An Adaptation Module With Dynamic Radial Basis Function Neural Network Using Significance Concept For Writer Adaptation
JOURNAL OF INFORMATION ASSURANCE AND SECURITY(2017)
摘要
In this paper, we focused on the handwriting-based biometric to personalize the hand held-devices, mainly used by one person, to recognize effectively its new writing style. For this end, we plug-in an adaptation module (AM) with a writer-independent recognition system (WIRS) to generate a writer-dependent recognition system. The WIRS response is then adapted considering the new writing style. The AM applied a sequential learning algorithm named GARBF-AM using a significance concept for writer adaptation based on a Radial Basis Function (RBF) neural network. The proposed GARBFAM algorithm defines a new Growing and Adjustment algorithm named GARBF-AM. This algorithm can dynamically insert new hidden neurons under predefined conditions on the significance of both the new input and the nearest neuron. Otherwise, our algorithm adjusts the nearest and the desired contributor neurons parameters. For experiments, two writer dependent datasets are used. The first is LaViola dataset and the second is MEnv-REGIM that is created considering different physical positions of the writer (sitting, standing, walking, going up/down stairs and by car). The experimental results based on the two datasets show that the performance of the generic WIRS has improved significantly when integrating GARBF-AM algorithm. The comparative study highlights the benefits of the using the GARBF-AM against the well known OAM algorithm.
更多查看译文
关键词
handwriting-based biometric, Incremental learning RBFNN, Writer adaptation, Information Security, Information assurance
AI 理解论文
溯源树
样例
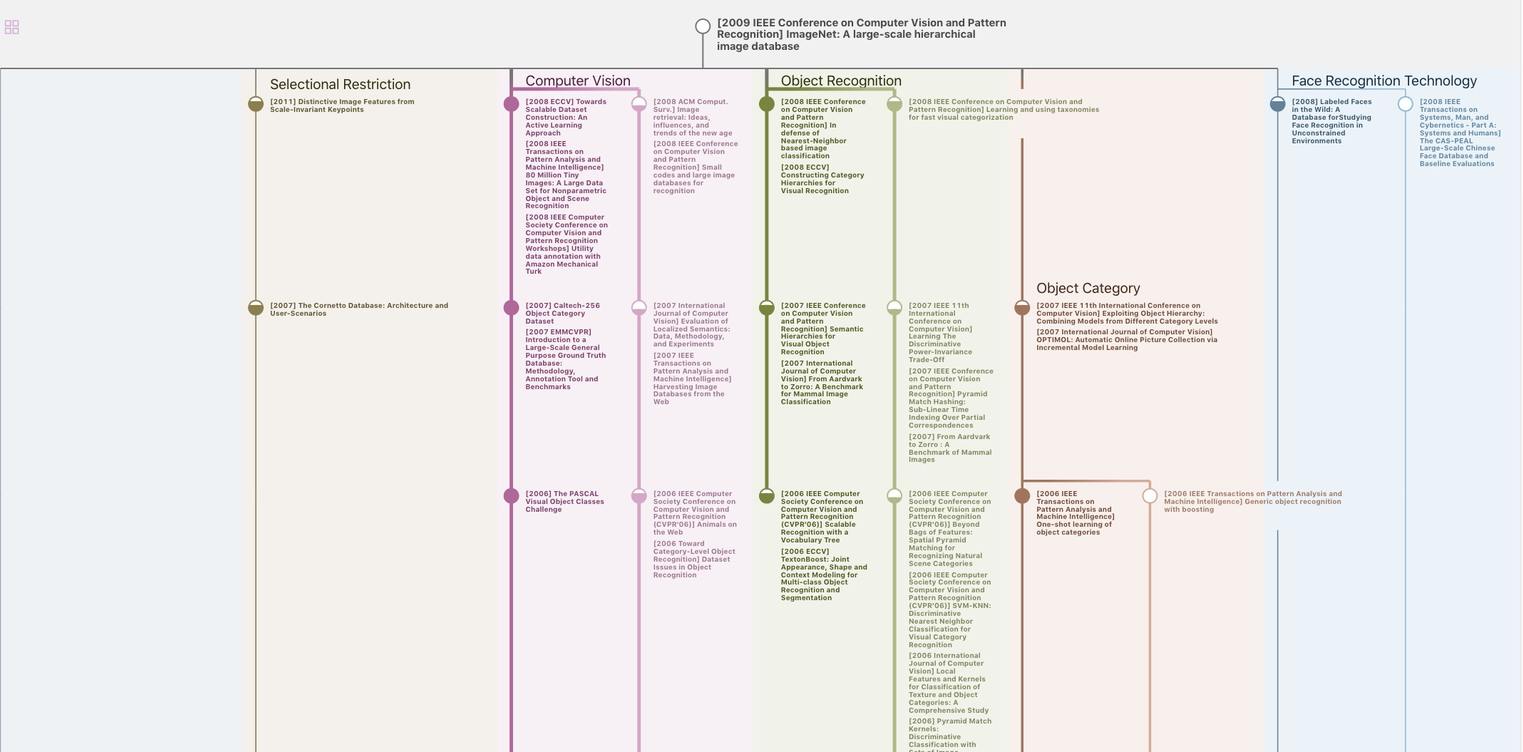
生成溯源树,研究论文发展脉络
Chat Paper
正在生成论文摘要