Robust Target Tracking Using Adaptive Color Feature And Likelihood Fusion
GEOSPATIAL INFORMATICS, MOTION IMAGERY, AND NETWORK ANALYTICS VIII(2018)
摘要
Designing a robust and accurate object tracker is important in many computer vision applications. The problem becomes more complicated when additional factors like changing appearance, illumination, and scale are introduced in the sequence. Recently, trackers that are based on the correlation filter method like Sum of Template and Pixel-wise Learners (STAPLE )(1) have shown state-of-the-art short-term tracking performance. STAPLE consists two major modules: learning correlation filter on HOG features and representing color information using RGB histogram. In this paper, we propose an improved STAPLE (iSTAPLE) tracker by adding the Color Names (CN)(2) to the correlation part of the tracker. CN complements HOG feature because using only HOG can lead to tracking failures in some cases where occlusion or deformation is present. As the color information could be a confusing factor and unreliable in tracking due to the rapid illumination changes, Bhattacharyya distance is used to measure the color similarity between the target and surrounding area to decide whether the color information is helpful. Since we use multiple feature cues to improve tracking performance, a robust approach to fuse multiple features is required. To fully utilize all features and optimize the tracking result, numerous weight combinations assigned to each feature are tested. We show through comprehensive experiments on the VOT Challenge 2016 dataset(3) that iSTAPLE obtains a gain of 25% in tracking robustness.
更多查看译文
关键词
visual object tracking, STAPLE, correlation filter, color name, fusion
AI 理解论文
溯源树
样例
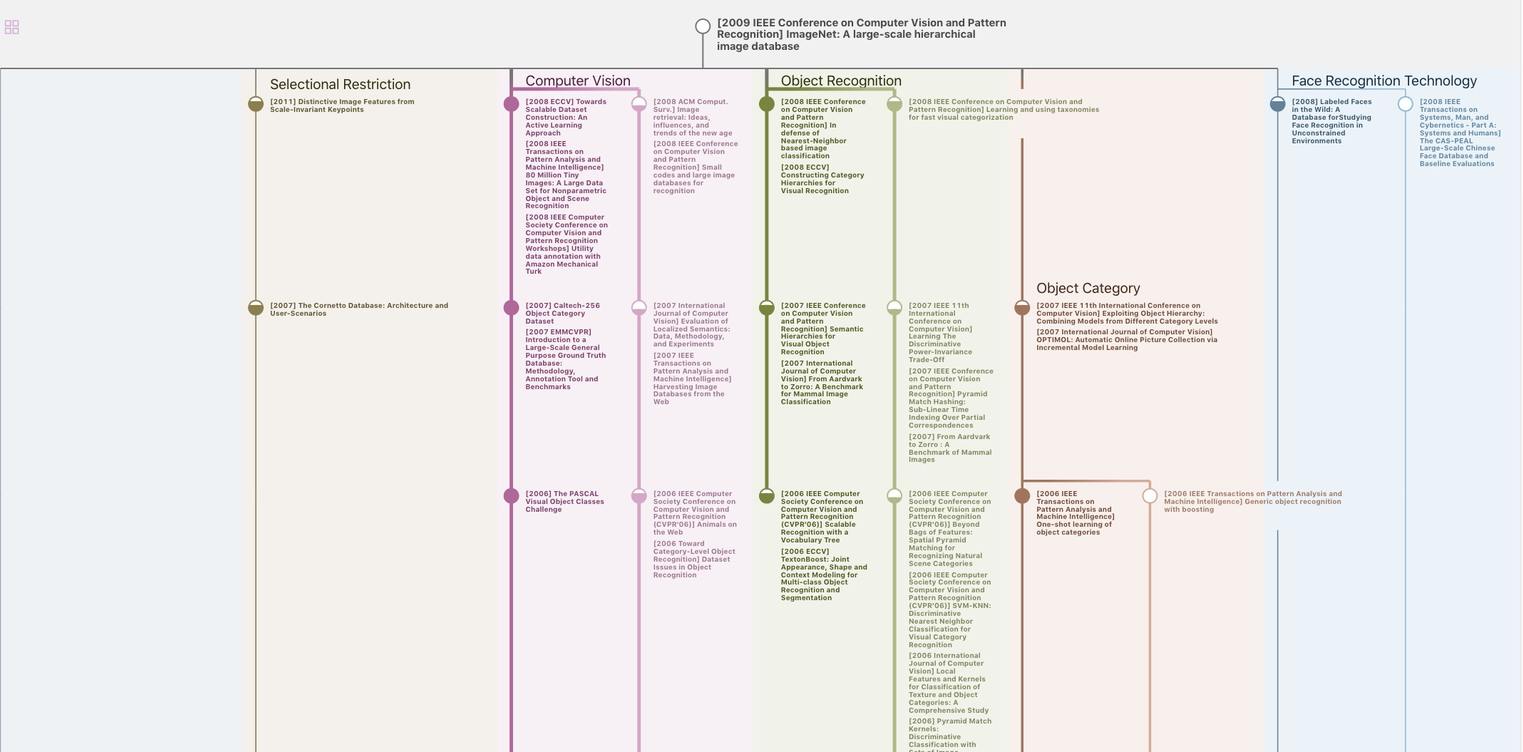
生成溯源树,研究论文发展脉络
Chat Paper
正在生成论文摘要