Target-Sensitive Memory Networks For Aspect Sentiment Classification
PROCEEDINGS OF THE 56TH ANNUAL MEETING OF THE ASSOCIATION FOR COMPUTATIONAL LINGUISTICS (ACL), VOL 1(2018)
摘要
Aspect sentiment classification (ASC) is a fundamental task in sentiment analysis. Given an aspect/target and a sentence, the task classifies the sentiment polarity expressed on the target in the sentence. Memory networks (MNs) have been used for this task recently and have achieved state-of-the-art results. In MNs, attention mechanism plays a crucial role in detecting the sentiment context for the given target. However, we found an important problem with the current MNs in performing the ASC task. Simply improving the attention mechanism will not solve it. The problem is referred to as target-sensitive sentiment, which means that the sentiment polarity of the (detected) context is dependent on the given target and it cannot be inferred from the context alone. To tackle this problem, we propose the targetsensitive memory networks (TMNs). Several alternative techniques are designed for the implementation of TMNs and their effectiveness is experimentally evaluated.
更多查看译文
AI 理解论文
溯源树
样例
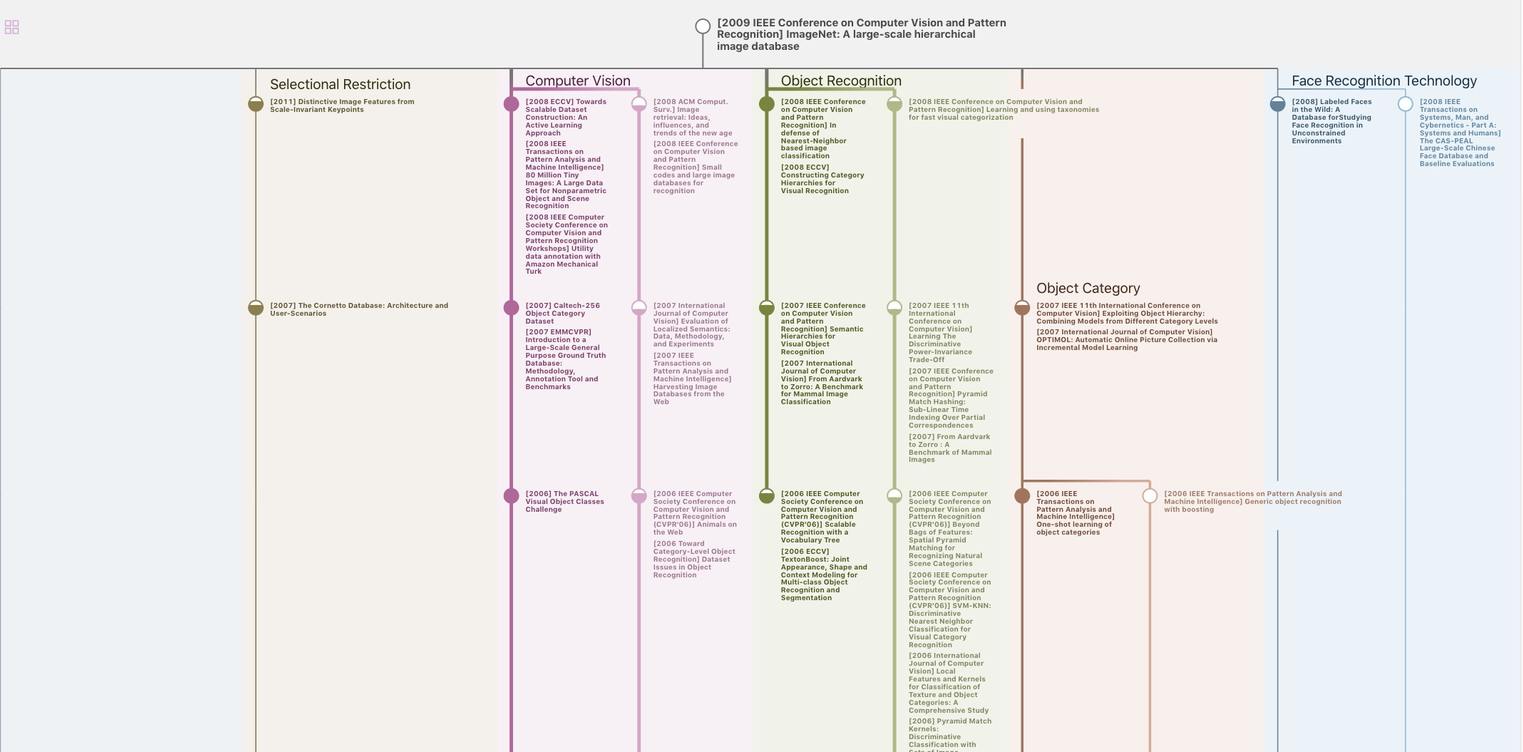
生成溯源树,研究论文发展脉络
Chat Paper
正在生成论文摘要