Solving Ordinary Differential Equations Using Wavelet Neural Networks
PROCEEDINGS OF THE INTERNATIONAL CONFERENCE ON MATHEMATICAL SCIENCES AND TECHNOLOGY 2018 (MATHTECH 2018): INNOVATIVE TECHNOLOGIES FOR MATHEMATICS & MATHEMATICS FOR TECHNOLOGICAL INNOVATION(2019)
摘要
In this paper, we present an artificial neural network (ANN) approach to approximate the solutions of ordinary differential equations (ODEs) with initial conditions. The wavelet neural networks (WNNs) with Gaussian wavelet and Mexican Hat activation functions are applied as universal approximators. The proposed methods convert the application of solving ODEs from a constrained optimization problem into an unconstrained optimization problem by satisfying the initial conditions exactly. Then, the momentum backpropagation (mBP) is employed to minimize the unsupervised error function, in which the only adjustable parameters are the weights from the hidden layer to the output layer. Initial value problems (IVPs) are solved to illustrate the applicability and accuracy of the proposed momentum backpropagation wavelet neural network (mBPWNN) methods. In comparison with the solutions of other existing ANN methods, numerical results showed that the mBPWNN methods yield a superior accuracy.
更多查看译文
关键词
Backpropagation Learning,Inverse Problems,Function Approximation,Partial Differential Equations
AI 理解论文
溯源树
样例
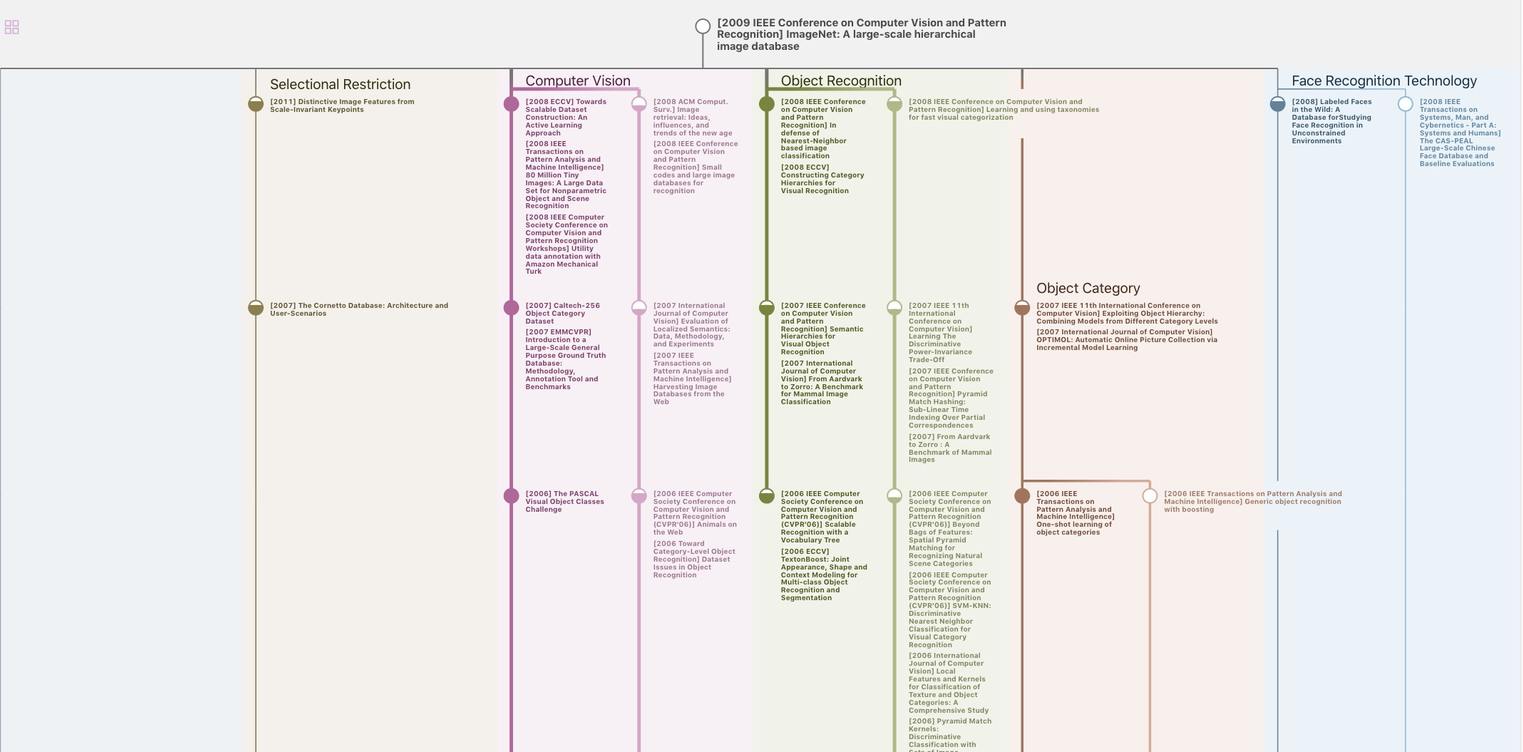
生成溯源树,研究论文发展脉络
Chat Paper
正在生成论文摘要