Identifying Severity Level of Cybersickness from EEG signals using CN2 Rule Induction Algorithm
2018 International Conference on Intelligent Informatics and Biomedical Sciences (ICIIBMS)(2018)
摘要
One of the typical gaming disorder is cybersickness. Cybersickness is the condition that occurs during or after exposed by the virtual environment. The increasing of cybersickness symptoms in gamers can lead to the poor health condition. Prior studies in investigating cybersickness employ subjective self-reports questionnaire, i.e., simulator sickness questionnaire (SSQ). However, the objective measurement is required to determine the actual condition of subjects due to cybersickness severity level. Therefore, this paper proposed identification of cybersickness severity level using electroencephalograph (EEG) signals. From the EEG, we extract the best feature such as percentage change (PC) of power percentage (PP) in beta and theta frequency band from pre- to post-stimulation. We found a specific pattern of cybersickness that marked by the sudden decreasing of PPβ during the recording between baseline segment (4 minutes) and the last part (4 minutes) of game playing. Unlike previous studies, this paper proposed the rules-based algorithm i.e. CN2 Rules Induction for identifying cybersickness severity level. This giving ease for medical-expert to determine appropriate diagnosis and treatment towards patients. The classification yields the best accuracy of 88.9% using the CN2 rule induction. It is outperforming other classifiers accuracies such as decision tree (72.2%) and SVM (83.3 %). According to the results, incorporating PC of the PPβ feature with the rules-based algorithm is working well for identifying cybersickness severity level from EEG.
更多查看译文
关键词
brain signals,motion sickness,power percentage of beta,CN2 rule algorithm
AI 理解论文
溯源树
样例
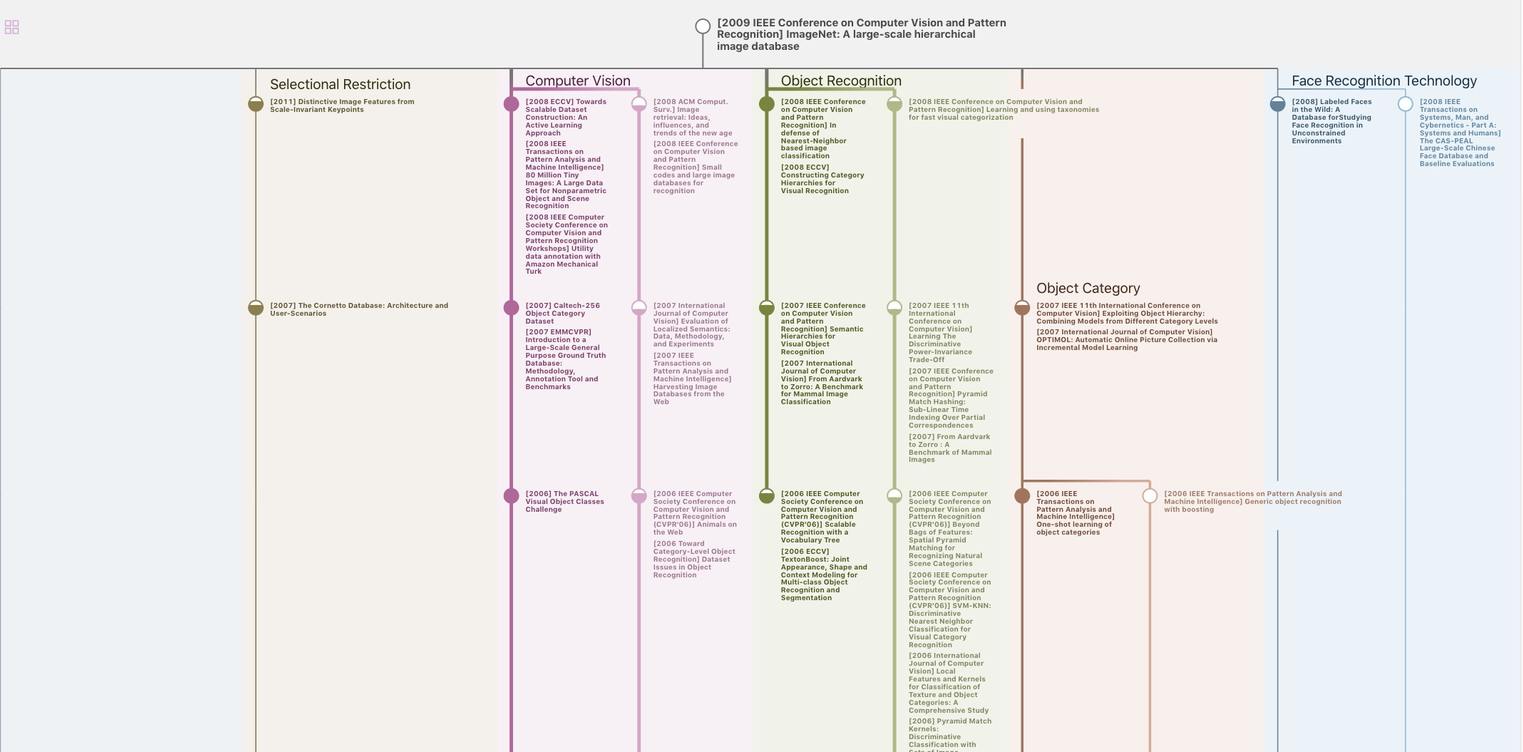
生成溯源树,研究论文发展脉络
Chat Paper
正在生成论文摘要