Motion Correction Of Respiratory-Gated Pet Image Using Deep Learning Based Image Registration Framework
15TH INTERNATIONAL MEETING ON FULLY THREE-DIMENSIONAL IMAGE RECONSTRUCTION IN RADIOLOGY AND NUCLEAR MEDICINE(2019)
摘要
Artifacts caused by patient breathing and movement during PET data acquisition affect image quality. Respiratory gating has been proposed to gate the list-mode PET data into multiple bins over a respiratory cycle. Non-rigid registration of respiratory-gated PET images can reduce the motion artifacts and preserve the count statistics, but it is time consuming. In this work, we propose an unsupervised non-rigid image registration framework using deep learning. We use a differentiable spatial transformer layer to warp the source image to the target image and use a stacked structure for deformation field refinement. Estimated deformation fields were incorporated into an iterative image reconstruction algorithm to perform motion compensated PET image reconstruction. We validated the proposed method using simulation and clinical data and showed its ability to reduce the motion artifact in PET images.
更多查看译文
关键词
deep learning, motion correction, PET, image reconstruction
AI 理解论文
溯源树
样例
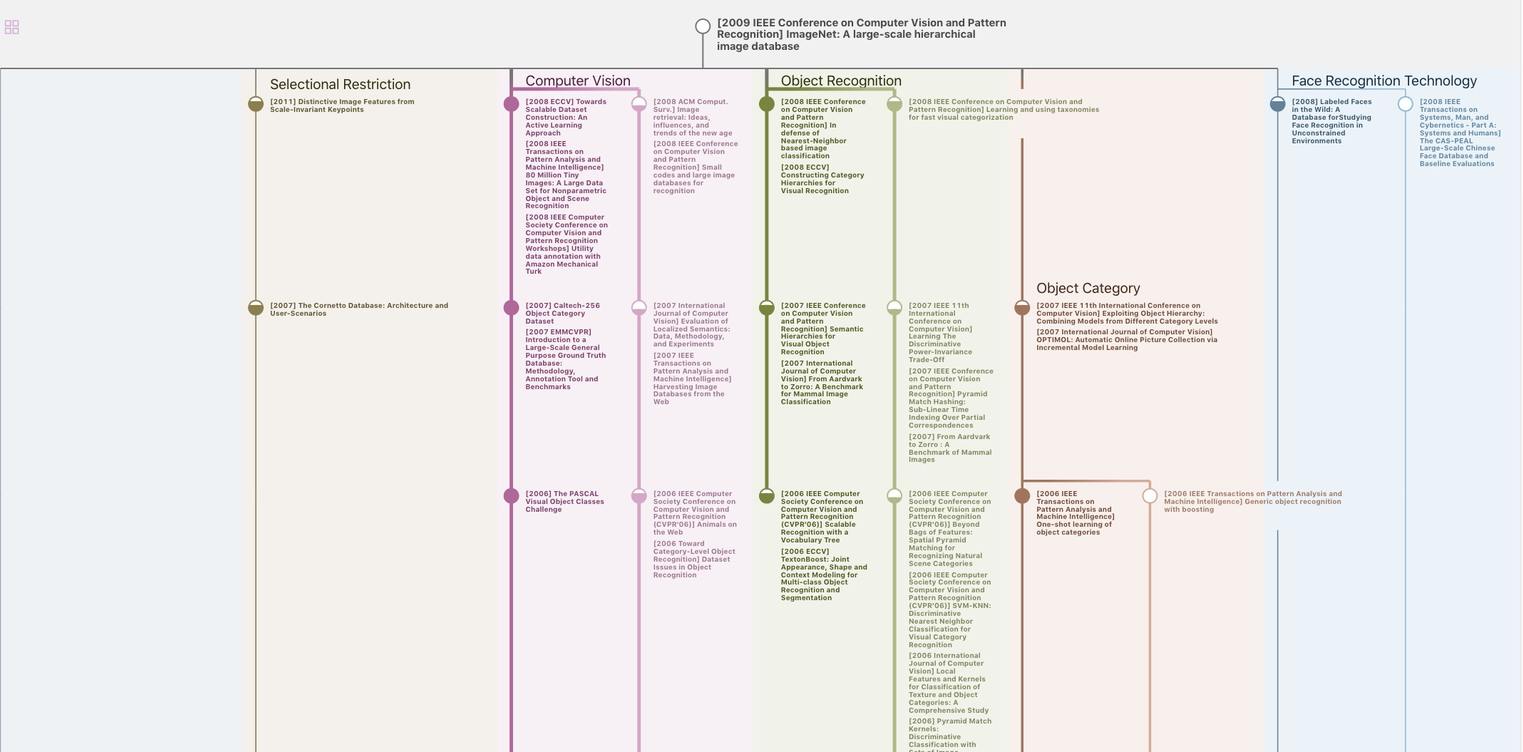
生成溯源树,研究论文发展脉络
Chat Paper
正在生成论文摘要