Impact Of Ai On Image Quality And Suv Quantification On F18-Fdg Pet/Ct
JOURNAL OF NUCLEAR MEDICINE(2020)
摘要
1479 Objectives: The goal of imaging is to get the best possible images in the shortest amount of time while minimizing radiation exposure and maximizing patient comfort. If a balance is not maintained in the radiation exposure and time of acquisition, excessive noise can degrade image quality. The use of Artificial Intelligence (AI) can bridge the gap between reduced counts and image quality by reducing the noise of an image. Subtle Medical is the first and only AI processing for F18-FDG PET/CT imaging with FDA approval called SubtlePET. It claims to enhance images with time and/or radiopharmaceutical dose reduction while maintaining image quality. The purpose of this study is to evaluate and compare PET image quality with the use of AI and assess any differences in the quantification of SUV within a lesion and internal reference point. Methods: The study included 15 random FDG-PET/CT whole body studies. Imaging protocols were set up using the institution’s standard of care for a standard F18-FDG PET/CT scan. Our standard full count protocol is 3 min/bed. We then simulated 50% counts (1.5 min/bed) and 25% counts (0.75 min/bed) before and after AI. Scans were read and analyzed by two board certified nuclear medicine physicians. Image quality was graded on a scale of 1 to 5 (1=poor; 5=excellent). A 3 cm volumetric region of interest was used to measure the SUVmax of the liver in the right hepatic lobe at the level of the adrenal gland and most FDG avid lesion. A log was created to document image quality, BMI, SUVmax of the liver and SUVmax of a lesion in all image sets. Results: Our data shows the image quality of the 50% count reduction + AI was closest to that of the full count study. The mean of the various imaging sets are seen in Table 1. In addition, across all BMIs, the SUVmax values of the liver and lesion in the 50% count reduction + AI was not statistically significant to the full count study whereas all others were statistically significant (Tables 2 & 3). Conclusions: The 50% reduced count study with AI most closely resembles the full count study with regards to image quality and SUV quantification. However, the use of AI with only 25% counts cannot adequately compensate for the image degradation. Further studies are being done evaluating the use of AI with PET radiotracers other than FDG.
更多查看译文
关键词
suv quantification,image quality,pet/ct
AI 理解论文
溯源树
样例
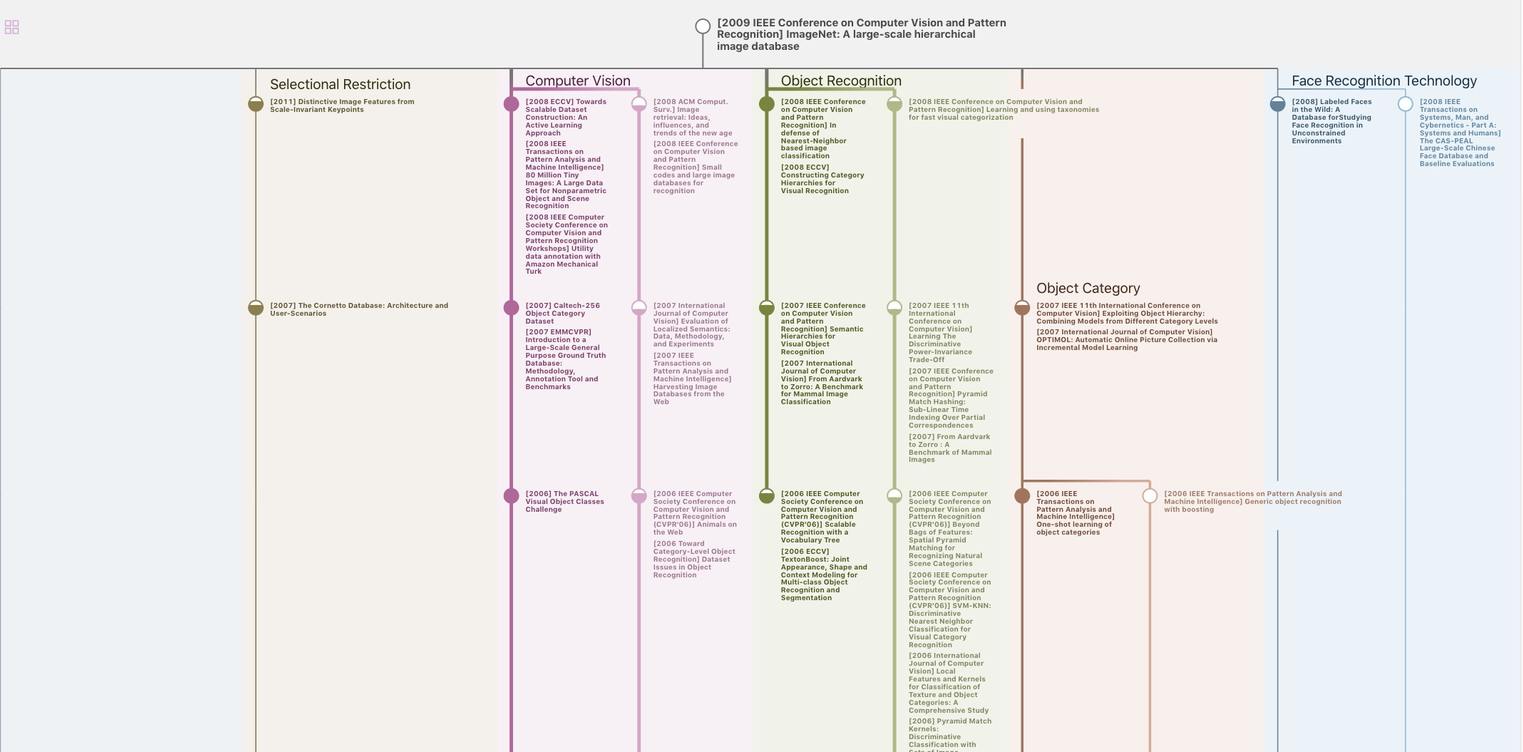
生成溯源树,研究论文发展脉络
Chat Paper
正在生成论文摘要