Reconstruction Of Cross-Correlations Between Heterogeneous Trackers Using Deterministic Samples
IFAC PAPERSONLINE(2020)
摘要
The exploitation of dependencies between state estimates from distributed trackers plays a vital role in so-called track-to-track fusion and has been extensively studied for state estimates with the same state space. In contrast, dependencies are often neglected when considering heterogeneous state estimates referring to different state spaces, since the necessary transformations make the analytic calculation complex or infeasible. This paper aims to develop an overarching framework for the reconstruction of cross-covariances between state estimates obtained in heterogeneous state spaces. The proposed method uses a set of deterministic samples to calculate dependent information. Thus, it allows for a distributed track-keeping of correlations that also encodes the transformation into the local subsystems. To highlight the algorithm, we use a linear problem with heterogeneous trackers only and discuss the correlation problem in detail. The results show superior performance compared to neglecting the correlations. Copyright (C) 2020 The Authors.
更多查看译文
关键词
Distributed Estii nation, Heterogeneous Track-to-Track Fusion, Cross-covariance Reconstruction, Deterministic Samples
AI 理解论文
溯源树
样例
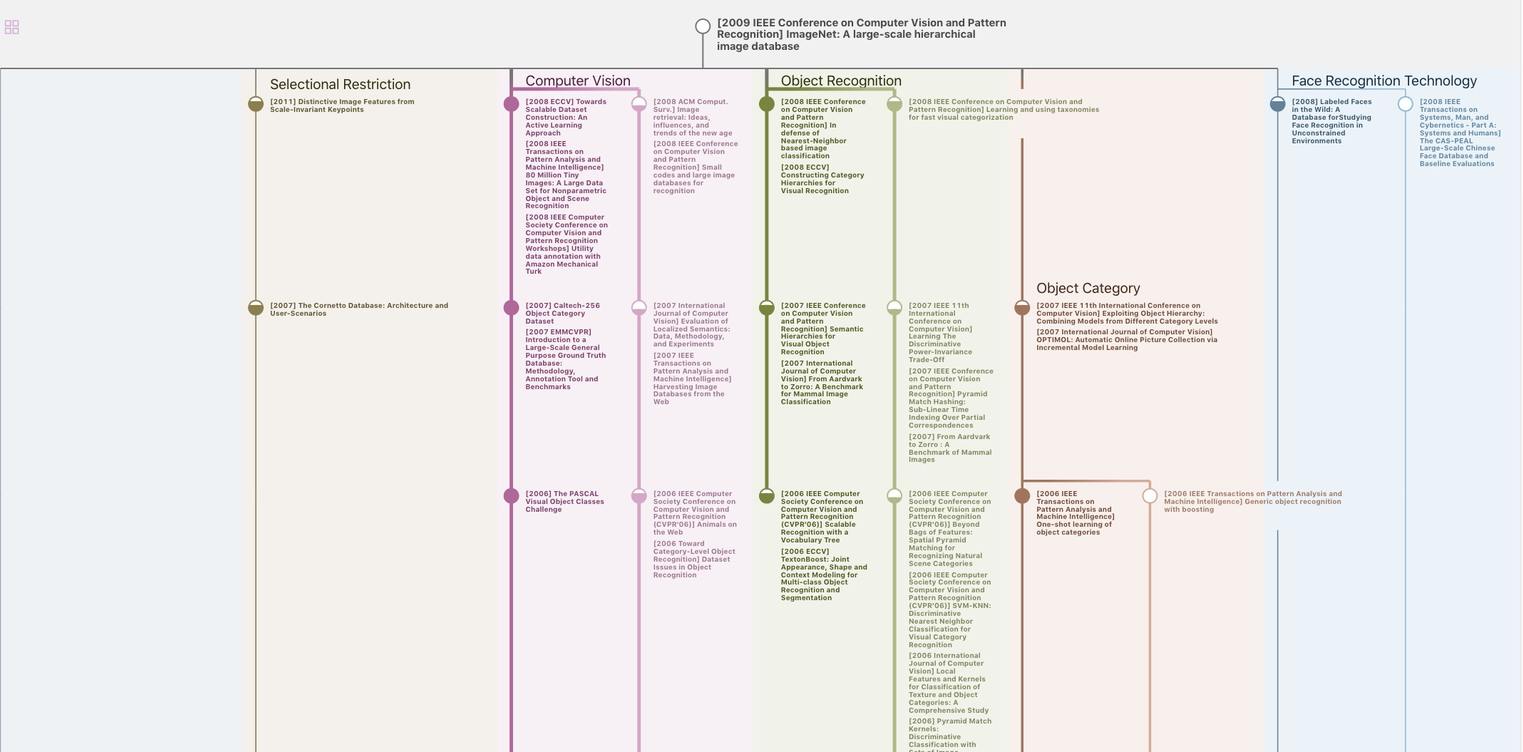
生成溯源树,研究论文发展脉络
Chat Paper
正在生成论文摘要