Experimental Analysis And Prediction Model Of Milling-Induced Residual Stress Of Aeronautical Aluminum Alloys
APPLIED SCIENCES-BASEL(2021)
Abstract
Featured Application This paper proposes a prediction model for milling residual stress based on a BP neural network. It aims to provide basic data calculation tools for analyzing the influence of milling residual stress on workpiece deformation, crack resistance, fatigue life, and corrosion resistance. Unlike traditional forecasting models, the prediction model is based on actual machining conditions. The prediction model contains the influence of various hidden factors, so it is closer to actual production. Additionally, the proposed model has a high prediction accuracy because of the strong nonlinear mapping ability of the BP neural network. Aeronautical thin-walled frame workpieces are usually obtained by milling aluminum alloy plates. The residual stress within the workpiece has a significant influence on the deformation due to the relatively low rigidity of the workpiece. To accurately predict the milling-induced residual stress, this paper describes an orthogonal experiment for milling 7075 aluminum alloy plates. The milling-induced residual stress at different surface depths of the workpiece, without initial stress, is obtained. The influence of the milling parameters on the residual stress is revealed. The parameters include milling speed, feed per tooth, milling width, and cutting depth. The experimental results show that the residual stress depth in the workpiece surface is within 0.12 mm, and the residual stress depth of the end milling is slightly greater than that of the side milling. The calculation models of residual stress and milling parameters for two milling methods are formulated based on regression analysis, and the sensitivity coefficients of parameters to residual stress are calculated. The residual stress prediction model for milling 7075 aluminum alloy plates is proposed based on a back-propagation neural network and genetic algorithm. The findings suggest that the proposed model has a high accuracy, and the prediction error is between 0-14 MPa. It provides basic data for machining deformation prediction of aluminum alloy thin-walled workpieces, which has significant application potential.
MoreTranslated text
Key words
aeronautical aluminum alloy, residual stress, prediction model, back-propagation neural network
AI Read Science
Must-Reading Tree
Example
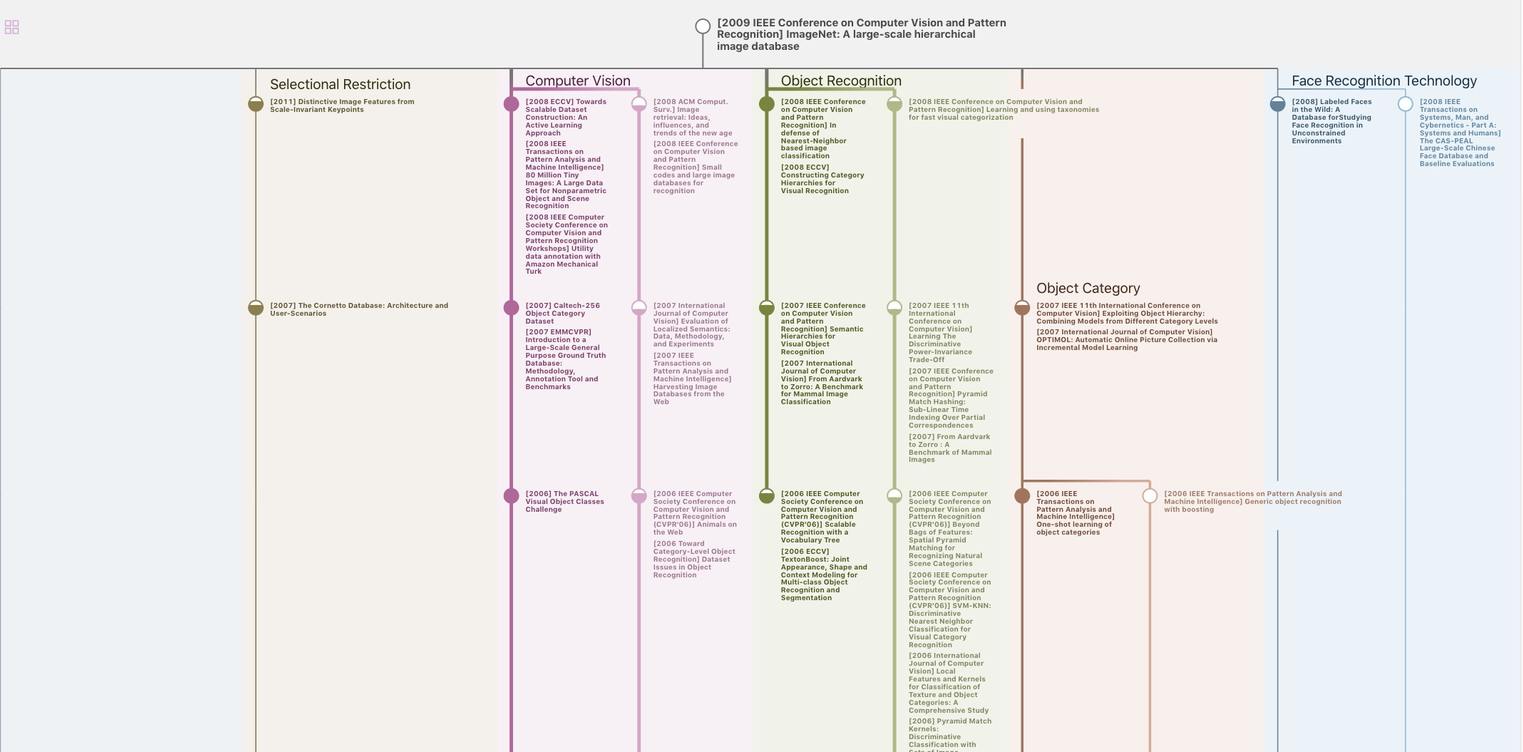
Generate MRT to find the research sequence of this paper
Chat Paper
Summary is being generated by the instructions you defined