A Distributed Algorithm For Nonconvex Quadratically Constrained Programs
IFAC PAPERSONLINE(2020)
摘要
This paper considers nonconvex quadratically constrained programs over undirected connected graphs. We focus on problems whose quadratic constraint forms have sparse hessians, i.e., each constraint only involves a small subset of variables. We present both centralized and distributed treatment of the problem, where the centralized method is used as a benchmark with which we compare the performance of the proposed distributed algorithm. Towards this objective, we derive a lower bound on the optimal value of the problem using a semidefinite relaxation. Then, we develop a decentralized algorithm based on proximal gradient ADMM that exploits the structure of the constraints to distribute the computations among the graph nodes. Indeed, the proposed algorithm does away with a central node. Each node updates its local variables via solving a simple subproblem, then communicate with its immediate neighbors. An application of this work is the AC optimal power flow problem in power networks for which we provide preliminary numerical results to validate our findings. Copyright (C) 2020 The Authors.
更多查看译文
关键词
Distributed optimization, convex relaxation
AI 理解论文
溯源树
样例
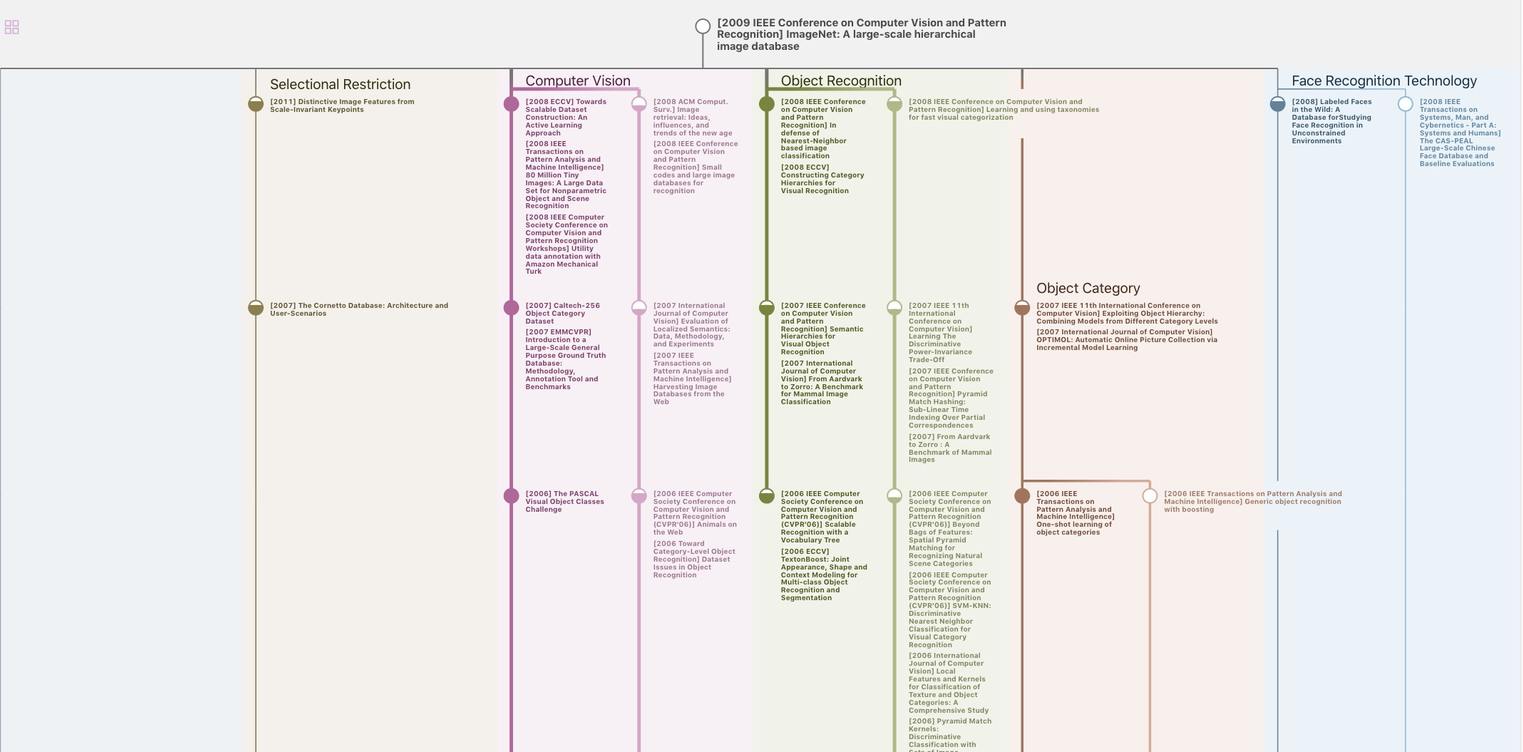
生成溯源树,研究论文发展脉络
Chat Paper
正在生成论文摘要