Application of ARIMA and Markov Combination Model in Medium and Long Term Electricity Forecasting
2019 IEEE 3rd International Electrical and Energy Conference (CIEEC)(2019)
Abstract
The quarterly electricity consumption curve shows certain regularity due to factors such as temperature and holidays. The ARMA(Autoregressive Moving Average) model has a good fitting ability for such time series, but the quarterly electricity consumption is also affected by economic growth. It is an ascending time series, which is a nonstationary time series. It cannot be directly predicted by ARMA model. Therefore, it is necessary to make finite difference processing to the original time series to stationary time series. The original time series is suitable for ARIMA(Autoregressive Integrated Moving Average) model. Any prediction model can not avoid the generation of residuals. The K-means clustering algorithm is used to divide the residual sequence state. By exploring the relationship between states at different moments, the transition matrix is established to predict the probability distribution of the residual state at the next moment. The predicted state is restored to the residual prediction value, and the prediction of the residual is completed to implement the correction of the original prediction value. That is, by predicting the residual by establishing a Markov model, the predicted value of the ARIMA model is corrected, and the prediction accuracy is improved.
MoreTranslated text
Key words
electricity forecast,residual correction,Markov,ARIMA,K-means
AI Read Science
Must-Reading Tree
Example
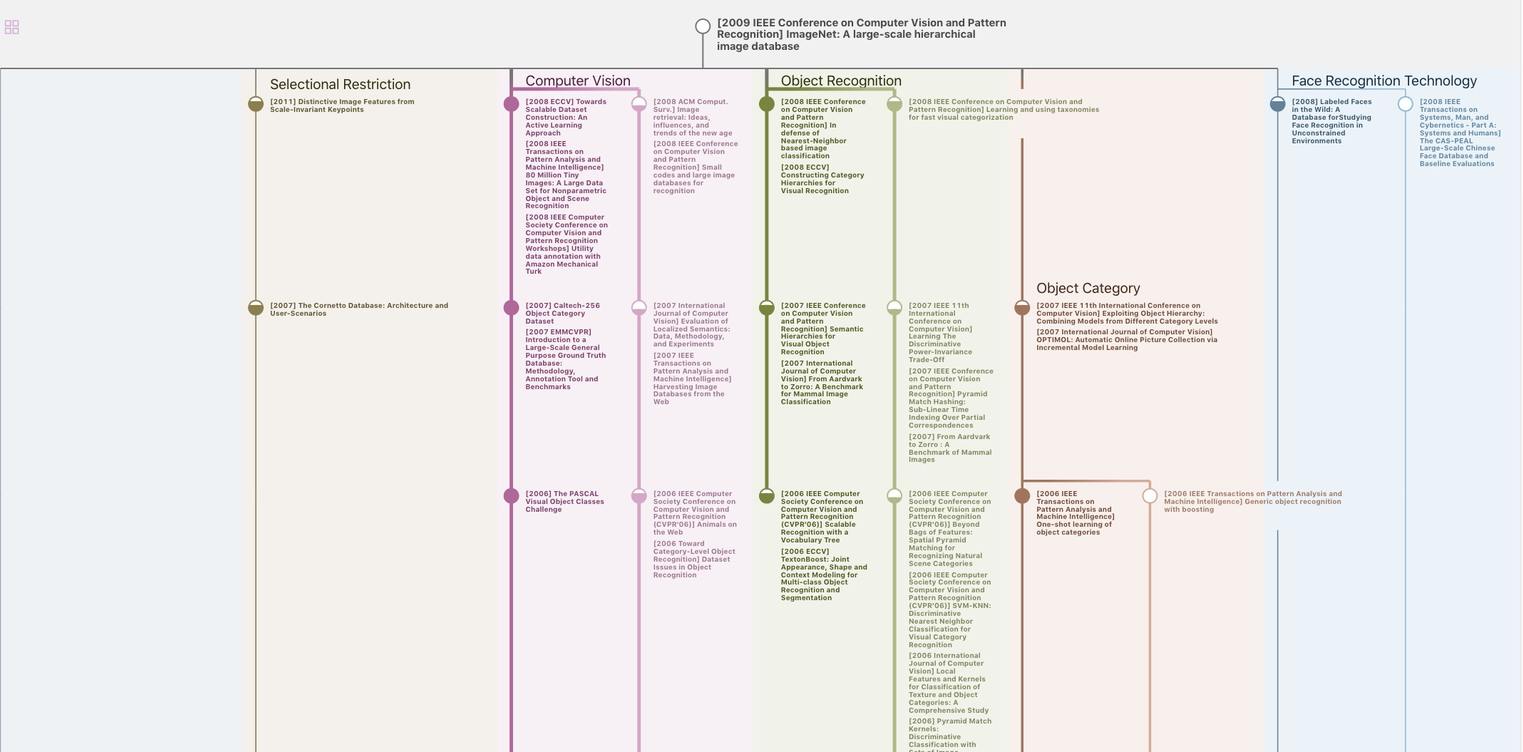
Generate MRT to find the research sequence of this paper
Chat Paper
Summary is being generated by the instructions you defined