Dynamic Non-Gaussian hybrid serial modeling for industrial process monitoring
Chemometrics and Intelligent Laboratory Systems(2021)
摘要
Process monitoring has been widely used for fault detection and performance supervision in modern industrial processes. Nevertheless, hybrid characteristics including Gaussianity, non-Gaussianity and dynamic usually coexist in process variables, which brings new challenge to obtain satisfactory monitoring performance. Aiming at the hybrid characteristics problem, this paper proposes a dynamic non-Gaussian hybrid serial modeling method for industrial process monitoring. First, a multivariate non-Gaussianity evaluation method is utilized to divide industrial process variables into the Gaussian variable subspace and the non-Gaussian variable subspace. Afterwards considering the hybrid characteristics including Gaussianity, non-Gaussianity and dynamic at information level, a dynamic principal component analysis (DPCA)-dynamic independent component analysis (DICA)-based hybrid serial modeling method is presented for analyzing simultaneously the dynamic Gaussian and non-Gaussian information in each variable subspace. Subsequently, the final monitoring results are obtained using Bayesian inference and the DPCA-DICA-based hybrid serial similarity factor is proposed for fault identification. Unlike the existing methods, the proposed method analyzes simultaneously the Gaussianity, non-Gaussianity and dynamic at different levels of variable and information for improving performance. The case studies including a numerical system, the Tennessee Eastman process and a practical industrial process demonstrate its feasibility and effectiveness.
更多查看译文
关键词
Process monitoring,Dynamic non-Gaussian hybrid serial modeling,Multivariate non-Gaussianity evaluation,Bayesian inference,Hybrid serial similarity factor
AI 理解论文
溯源树
样例
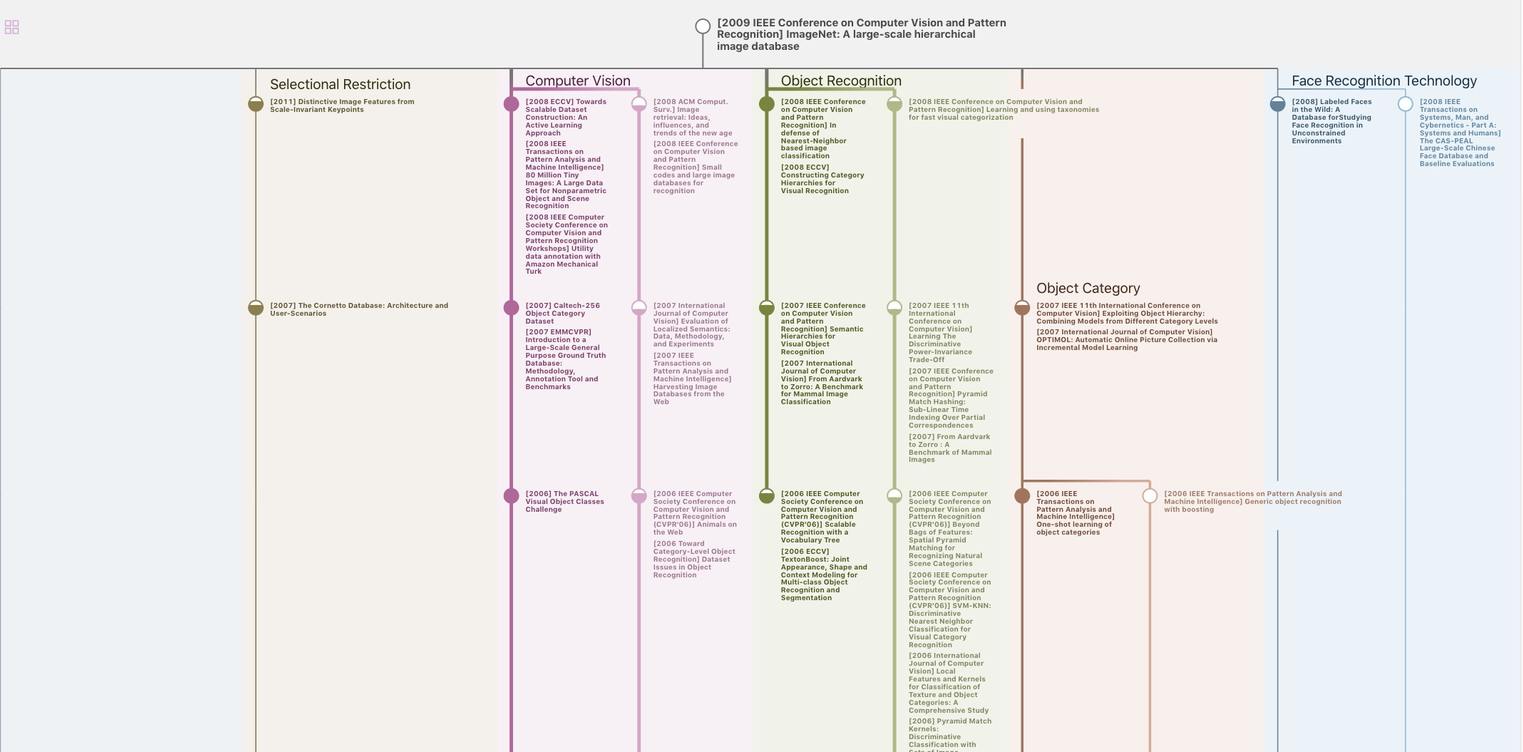
生成溯源树,研究论文发展脉络
Chat Paper
正在生成论文摘要