Emerging Insights From Eye-Movement Research On Category Learning
COGNITION IN FLUX(2010)
摘要
Emerging Insights from Eye-Movement Research on Category Learning Bob Rehder (bob.rehder@nyu.edu) Department of Psychology, New York University 6 Washington Place, New York, NY 10003 Mark R. Blair (mark_blair@sfu.ca) Cognitive Science Program & Department of Psychology Simon Fraser University, 8888 University Drive, Burnaby, BC, V5A 1S6, CANADA Aaron B. Hoffman (aaron.hoffman@mail.utexas.edu) Department of Psychology, University of Texas 1 University Station A8000, Austin, Texas 78712-0187 Marcus R. Watson (marcusw@psych.ubc.ca) Department of Psychology, University of British Columbia 2136 West Mall, Vancouver, B.C., V6T 1Z4, Canada Keywords: eye-tracking, category learning; categorization; attention; learning; optimization, Parkinson’s disease, inference learning, error processing, working memory. Introduction Attempts to develop an accurate measure of eye movements are over a century old (e.g., Delabarre, 1898; Huey, 1898; as cited in Karatekin, 2007), and predate the earliest studies of categorization (Hull, 1920). Given the long history of both categorization and eye-tracking, it is surprising that eye- tracking has only recently been added to the categorization researcher’s toolbox (Rehder & Hoffman, 2005a). Selective attention is an important component of theories of categorization and eye-tracking provides a measure of what features of a stimulus participants have selected to attend. There are alternatives to eye-tracking, such as inferring attention allocation based on model fits or carefully designed transfer tasks. However, these methods lack the directness of eye-tracking and provide only a coarse measure of how attention shifts over the course of learning. Moreover, they provide no account of how attention is allocated early in learning and within a single categorization trial (including after feedback is presented). This more fine- grained data can not only clarify our understanding of key phenomena, it broadens the range of experimental questions that can be asked to understand how humans learn categories (Blair, Watson & Meier, 2009; Blair, Watson, Walshe & Maj, 2009; Hoffman & Rehder; 2009; Kim & Rehder, 2009; Rehder, Colner & Hoffman, 2009; Rehder & Hoffman, 2005a; Rehder & Hoffman, 2005b; Watson & Blair, 2008) This symposium brings together four talks on eye- tracking and categorization. Each talk focuses on a different aspect of categorization and demonstrates how using eye- tracking can extend our knowledge. One recent trend in category learning is the use of alternative training procedures. The inference learning task is the most popular of these procedures and in the first talk Aaron Hoffman presents eye-tracking data illuminating the differences between inference learning and categorization. Bob Rehder then presents his recent work on understanding the learning difficulties associated with Parkinson’s disease. Marcus Watson discusses work using eye-tracking to inform our understanding of the basic issue in category learning: error. Finally, Mark Blair discusses the relationship between working memory, attention and performance in a category learning tasks. Inference versus classification learning It has been proposed that whereas feature inference learning promotes learning a category’s internal structure (e.g., typical features and feature correlations), classification promotes the learning of diagnostic information (Markman & Ross, 2003). We tracked learners’ eye movements and found that inference learners fixated features that were unnecessary for inferring a missing feature—consistent with their acquiring the categories’ internal structure. However, those fixations were limited to features that needed to be predicted on future trials. Inference learning appeared to induce both supervised and unsupervised learning of category-to-feature associations, rather than any general motivation to learn the internal structure of categories. In a second study, we compared how inference and classification learning support learners’ ability to draw novel contrasts—category distinctions that were not part of training. We found that classification learners were at a disadvantage at making novel contrasts. Eye movement data indicated that this conceptual inflexibility was due to (a) a narrow attention profile that fails to encode many category features and (b) learned inattention that inhibits the reallocation of attention to newly relevant information. Implications of these costs of supervised classification learning for views of conceptual structure will be discussed.
