Fault Diagnosis Of Rolling Bearing Using The Hermitian Wavelet Analysis, Kpca And Svm
2017 INTERNATIONAL CONFERENCE ON SENSING, DIAGNOSTICS, PROGNOSTICS, AND CONTROL (SDPC)(2017)
Abstract
Fault diagnosis of rotating machinery is receiving more and more attentions since it plays an important role in guaranteeing production efficiency and safety. In this paper, a novel method of fault pattern identification for rolling element bearing (REB) based on the time-wavelet energy spectrum with the Hermitian wavelet analysis, kernel principal component analysis (KPCA) and support vector machine (SVM) is proposed. The Hermitian wavelet has a desirable ability to detect the singularity characteristic and keep instantaneous phase of fault impact signal. Therefore, continuous wavelet transform (CWT) based on the Hermitian wavelet is applied to acquire the time wavelet energy spectrum which indicates the fault statistical feature information. Then, the KPCA is used to reduce dimension of the energy spectrum because of its excellent nonlinearity. Finally, the feature vectors consisted of principle components (PCs) are fed into the SVM in order to realize fault classification. The experimental results illustrate that the proposed method can not only accurately recognize fault pattern, but also simplify the input of SVM and improve the recognition efficiency.
MoreTranslated text
Key words
Bearing, Fault diagnosis, Hermitian wavelet, Fault feature
AI Read Science
Must-Reading Tree
Example
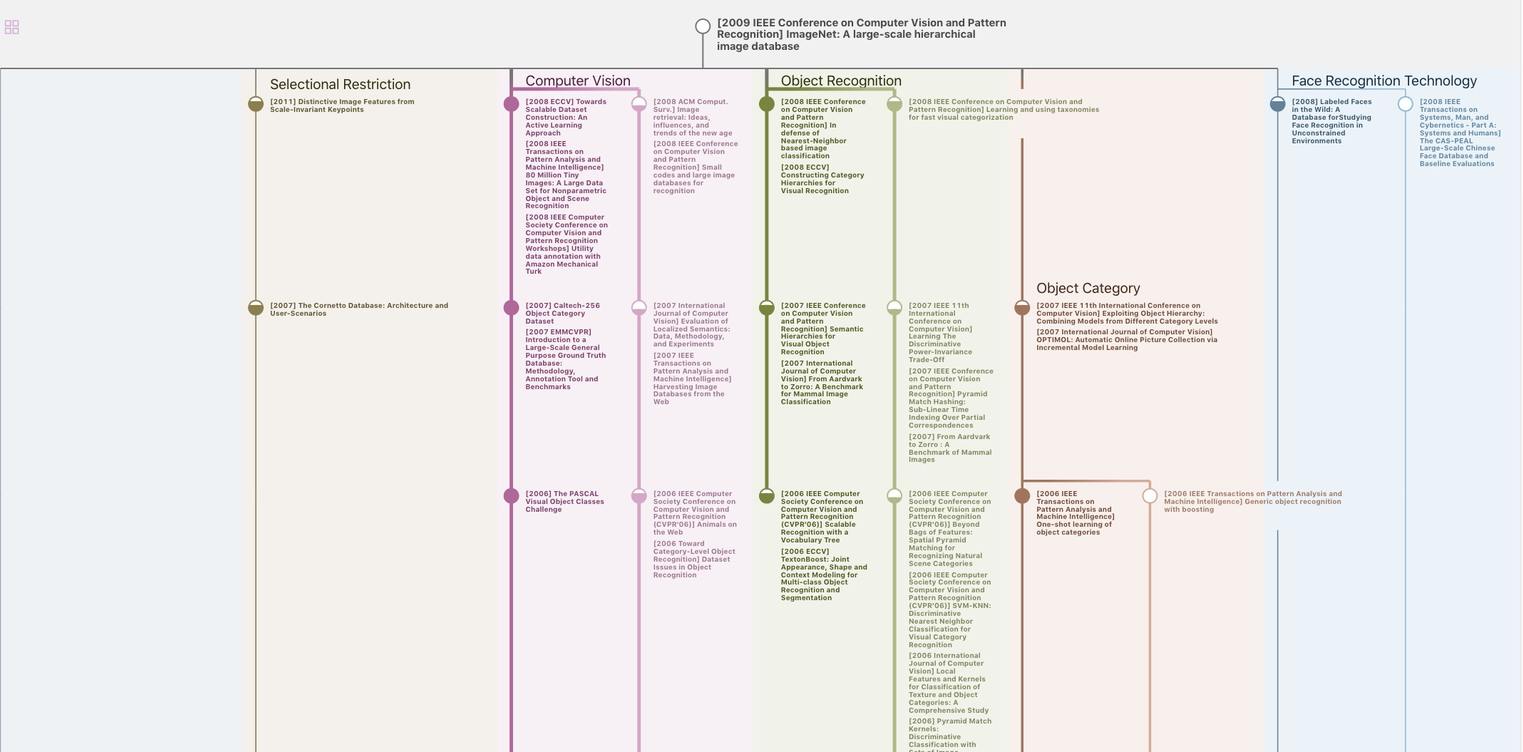
Generate MRT to find the research sequence of this paper
Chat Paper
Summary is being generated by the instructions you defined