Analyzing And Visualizing Deep Neural Networks For Speech Recognition With Saliency-Adjusted Neuron Activation Profiles
ELECTRONICS(2021)
摘要
Deep Learning-based Automatic Speech Recognition (ASR) models are very successful, but hard to interpret. To gain a better understanding of how Artificial Neural Networks (ANNs) accomplish their tasks, several introspection methods have been proposed. However, established introspection techniques are mostly designed for computer vision tasks and rely on the data being visually interpretable, which limits their usefulness for understanding speech recognition models. To overcome this limitation, we developed a novel neuroscience-inspired technique for visualizing and understanding ANNs, called Saliency-Adjusted Neuron Activation Profiles (SNAPs). SNAPs are a flexible framework to analyze and visualize Deep Neural Networks that does not depend on visually interpretable data. In this work, we demonstrate how to utilize SNAPs for understanding fully-convolutional ASR models. This includes visualizing acoustic concepts learned by the model and the comparative analysis of their representations in the model layers.
更多查看译文
关键词
explainable AI, visualization, model introspection, speech recognition, convolutional neural networks
AI 理解论文
溯源树
样例
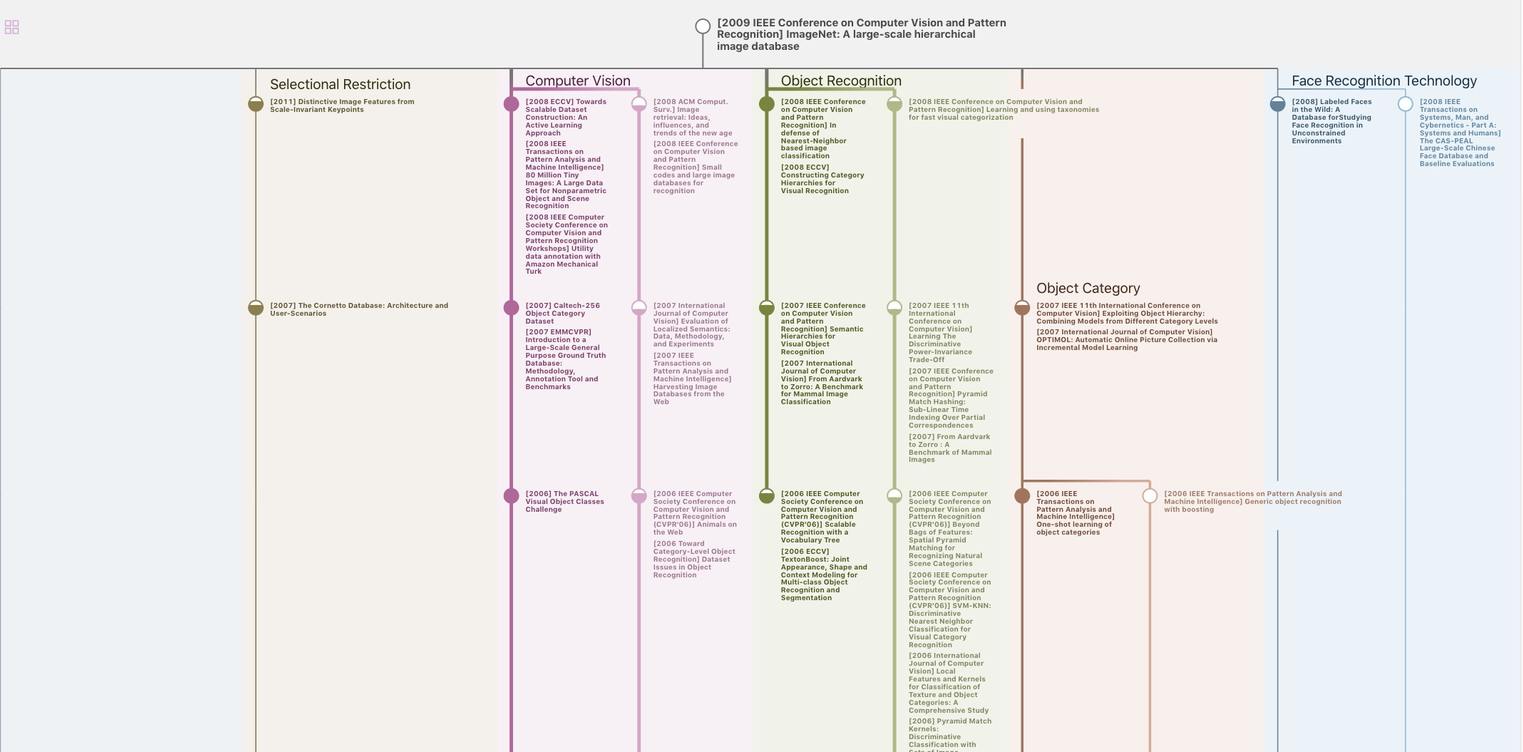
生成溯源树,研究论文发展脉络
Chat Paper
正在生成论文摘要