisfactory performance. We present a novel framework for different classes of nonlinear systems, that allows to significantly reduce the complexity by introducing a least-mean-squares block before, after, or between tensors to reduce the necessary dimensions and rank required to model a given system. Our simulations show promising results that outperform traditional tensor models, and achieve equal performance to comparable algorithms for all problems considered while requiring significantly less operations per time step than either of the state-of-the-art architectures.
更多查看译文
关键词
Tensors,Approximation algorithms,Adaptive systems,Complexity theory,Adaptation models,Task analysis,Signal processing algorithms,Tensor decomposition,LMS,machine learning,low rank approximation
AI 理解论文
溯源树
样例
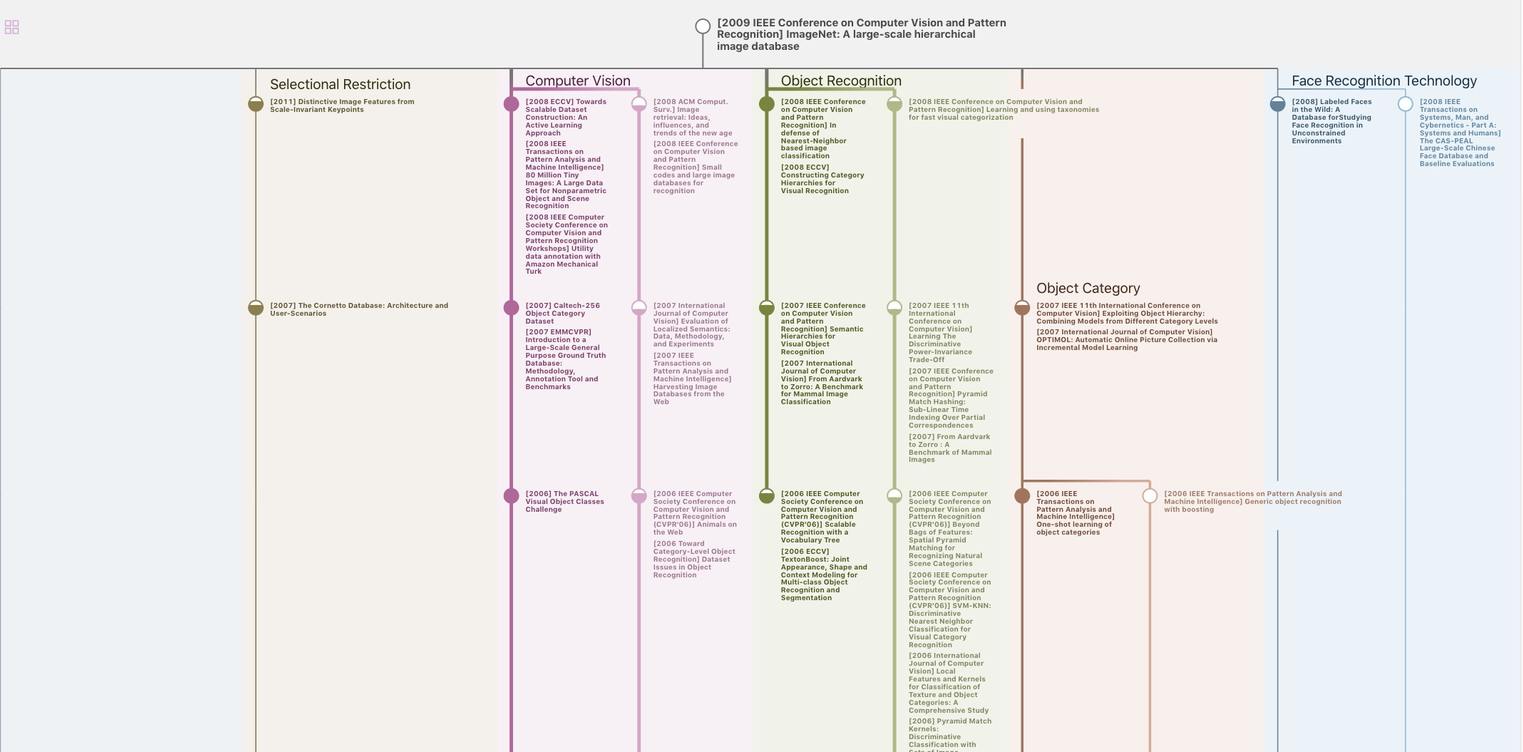
生成溯源树,研究论文发展脉络
Chat Paper
正在生成论文摘要