Experimental And Theoretical Study On The Robustification Of Acoustic Emission Inspection With Recurrent Neural Networks
MECHANICS OF BIOLOGICAL SYSTEMS & MICRO-AND NANOMECHANICS, VOL 4(2019)
摘要
This paper presents a summary of an experimental and theoretical study conducted to improve the performance of Acoustic Emission (AE) based structural health monitoring. Two broadband AE sensors are attached to a test specimen and a series of artificial test signals were generated using multiple pencil-lead breaks. The geometry of the test specimen is such that; one of the AE sensors always receives a direct wave and the second sensor receives reflection (or echo) of the same direct wave received at the first sensor. Time series data of the direct and reflected waves, recorded at a rate of 5 MHz, are used to train a multi-layer recurrent neural network (RNN) in a supervised setting. The inherent patterns learned from the training data are used to classify received acoustic emission waveforms into reflection and direct waves. Preliminary results show that the proposed technique, at least in a similar setting and material, improves the performance of AE based health monitoring.
更多查看译文
关键词
Acoustic emission, Recurrent neural network, LSTM, Classification, Sequence
AI 理解论文
溯源树
样例
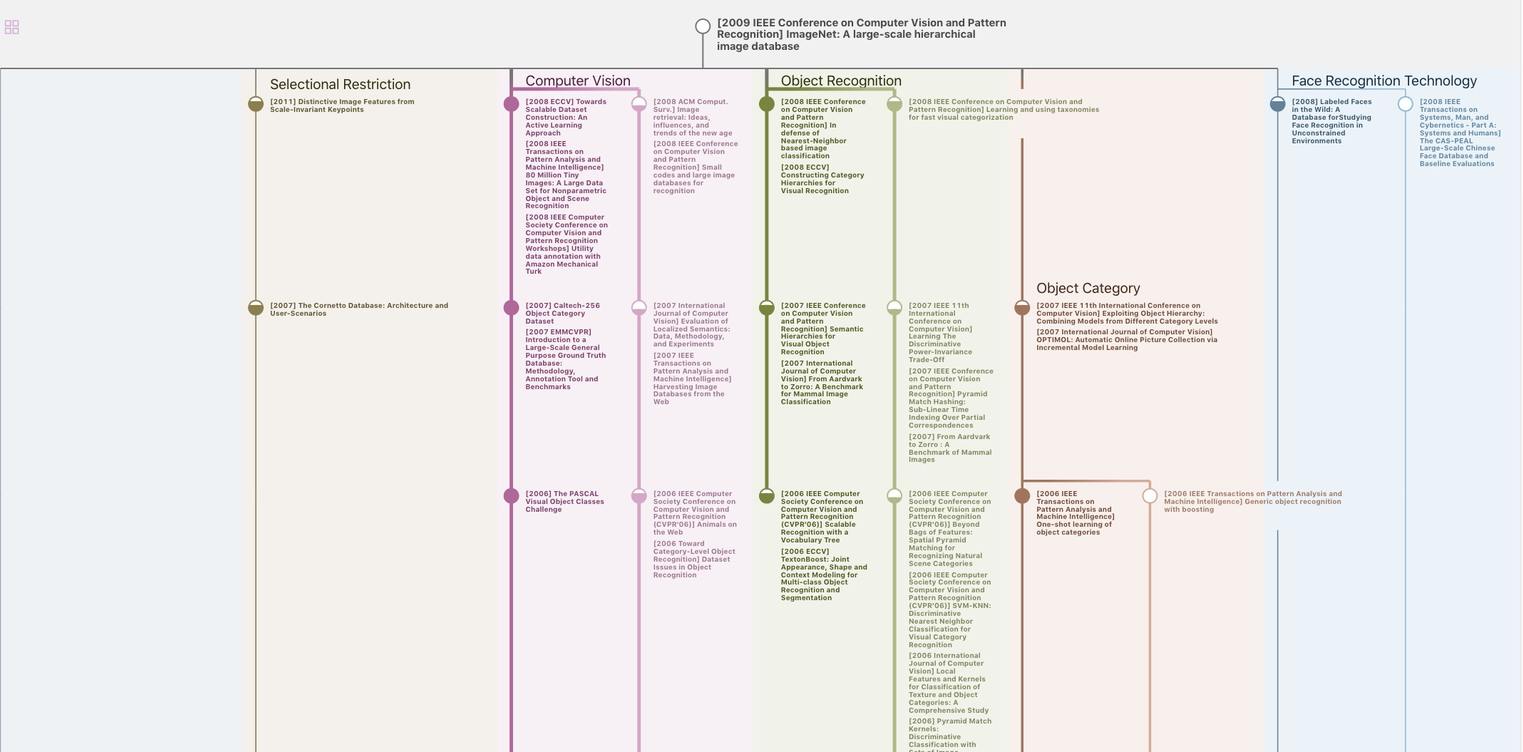
生成溯源树,研究论文发展脉络
Chat Paper
正在生成论文摘要