Maneuverability Hazard Detection And Localization In Low-Altitude Uas Imagery
ARTIFICIAL INTELLIGENCE AND MACHINE LEARNING FOR MULTI-DOMAIN OPERATIONS APPLICATIONS II(2020)
摘要
Object detection and localization is an important problem in computer vision and remote sensing. While there have been several techniques presented and used in recent years, the You Only Look Once (YOLO) and derivative architectures have gained popularity due to their ability to perform real-time object localization as well as achieve remarkable detection scores in ground-based applications. Here, we present methods and results for performing maneuverability hazard detection and localization in low-altitude unmanned aerial systems (UAS) imagery. Imagery is captured over a variety of flight routes and altitudes, and then analyzed with modern deep learning techniques to discover objects such as civilian and military vehicles, barriers, and related hindrances to navigating cluttered semi-urban environments. We present our findings for the deep learning architectures under a variety of training and validation parameters that include pre-trained weights from benchmark public datasets, as well as training with a custom, mission-relevant dataset provided by U.S. Army ERDC.
更多查看译文
关键词
deep learning, object detection, unmanned aerial systems (UAS)
AI 理解论文
溯源树
样例
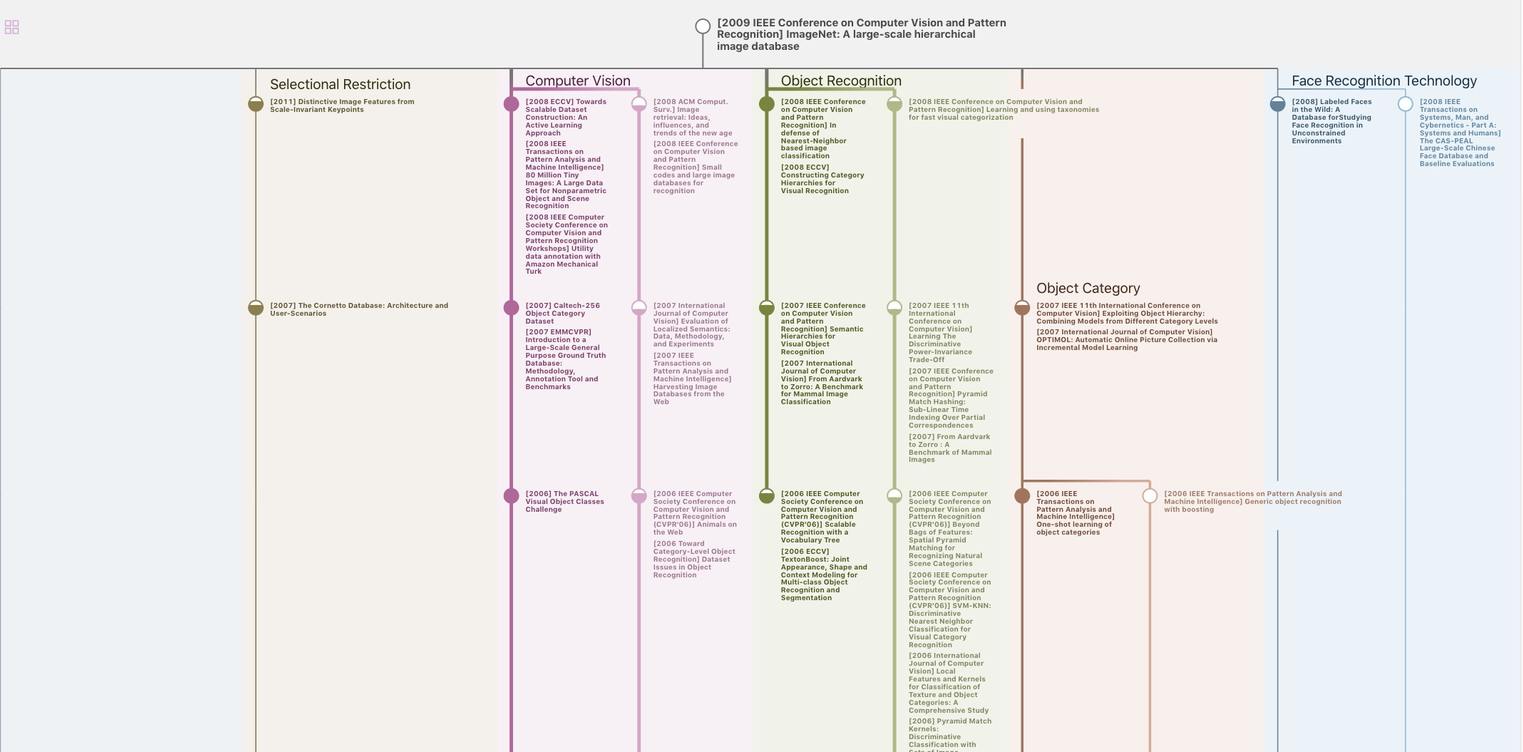
生成溯源树,研究论文发展脉络
Chat Paper
正在生成论文摘要