A comparative study of hybrid ensembles for forecasting time series applications
REVISTA BRASILEIRA DE COMPUTACAO APLICADA(2021)
Abstract
Time series forecasting assists decision making in several areas such as marketing, economics and industry, being that the main purpose is to estimate the future behavior of a sequence of observations. Thus, sets of hybrid models (ensembles), which combine machine learning and statistical models, have been shown efficient in predicting time series. However, the correct selection of models and combination in an ensemble is important to ensure the system performance. Thus, this work proposes and compares different approaches of hybrid ensembles to improve time series forecasting. A set of forecasting models and different combination strategies are proposed to deal with time series of different patterns. The first ensemble approach combines a set of accurate models using four combination strategies. The second ensemble approach selects automatically models and combination using meta-heuristics. The approaches are compared using a new data set from a cosmetic distribution company and a public data set. The results demonstrate that the proposed ensembles are efficient to reduce the prediction errors.
MoreTranslated text
Key words
Machine Learning,Ensemble,Metaheuristic,Time Series Forecasting
AI Read Science
Must-Reading Tree
Example
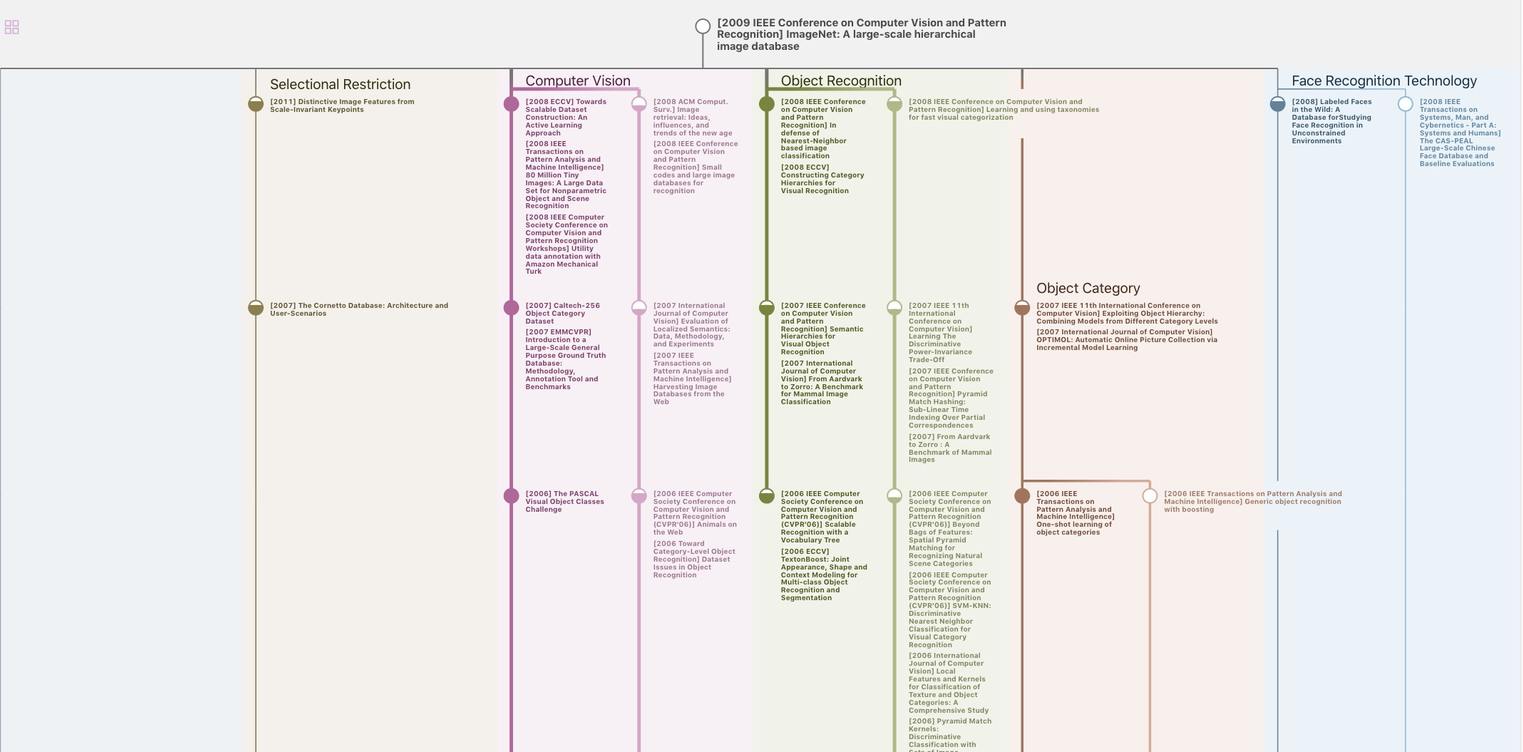
Generate MRT to find the research sequence of this paper
Chat Paper
Summary is being generated by the instructions you defined