Estimation of intersection traffic density on decentralized architectures with deep networks
2017 International Smart Cities Conference (ISC2)(2017)
摘要
Intelligent Intersection Traffic Management has become increasingly important because of the need to reduce congestion and improve the overall travel experience of commuters. Given the dynamic nature of everyday city traffic, this paper proposes real-time processing of videos from cameras to estimate the traffic density and optimize the signal parameters of the intersection. The region-of-interest of the road segment is automatically extracted as a one-time operation, and the density is estimated using an ensemble of background subtraction and variants of convolutional neural networks. The architecture is scalable as the processing is entirely decentralized on the edge computing device installed at every intersection. We demonstrate that our technique is resilient to low resolution videos, long fields of view and chaotic traffic conditions with high occlusion. The proposed algorithms generalize across deployments with minimal reconfiguration.
更多查看译文
关键词
Intelligent Traffic Systems,Deep Learning,Convolutional Neural Networks,Edge Computing,Background Subtraction,Faster RCNN
AI 理解论文
溯源树
样例
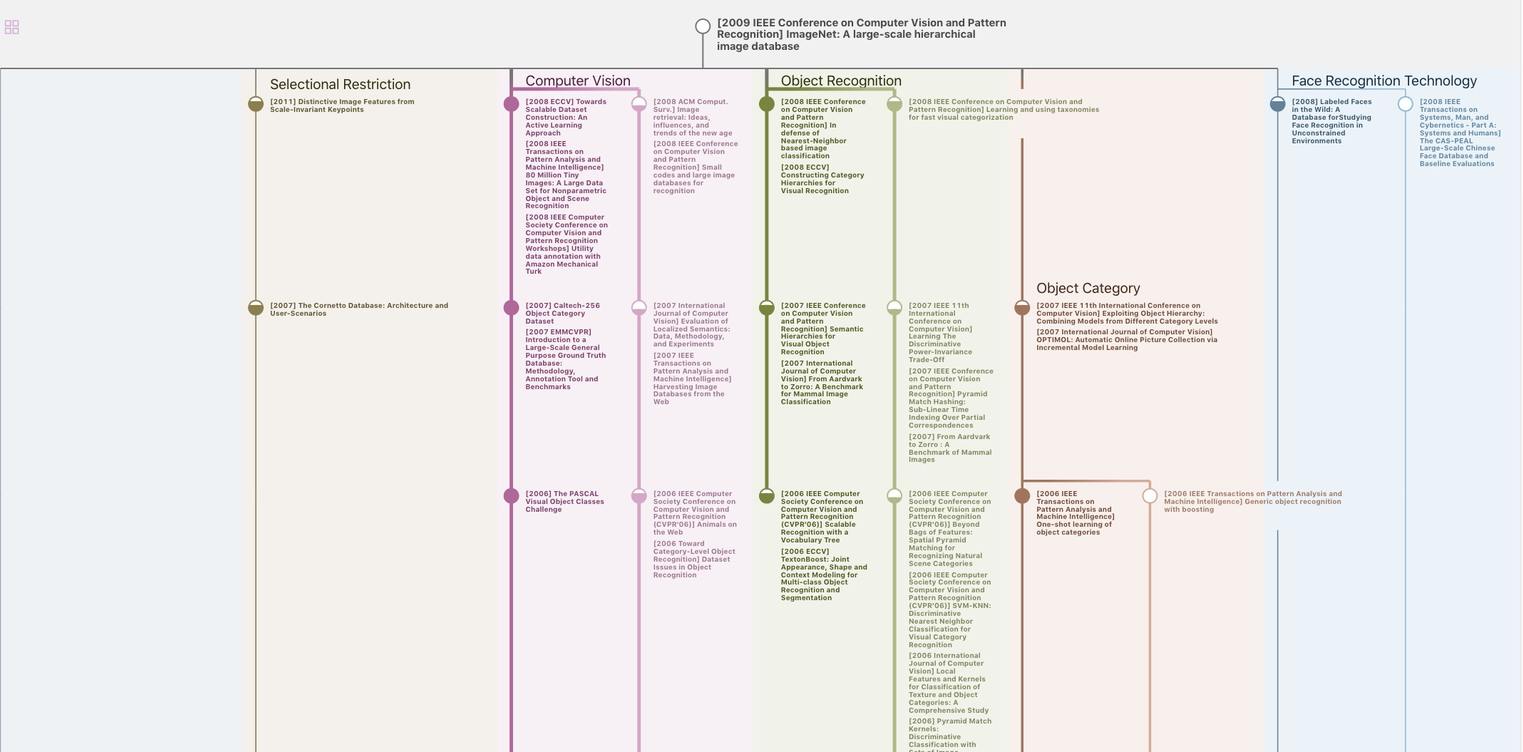
生成溯源树,研究论文发展脉络
Chat Paper
正在生成论文摘要