更多查看译文
关键词
eye-tracking, category learning, categorization, attention, learning, optimization, Parkinson's disease, inference learning, error processing, working memory
AI 理解论文
溯源树
样例
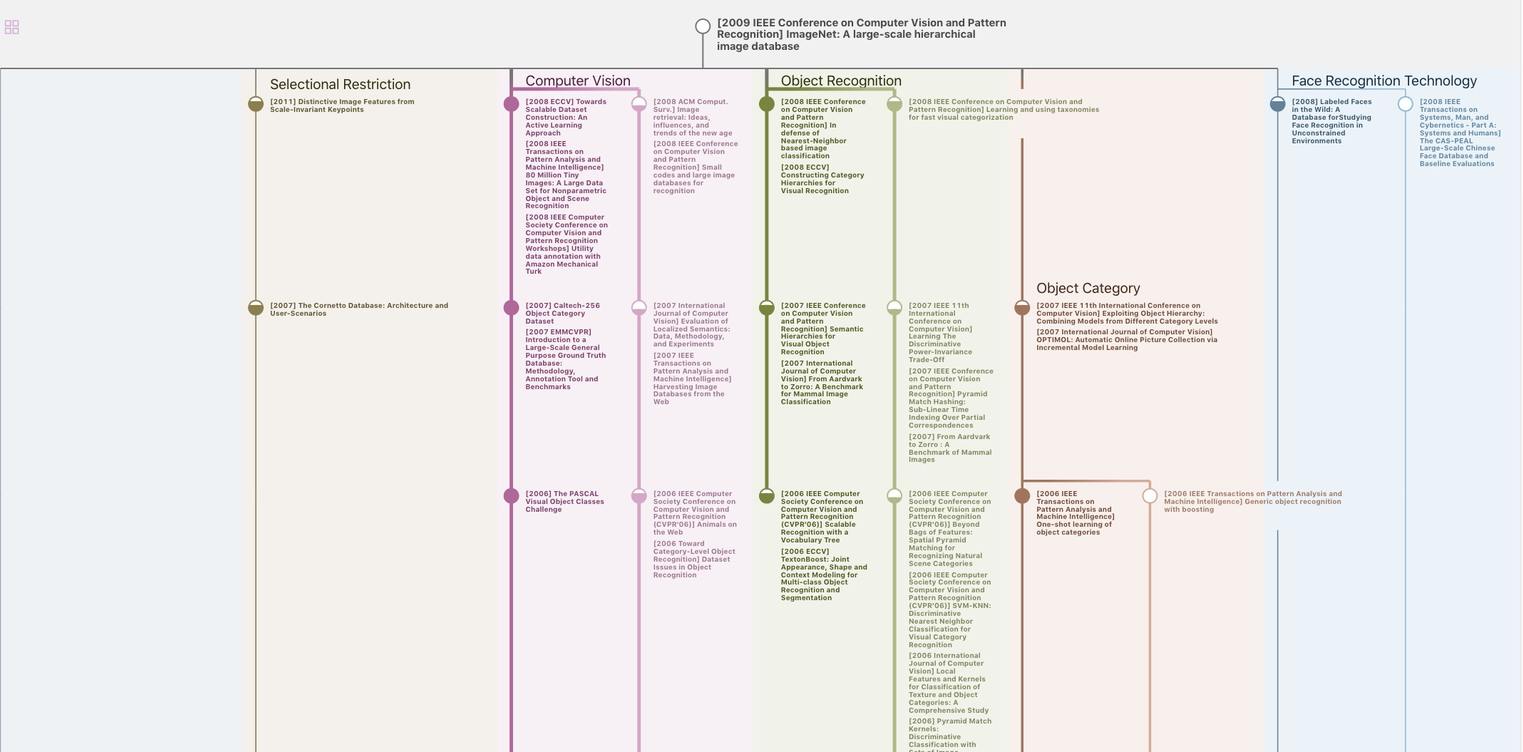
生成溯源树,研究论文发展脉络
Chat Paper
正在生成论文摘要